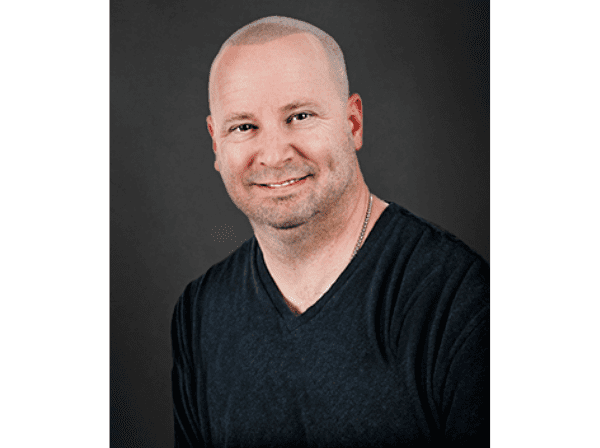
Click to learn more about author Chris Lynch.
So, you’ve made the decision to go all-in on the cloud. (BTW, I don’t blame you — as a CEO, I’ve made the same choice myself, both for the company I run and the companies that I invest in.) Now your attention needs to focus on how to maximize the ROI from your cloud investment while delivering on all the expectations. I often hear from our customers about the benefits they are experiencing from the cloud, but then they tell me that the benefits have come at the expense of performance and/or costs. This is a relatively long way of saying that the ROI isn’t living up to the hype and expectations.
Numerous Fortune 100 customers have also shared concerns about planning for cloud costs, especially in situations where they are aggressively trying to get data in the hands of the masses. In some circumstances, they’ve experienced individual query costs in excess of $50,000, which is an unforeseen budget buster. So, how do you maximize ROI for analytics in the cloud? The solution might not be as complicated as you think.
Cloud data platforms primarily charge for computation cycles, which means that for every question you ask, all your rows of data have to be scanned. This consumes CPU cycles and racks up expenses. A primary optimization tactic is to reduce the number of rows of data prior to the questions being asked, which is a traditionally manual effort and one that requires extensive business knowledge and technical expertise (like that of a data engineer). As you can imagine, this isn’t an easy person to find, and if you can find one, it’s incredibly expensive and time-consuming.
Not to mention, the data structures or tables your data engineer would create are essentially irrelevant almost immediately after they are published for consumption. This is because, like everything else in life, things change — especially the things that we are trying to analyze to ultimately influence customer or market behavior. To net it out, while there are manual methods you may attempt like engineering data and creating summarization tables and queries, there is an inherent mismatch between interactive analytic workloads. One of the most powerful capabilities that the cloud can make a reality and the manual methods of data engineering to try and make it financially feasible. So, once the unicorn hire you have found who specializes in data cleansing, pruning, and optimization completes the work — it’s all for naught.
Secondarily, latency, or the delay before a transfer of data begins following an instruction for its transfer, is significantly higher than it was on-premises. While part of this challenge is physics related (so, there’s not much we can do about that), there is a fundamentally different approach to analytics on modern cloud data platforms that isn’t perfectly aligned with the question and answer phases of BI and AI/ML workloads. This second challenge can somewhat be addressed similarly to the first challenge, but ultimately this one is more of an engagement and resource productivity challenge, whereas the previous challenge is primarily a dollars and cents challenge. While different, they are largely the same, as every executive is focused on ROI and the impact analytics in the cloud can have on their business, competitive advantage, customer sentiment, etc.
So, what should you do? It’s important to understand the economic impacts your investments will have on driving revenue through relevant, trusted KPIs, establishing predictability of compute costs in the cloud, and force-multiplying your data and analytics teams. To quantify these economic impacts, we’ve created simple calculators that you can use when defining the business outcomes you’re trying to achieve.
The good news is there are products to help achieve these economic goals that not only complement the cloud data platforms but also dramatically improve their ROI. The introduction of cloud OLAP capabilities into the data platform ecosystem (data lake, relational, on-premises, cloud, and hybrid cloud) helps to address the Data Management, semantic layer, and security/governance aspects of analytics workloads in the cloud.
With technology to automate data engineering and query optimization, our customers frequently experience an order of magnitude performance improvement with an 80 percent cost reduction. In other words, they are seeing incredible ROI in a finite window of time. Beyond the performance and platform optimizations, the power of a semantic layer enables them to migrate workloads transparently to the platform du jour, without requiring application rewrites, user re-training, etc.
As the data revolution continues and platforms emerge that promise better performance, transparency, and scalability, investing in the right complementary technology will ensure that you realize those economic benefits while securing what has previously eluded most — unprecedented ROI.