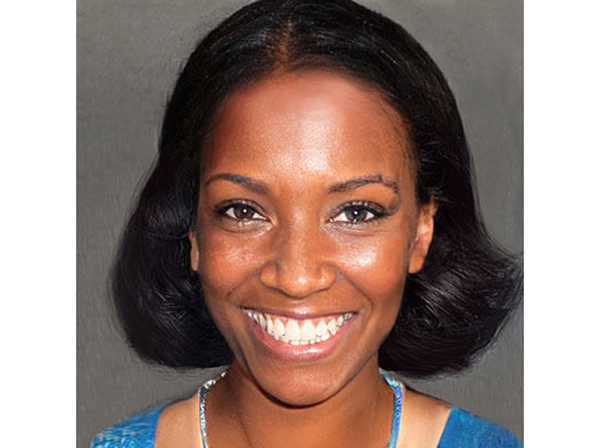
Every modern business professional understands the importance of data. Data can separate the forest from the trees, providing business leaders with a new perspective. From valuable insights regarding customer satisfaction to understanding vital improvements that you can make operationally, data analytics can provide a huge return on investment.
However, many businesses shy away from Data Science and analytics because they feel that they don’t have enough data gathered or that the data they do have isn’t organized well enough to begin an analysis – an attitude that is misguided.
This article will discuss the importance of understanding and analyzing business data, no matter how much you already have. It will also discuss the advantages of planning a data analytics approach before your company gathers large amounts of data and begins comparing Data Management strategies.
Let Your Objectives Shape Your Data Analytics – Not the Other Way Around
Many IT leaders make the mistake of shaping data analytics projects around the data they already have. Unfortunately, this approach is incorrect. It increases the likelihood that your project will “reveal” insights into issues your company has already identified or place focus on areas of improvement that should not be prioritized.
Contrary to popular belief, looking at data is not the equivalent of reading tea leaves. Gathering and organizing data by itself will not help your business run more efficiently or improve revenue. Before data is analyzed, companies should step back and identify the business problems or opportunities they seek to address through data analytics. For example, a data analytics project focused on enhancing customer satisfaction and uncovering customer needs will look much different from a project focused on improving operational efficacy.
The best way to begin a Data Science approach is to start with a clearly defined objective in mind. However, that doesn’t mean you won’t uncover unexpected, valuable insights along the way. It just enhances your probability of maximizing returns on your Data Science investments and enables your company to better focus on how they will gather data and what types of data they should focus on.
Once Objectives Are Identified, Your Data Strategy Can Begin
So, your company has decided that it would like to focus on marketing data to develop a targeted advertising campaign. Now that you have a clear objective, the next step is to consider how to gather data for analysis and how this data will be stored.
Machine learning or AI algorithms often require specific data types, while creating “data stories” or customer personas requires a more holistic approach. Understanding how your business objectives will shape your approach towards collecting data is important for a good data strategy.
All too often, companies spend weeks or months organizing and managing databases only to discover that the data they have isn’t compatible with the type of analytics tools they need to use. This is an example of putting the cart before the horse. Once your company is clear on the business problems Data Science will address, you can determine whether your organization will need to perform predictive, diagnostic, descriptive, or holistic analytics.
While it’s possible to build data warehouses in hindsight, this can often incur an unnecessary cost and delay your project. You can expect to pay at least $45 an hour for a freelance web developer to help organize and store all your data points, but keep in mind that they won’t be able to perform the data analysis for you. Therefore, it can’t be stressed enough that a company is in a much stronger position determining data objectives and the type of data needed to gain insights on said objectives before gathering large amounts of data and storing them in a particular format.
Start Small and Build Your Data Up
One common misconception in Data Science is that large volumes of data points are needed to gain valuable business insights. This is untrue, as many important business problems can be solved with simple descriptive analytics. Moreover, these business problems can even be gathered from spreadsheets at the beginning of your data project journey.
The word “data” can add a layer of complexity that is sometimes unnecessary. When companies want to discover what their customers need or uncover common pain points, they often start with a simple customer survey or request feedback and organize the data from there.
For example, client surveys have revealed that 40% of small business owners depend entirely on online banking services for their business needs. Although soliciting answers to customer surveys is simple, it can often uncover huge insights. In this case, the survey revealed how important it is for banks to focus on digital solutions to gain and retain their small business clients.
Conclusion
Far from being a disadvantage, starting your organization’s Data Science journey with limited data can enable you to create a highly focused approach toward your analytics strategy that will result in huge dividends.
Clearly defining the ideal results in your Data Science journey will help your organization better understand what data it needs to gather and whether new systems need to be put in place to generate this data. The type of data, its format, granularity, and volume will inform what type of data analytics tools you choose as well as what type of Data Management solutions are needed.