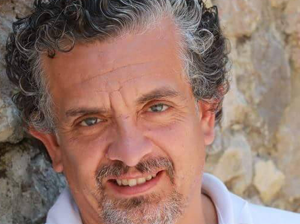
Click to learn more about author Michele Iurillo.
Sometimes large companies choose tools and technologies guided by Gartner’s Magic Quadrant in order to “not take risks,” without knowing that this is not synonymous with a guarantee of good performance and easy implementation.
In the Data Governance scenario, there are large vendors that incorporate a governance tool into their ecosystem, companies that continue to raise capital without really having customers, and other realities perhaps more specific, few known, and sometimes very effective. Being awarded “Gartner Stars” is something similar to being rewarded with Michelin stars for restaurants. Winning these stars means being under a magnifying glass and actively working to keep your position, in the process, possibly forgetting about the satisfaction of your customers — focusing more on marketing and less on development (raise your hand if you do not think so and are not a vendor).
Data is the greatest asset of our companies. You have to manage it or, as I like to say, “goal manage it,” so if you have not yet decided on a DG tool, it’s time to look for one — but do so without being guided exclusively by Gartner, Barc, etc.
As an expert, I recommend looking a little further, and I propose a series of indicators to review in order to evaluate the best Data Governance tool and make this decision for yourself.
First of all, we must clarify that if it’s true that everything starts with the metadata, a data governance tool is not a metadata tool. It is the fusion of a metadata tool with a BPM and with the presence of some kind of metadata catcher system.
At the end of this article, I will leave you with a simple Excel sheet to help you make your evaluation.
Below, I will outline which indicators should guide you in choosing the perfect tool.
1. Multi-Tenancy:The term “multi-tenancy software” refers to a software architecture in which a single instance of the software runs on a server and serves several tenants. One important issue with certain Data Governance tools is the inability to manage more than one instance and the multiplicity of roles and projects. In terms of roles, sometimes the data owner of a certain project has the role of a data steward in another project or in another domain. A DG tool must have a multi-holding approach. It’s mandatory!
2. Cloud Deployment: Does the tool allow for cloud deployment? Even if your system doesn’t need this deployment, you have to think ahead. Is it compatible with AWS, Azure, and Google Cloud? Is it compatible with your storage systems? Do you have a deployment in Docker or Kubernetes?
3. Licensed or Open Source: Until a few years ago, having to pay for a license and its maintenance was synonymous with solvency. Today, many companies prefer to bet on open source tools along with specialized professional services, since many times, these tools are just as a solvent and not as closed as commercial tools.
4. Open System or Closed Ecosystem: Many times, technology marks our choices in terms of software, generating the problem of “ghost IT.” Although it is natural to try to maintain a global technological framework, this is impossible to assume in large corporations. The more they try to pressure the system, the more the ghost IT phenomenon appears with all the security problems that it entails. We really don’t know if the current technological ecosystem will be the same in 5 years. Experts recommend that a governance tool be completely independent of the rest of the technology (for an agnostic approach), as it does not need a large volume of resources since it works with metadata and not with all the data. It is perfectly feasible, for example, that your SAP ecosystem has a different tool.
5. Usability of the Business Glossary: It is necessary to evaluate whether the Data Governance tool allows you to create taxonomies, manage business terms, and import mass business terms. Most companies have work that’s been developed in Excel or another tool such as Confluence, the reason being that all previous work can be retrieved. If this work has not yet been developed, it is best to opt for an easy and immediate tool that does not require, for example, training or certifications to be able to use it.
6. Custom Attribute: How does the software name and describe custom attributes? Beyond naming the attribute, it is important to provide a definition, a short description (with a little background), a long description (a few paragraphs of greater depth), an example, and a security classification (indicating the level of security such as public, internal or confidential). Some tools allow us to work with templates in an open way, and this is a great advantage. Check that your suite can work with templates, as this makes the work much faster.
7. Personalized Relationships: When evaluating customizable relationships, consider acronyms, abbreviations, synonyms, replacements/substitutions (indicating obsolete terms), assigned assets, allowed values (linking the business term to associated reference data), and data policies and rules. Above all, check that the tool has versioning management; it is important to be able to return or evaluate a term or a relationship. There must be full traceability of terms to know “who” has changed the “what” and “when” (this is the BPM part).
8. Data Management: Data managers must be able to manage artifacts such as business terms, data policies, data standards, Data Quality rules, Data Quality metrics, master data rules, master data tasks (e.g., duplicates), and any other artifacts that are fully configurable (e.g., regulation). Good questions are not so easily answered in the metadata tool panorama.
9. Customized Roles: Custom roles can include data owners, DGOs, data stewards, stakeholders, subject matter experts and managers, external auditors, etc. Choose a tool that does not have closed roles or, better yet, has role templates so you can create new criteria and rules at any time.
10. Authorization Workflows: It is important to define authorization workflows. For example, you can include regional managers, global managers, and IT in the case of a multi-national entry code changes. Sometimes, some DG tools present a real BPMS for the definition of workflows. Ask questions like is this term from the glossary the last one? Who modified it? What was modified? Is it a version approved by the DGO?
11. Master Data Rule: Evaluate if the tool will allow you to create data enrichment rules, create data validation rules, create relationships between entities, create record matching rules, establish confidence thresholds, and create record consolidation rules. A good friend of mine always says that if we have to make master data, we have a Data Quality problem. I also think that with data virtualization, MDM doesn’t make much sense, but it is still an argument.
