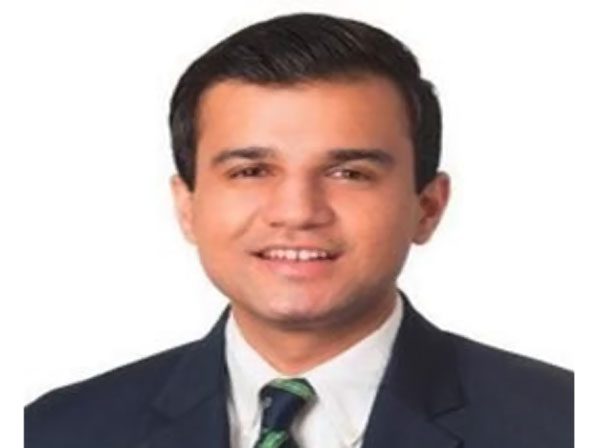
Click to learn more about author Konain Qurban.
It is a journey to ensure the alignment of analytics initiatives to organizational objectives, combined with consistent and effective coordination of activities across all business units.
The road from a pile of raw data to insights and from insights to action is paved with strategic goals. More often than not, organizations spend the majority of their time going from raw data to insights, which forms a formidable part of their analytics journey.
“Due to the existence of data irregularities, data engineers are required 80 percent of the time, and data scientists are required 20 percent of the time on a transformation roadmap. Developers are always required as part of the workflow pipeline.” – Jawad Raza, Head of Data Analytics, Big Data, and AI, Meezan Bank Limited
This is primarily because the process of extracting quality, ready-for-action insights from raw data takes a lot of time and effort. This has become the norm since, owing to much-advanced computing power and enhanced storage capacity, organizations are now faced with dealing with unprecedented amounts of unstructured or semi-structured data, more than ever before. This data is generated from various sources like financial logs, text files, multimedia forms, sensors, and instruments. Simple data visualization tools are not capable of processing such huge volumes and variety of data. And so, organizations need more complex and advanced analytical tools and algorithms in order to process, analyze, and draw meaningful insights out of it.
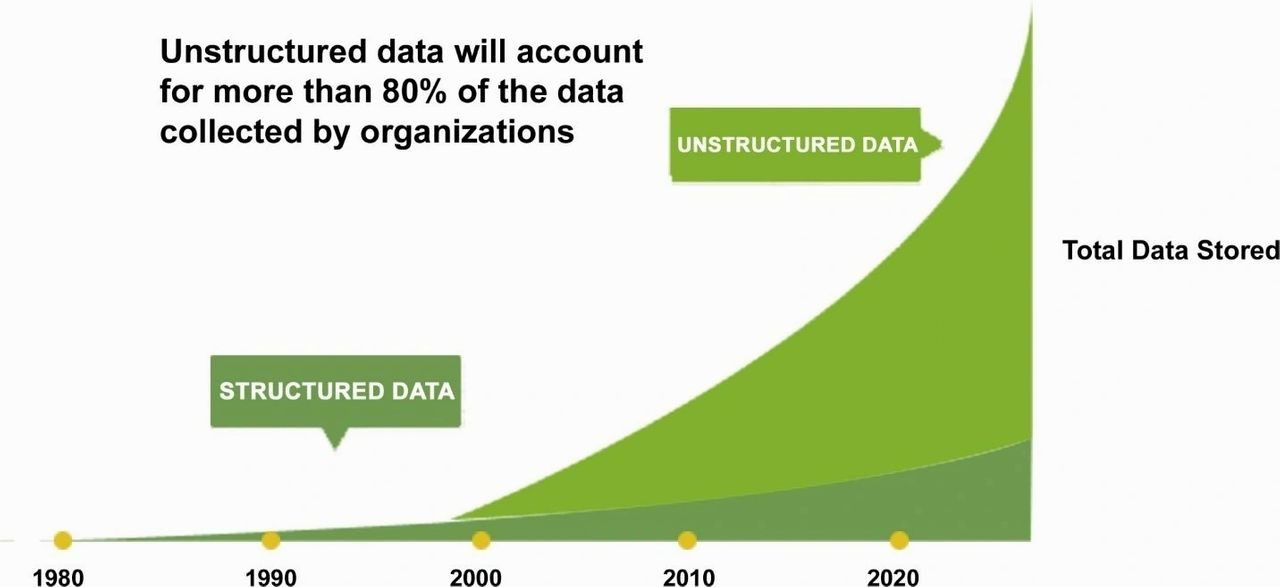
With that being said, organizations that have realized the potential of “winning with data” have already started their data-driven transformation by investing heavily in building their analytics capability and workforce. An Oliver Wyman insight suggests that the game-changing value of leading with data and analytics impacts both sides of the balance sheet for any organization — from revenue growth and deepened customer loyalty to greater efficiency and reduced risk and operational losses.
