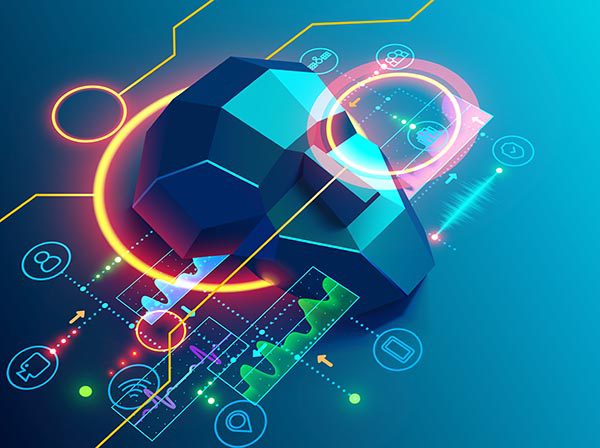
Our future will rely on advanced technologies like Data Science (DS) and machine learning (ML). These technologies not only enable people to convert machine language into comprehensible human language and improve images, but they also help us understand past incidents, shape the present outcomes, and accept the inevitability of future events.
The future potentials lie far beyond our current understanding. Data power has already transformed one industry sector after another, from healthcare to insurance, and now blockchain promises a slew of benefits for financial services, risk analysis, and even security applications. How will data scientists contribute in an environment of such superior technologies?
The Evolution of Data Scientists over the Past Decade
Over the past 10 years, data scientists contributed so strongly to product designs and manufacturing processes, that currently many product-development teams consist of a large percentage team members with Data Science backgrounds. See how Eric Mayefsky, Head of Data Science at Quora answers the question: How Will Data Science Evolve Over the next Decade?
The popular belief is that Data Science, with its varied skills composition, will continue to penetrate other business activities like manufacturing lines and product development. One problem that can confront a data scientist hired today is that very often the role that they’re hired for is not exactly what they end up doing on the job. Hopefully, as the data technologies of the future improve the talent-acquisition process, data scientists are hired with job descriptions that match their daily tasks on the job.
The Formidable Duo: Machine Learning and Data Scientists
Machine Learning technology learns from human-input data. Thus, human experts form a critical component of the machine’s “learning process.” And when machines get stuck with deep analytics, it is the human data scientists who come to rescue the machine from the comprehension bottleneck. According to The Data Scientist of the Future: What Will They be Doing?, data interpretation and visualization will always remain the domain of data scientists.
While pairing ML technology with skilled Data Scientists, the author of this Digital.gov post suggested that typically three types of learning happen when a machine is the student: supervised learning where the machine is guided by past data to arrive at future decisions; unsupervised learning where the machines makes “inferences” on its own, based on its pattern recognition capabilities; and a combination of supervised and unsupervised learning. Clearly, machines have to be guided by human input.
In the near future, the removal of data scientists is nowhere in sight. Rather, the human data experts can augment the capabilities of smart machines by pre-selecting which types of datasets can be best for learning in a given situation. This will surely save processing time.
5 Predictions for the Future of Machine Learning has pointed out that the future cloud-based business-analytics platforms will allow ML algorithms to learn continuously from net-connected resources, and that may reduce the human effort to a substantial degree during deep-dive analytics.
Another significant change in the role of data scientists that is about to happen is full-scale embracing of open-source platforms. Low-budget businesses of the future can engage Data Scientists for open-source solutions to their data-driven businesses. Contemporary Data Scientists: Working Machine Learning at Scale gives a preview of this impending trend in businesses.
Data Scientists in Future Businesses
In today’s competitive business environment, advanced technologies like ML and deep learning (DL), are rapidly becoming integral to AI applications deployed for business operations. The Realities of Machine Learning for the Benefit of Tech Leaders helps explain why embedded ML algorithms and real-time analytics are the current buzzwords in business analytics. In an era where technology seems most relevant for modern, data-driven businesses, ML investments have promised tangible value.
The basic data tasks like multi-source data collections, data cleaning, or data preparation may be handled by advanced ML algorithms, but human brains will still be required to conduct deep-dive analysis of available data. In modern analytics and BI systems, more and more data procedures and tasks are becoming automated, but machines are severely handicapped, so far as deep analytics is concerned.
Machine intelligence is solely reliant on fed data, and their learning exercise will depend on the quality, recency, and relevance of the data that has been fed. On the other hand, the human Data Scientists will be able to apply independent analysis and judgment when they look at those same data, and arrive at much better conclusions. This Medium article describes machines in action during product design.
So even the most intelligent machine with advanced algorithms will seek the help of human data scientists for “out of the ordinary” data analysis situations. Another way data scientists are needed is to identify root causes and narrow down the range of datasets required for particular analysis. Gartner explains how Data Science is enhancing the scope of ML-enriched business analytics.
Data scientists also perform exploratory data analysis, where no clear objective has been set, but valuable discoveries may be unearthed during the exploration.
Will Data Scientists Become a Core Member of Future Healthcare Teams?
Big Data, along with ML, has provisioned next-generation healthcare, where accurate and timely research of patient data can significantly improve treatment outcomes. The ability to integrate diverse types of medical data—medical histories to DNA profiles, and data from wearable devices—has increased the level of patient care.
This Forbes blog post gives a glimpse into the future of healthcare. With so much complex data from diverse sources to analyze, future healthcare teams will surely need qualified data scientists in their teams to deliver timely and efficient treatment procedures to critical patients. In such scenarios, data scientists will work in tandem with superior ML applications and tools to gather, analyze, and interpret data in real time for improved treatment decisions.
Machine Learning Automation Will NOT Replace Data Scientists
Although the pace of AI research threatened the existence of data scientists at one point in time, by now, technology leaders and experts are convinced that even full automation of ML-enabled systems have limitations, which only data scientists can resolve. Advanced machines and tools do a fine job of handling rote tasks to free up human labor and time.
It may be safe guess to conclude that future automated systems working across industry sectors will have back-up teams of highly capable data scientists to handle deep analytics and many advanced data-processing tasks that machines fail to handle.
Lastly, human brains will definitely be needed to interpret the results provided by machines, and then convert them into custom solutions for the problem at hand. Automated Machine Learning Won’t Replace Data Scientists gives reassurance of this.
What Is in Store for the Near Future of Data Science?
A Technopedia article states that 2019 is the year when AI and ML will go mainstream in data technologies. However, this year, the industry leaders and operators may see the rise of Data Engineers in place of Data Scientists, because they connect the humans with the machines. The Data Engineers need to prepare the machines and the data before the Data Scientists can dive in.
According to Sam Underwood, VP of Business Strategy in Futurety, a data analytics and marketing agency, quoted from the article above:
“Many of our clients and partners have started to kick the tires on deeper data science initiatives in 2018, and we feel that the momentum is growing quickly to integrate data science into organization-wide decision-making and policy by the end of 2019.”
According to this industry leader, 2019 will also pave the way for marketers and social-media experts to analyze more mobile and social data to fathom consumer opinions and feelings. If You Can’t Beat ’Em Join ’Em: The Future of Augmented Intelligence explains why it is smarter for humans and machines to team up.
Closing Note
8 Experts on the Future of Artificial Intelligence & Big Data has an answer to the question of how future data scientists will augment machine intelligence: AI technologies are not here to replace the human data technologists, but they can certainly offer the combined labor of millions of data scientists, over-burdened with the avalanche of data stockpiles in an average business. AI will ease the laborious data processes like data gathering, data integration, data cleaning, and data preparation, so that the human experts can spend their work time on advanced analytics.
Image used under license from Shutterstock.com