12. Data Lineage: Does the tool allow you to document the data lineage, including jobs running in parallel? Does it allow a graphical visualization of the data flow? Does it have RDA and GDPR compliance? Can you recover old COBOL processes? When we think about Data Governance, we have to align ourselves with the real processes of the company. We don’t have to throw anything away, nor reconstruct anything. If something works, we have to document it, but it is not always an easy task.
13. Impact Analysis: Will the tool create an impact analysis, specifically for the assets identified in the data lineage? Is it possible to graphically visualize (with a graph database) the impact?
14. Hierarchy of Data Artifacts: The tool should allow you to link policies, rules, terms, and reference data, including automatically generating reports from the metadata and its management. From my perspective, working in Data Governance means always enriching the metadata, but do we really know what this metadata tells us?
15. Profiling of Various Data Sources: This includes manual (SQL scripts), automated (vendor tools), and various data sources (yes, some COBOL too) — not only structured but also unstructured data and especially reports and reporting (SAS, MicroStrategy, etc.). We cannot know what our technological ecosystem will be like in 5 years, so we cannot know which data sources we will use. The most coherent solution would be to have everything virtualized with tools such as C3, Querona, or Denodo.
16. Data Quality Scorecard: Don’t underestimate the value of a scorecard, which lists information governance metrics, objectives, periodic status updates, and baselines. Your tool must have the ability to use basic quality rules internally and be able to connect to external Data Quality engines. If the tool doesn’t have a good data visualization system, it can at least use external frontends to do so (such as Power BI and Tableau).
17. Problem Logging and Data Alerts: The data problem log should track the problems, the administrator assigned, the data assigned, the date resolved, and the current status (e.g., closed, administrator talking to the legal department, etc.) It is not only about generating a log but a control process of problem resolution (with a ticketing system or being able to relay to external tools such as Jira, Confluence, or Slack.)
18. Data Problem Resolution Process: Ensure that the problem management and resolution process is fully documented (we are also required to do so by regulation in many cases).
19. Support for Internal/External Audits: Each repository must have a data owner and will be audited for compliance with specific Data Governance policies, such as 1) the presence of a data dictionary, 2) whether the rules have been documented, and 3) who determines access controls. The software must be able to generate roles for external auditors.
20. Data Governance KPIs: There are many possible KPIs in DG. Here some ideas: business glossary number of candidate terms, number of pending terms, number of approved reference data, number of candidate code values, number of pending approval, number of approved data issues, number of pending data issues, number of data issues resolved in the last Data Quality period; Data Quality index by application, by critical data element information vectors, by data manager, by data owner, by data repository, by application, or by data domain.
21. DAMA: The tool should follow the DAMA-I approach expressed in the DMBoK2, a simple command that has great importance unless we have decided to follow another framework such as DGI or EDM.
The DMBoK2 text states the following:
“A Metadata Management system must be able to extract metadata from many sources. Design the architecture to be able to scan the various sources of metadata and periodically update the repository. The system must support manual metadata updates, requests, searches, and metadata lookups by various user groups.”
“A managed metadata environment should isolate the end-user from the various and disparate metadata sources. The architecture should provide a single point of access for the metadata repository. The access point must provide all related metadata resources transparently to the user. Users must be able to access the metadata without being aware of the different environments of the data sources. In large data and analytical solutions, the interface may have user-defined functions (UDF) to draw on various datasets, and exposure of the metadata to the end-user is inherent in those customizations. With less reliance on UDF in the solutions, end-users will collect, inspect, and use the datasets more directly and the supporting metadata will generally be more exposed.”
Conclusions
As I said at the beginning of this piece, data is the greatest asset of our companies. We must manage it or, as I like to say, “meta manage it,” so if you have not yet decided on a DG tool, now is time to look for one without being guided exclusively by Gartner, Barc, etc.
To make your work easier, I have included an example Excel file below with all the indicators you should look for. You will be able to modify the criteria and give more or less weight to the characteristics that are appropriate for your Data Governance project.
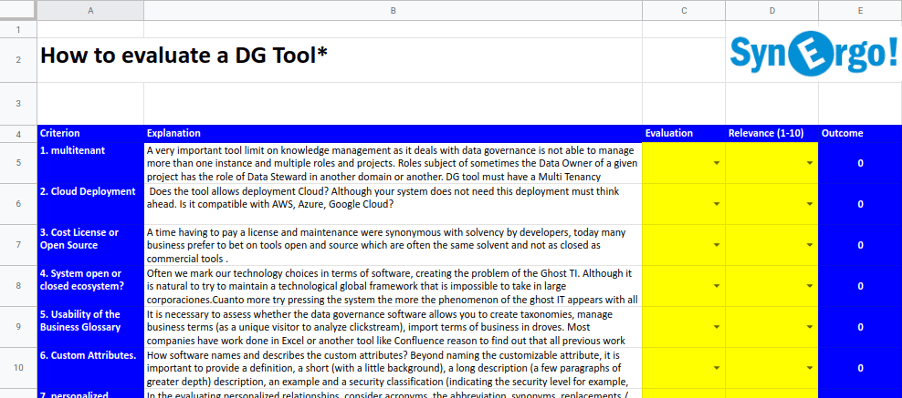