For organizations that have initiated their data-driven transformation, they have managed to alter at least some of their existing business processes. For instance, by converting their transactional and operational data into signals, these organizations have successfully been able to base at least some of their key operational, tactical, and strategic decisions on such signals. Take the popular ride-hailing company Uber, for example, that uses machine learning to connect riders with drivers by analyzing data about its customers.
With access to data growing exponentially, it has become evident that technological innovation will continue to disrupt traditionally-shaped businesses, industries, and world markets. In today’s digitally-charged global economy, the boundaries between the technology industry and other industries are quickly blurring — perhaps even disappearing.
“It’s going to continue to become more and more challenging to define what is a tech company and what is an industrial manufacturer, or a retailer, or any other type of enterprise.” – Pat Hyek, Global Technology Industry Leader, EY
So, whilst being mindful of the fact that the potential of a data-driven culture in organizations is no-doubt valuable, knowing it and implementing it are two very different things. So how does an organization transform into a data-driven organization?
The Journey Starts with the Why?
As with any business scenario, organizations must take time to understand why they need data. This simple question enables them to formalize a standard data-driven implementation plan that will ensure a successful transformation in the end. In addition to that, it also prompts a lot of follow-up questions, all of which need to be answered and thought through thoroughly. So, really, the point of this exercise is to maintain an organization’s focus on understanding the overall objectives and requirements from a business or operational standpoint.
The reality with many organizations is often alarming. They hurry to mobilize the required Business Intelligence (BI) resources without putting much thought behind the quality of their data, how it fits into their organization’s culture, the role it plays in their decision-making process, and the impact it has on other departments.
“How do you make sure that the data you get is good, clean data? ‘Garbage In, Garbage Out’ still applies. Garbage Into a great model; the model itself doesn’t give great results. So, it’s more than just the hardware, software, infrastructure, and applications; it’s the people and the governance around it that is of the essence.” – Matt Ariker, COO, Big Data Analytics, McKinsey & Company
Seldom do organizations realize the importance of the copious amounts of data they are exposed to on a daily basis, let alone have the robust Data Quality measures and controls to be able to effectively and ethically deal with it. This is the reason why organizations must focus on understanding why they need data in the first place because the answer to this question will form the basis of their Data Governance policy.
So, to sum up, for most organizations, the need for data stems from their desire to better understand their customers or to improve their customer service or product offerings. It is from this core need that organizations can then begin to implement their data-driven strategy.
Being Data-Driven
In terms of data-driven strategy, organizations must first think about treating data as a strategic asset. They also have to treat analytics as a strategic competency. Analytics is a broad term that covers topics such as Mathematics, Statistics, and Data Science, but organizations must really think about what skills they really need.
Any organization’s data-driven strategy can be broken down into three major components:
1. Organizational-Level Analytics: Organizations should really work towards having an analytics-driven culture. They also have to put the right organizational construct in place. More and more organizations, globally, are moving towards the hub and spoke organization design, where they are embedding analytics resources and teams close to the point at which the analytics is being applied. It is an intelligible and flexible concept that can be organized in a variety of ways, giving businesses immense possibilities.
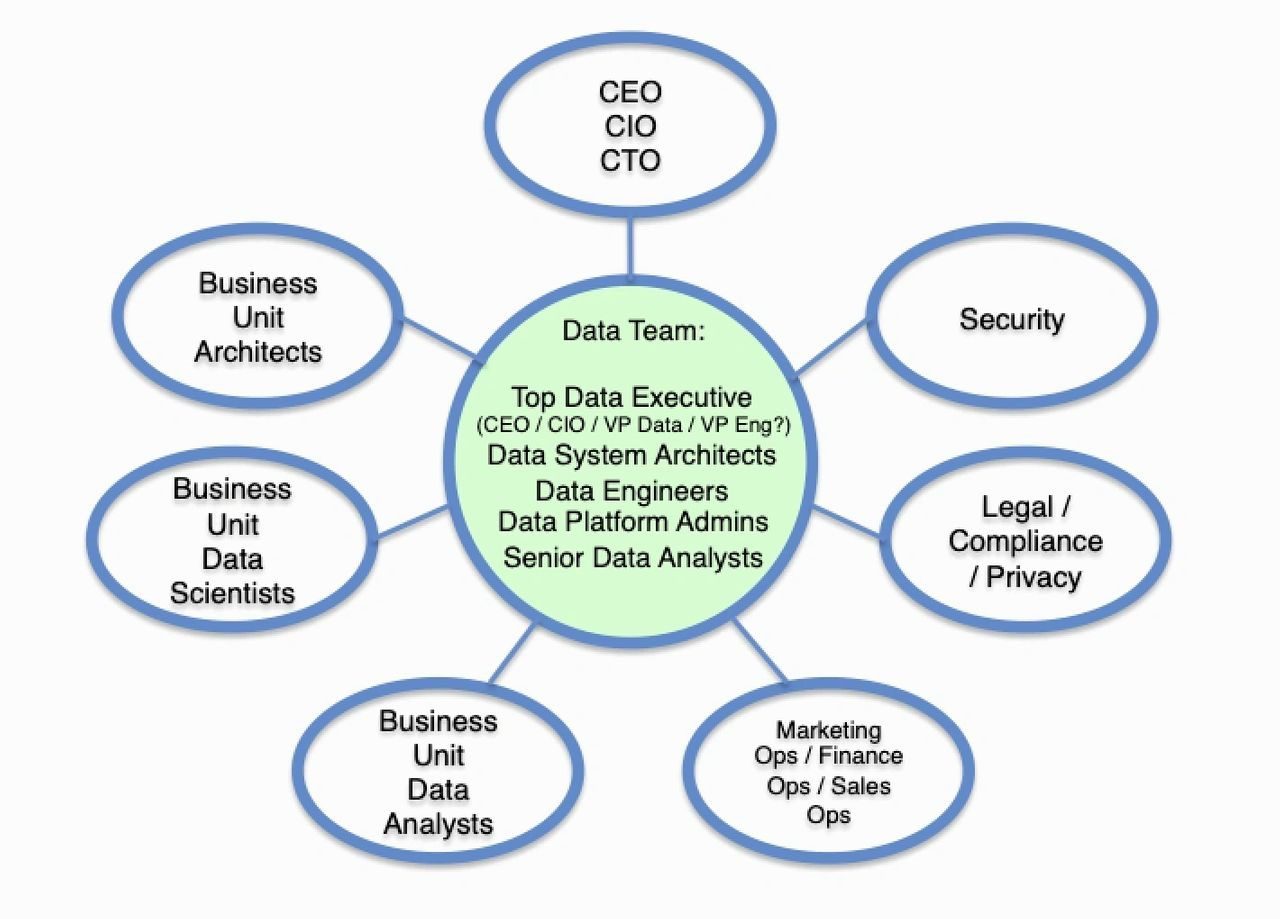
2. Individual-Level Analytics: In terms of analytics at the individual level, organizations must start with the end-user in mind. Think about the incentives that those individuals are interested in and ensure that the incentives are aligned with the changing behavior, the changing action, or the process that organizations want those end-users to take. Another key aspect is to measure results and ensure organizations have good feedback loops in place. They have to be able to see what is and is not driving value and results.
3. Human Element: Finally, it is also important to keep the customer in mind, so adding members to the data team with a background in the social science domain is really helpful. It is of the utmost value for organizations to be able to connect the data with human factors/consumer behavior. For instance, an online retail business that is trying to recommend products to its customers can realistically predict which products a customer will buy in a given period of time by looking at past purchases and browsing history.
Getting the Insights
Now, that the organizations have streamlined their data gathering and data understanding process, getting to insights is the real challenge. So, even if the data is in order, as described above, that doesn’t guarantee an automatic flow of insights. This is especially true when it comes to non-traditional sources of data, such as social media.
This is where developing the right data skills within the organization is of paramount importance. A 2016 report from CrowdFlower showed that 83 percent of organizations were struggling to meet the data skills requirements they need. These findings aligned with a study done by Gartner a few years earlier. Therefore, it is necessary for organizations to equip themselves with the right skillsets that will lead to an accurate, accessible, and integrated transformation of data into concrete insights.
Building a Data-Driven Strategy is a Patient Process but an Important One
Deploying an organization-wide data-driven transformation with the ambition to revamp, all at once, the strategy, organization, analytics, and technology stack can be an uphill task for management and employees. History and experience reveal that more than one out of two such transformation programs fail to meet the expectations and ambitions in terms of the economic return and timeline.
Hence, approaching a data-driven transformation with, for example, an end-to-end Data Architecture overhaul or an organization-wide change centered around data and analytics requires considerable patience since it can take somewhere between a few months to a few years to generate material returns and may garner strong resistance from management and employees.
Conclusion
So, to conclude, it all boils down to two things — Strategy and Leadership. Organizations have to really embed analytics into their business strategy. They need to have a business strategy that is really putting data analytics at the center of how they are competing and creating value in their industry. They also have to pick the right leader. Having an executive at the enterprise level and having leadership teams at the department and business unit levels is critically important.
As we learned over the course of this article, data analytics can provide a breadth of information to organizations on how their consumer patterns are evolving. Equipping themselves with the right skillsets can also help them create solutions to address current and future needs, particularly enhancing their digital footprint and capabilities. The current pandemic has been a powerful wake-up call for organizations of all sizes and industries, and the coming months will undoubtedly bring more surprises.
Organizations will have to figure out how to fill the gaps created by changing employee and consumer expectations and reimagine their operations for the future.