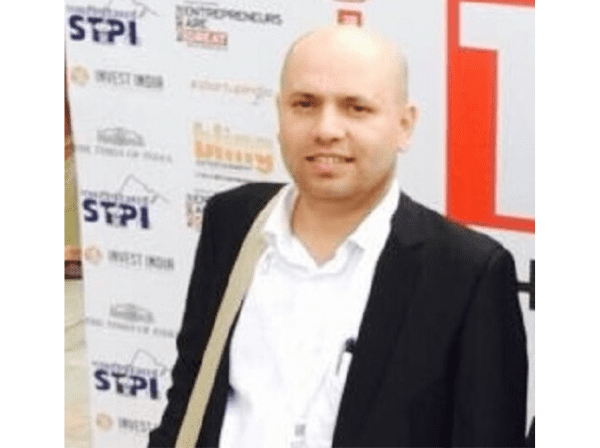
Click to learn more about author Ashok Sharma.
Machine learning (ML) algorithms are everywhere these days. AI applications aren’t something that will be present in the future. They’re already here and have begun making an impact on our lives. AI usage is currently in an embryonic stage and faces significant challenges.
The biggest challenge surrounds the availability of data. Data is the lifeblood of any AI training system, and real-world data is hard to come by. While privacy laws limit its usage, a bigger issue is that consumer behavior is changing rapidly, and historical data risks becoming obsolete by the time it’s cleaned and prepared for AI modeling.
Synthetic data is crucial to helping AI overcome this hurdle. Here’s how companies in these six industries have begun using synthetic data to power their products.
Autonomous Vehicles
The self-driving car has been a long-sought-after goal in the vehicle manufacturing industry. Even tech giants such as Google and Apple have been pursuing it, with varying degrees of success. Autonomous vehicle development is a perfect example of how real-world data limitations can stall development.
ML algorithms that power self-driving vehicles should ideally drive on public roads and learn via real-world data. However, implementing such a plan is dangerous and impractical. Synthetic data sets generated according to user specifications allow AI developers to feed as many scenarios as possible to their systems.
Vehicle algorithms are trained according to terrain and situations endlessly without putting any human lives at risk. As a result, the development of self-driving vehicles has leaped forward, and they’ve already hit public roads.
Marketing
The field of marketing has an unlimited number of use cases for ML algorithms. Everything from optimizing budget spends to creating customized campaigns to exploring buyer behavior patterns is ripe for harvesting. The issue is that GDPR and data privacy laws prevent companies from feeding consumer data to their algorithms.
Synthetic data that replicates real-world data has been heaven-sent for marketers. These data sets are created by replicating smaller, real-world data sets and adding user-defined parameters to generate data applicable to various conditions. Consumer identifying data is replaced by fake information, and this preserves user privacy.
Companies such as H&M already use bots to gather user preferences and tailor ad campaigns. Synthetic data replicated from the user data will make these marketing campaigns even more efficient.
Surveillance
“Remote sensing data (imagery captured by satellites, airplanes, and drones) provides a unique channel to uncover valuable insights on a very large scale for a wide spectrum of industries,” says Dor Herman, CEO and Co-Founder of synthetic data provider OneView.
The defense industry was an early adopter of AI. One of the first applications of AI on the battlefield was surveillance. Alerting soldiers to the potential threats and allowing them to adapt quickly to challenges is critical. Real-world data collected from war theaters is messy and unreliable due to the nature of that environment.
Synthetic data allows defense departments to model a wide variety of scenarios at the click of a few buttons. Algorithms can be trained faster and for far less cost compared to data collected from battlefields. Best of all, synthetic data allows teams to easily randomize environments and challenge their ML algorithms better during testing.
The use of AI in defense is growing to include remote unmanned vehicles and battlefield healthcare solutions. Synthetic data is the key to unlocking the true potential of AI and minimizing the loss of human lives.
Finance
The finance industry is vast, and ML applications abound throughout. Fighting money laundering is a top priority for global financial firms, and the use of AI in detecting abnormal patterns in transactions is prevalent. Detecting anti-money laundering (AML) violations are challenging because of the different variables involved.
For example, malicious actors can use any number of combinations of shell companies, numbered bank accounts, and front businesses in highly regarded jurisdictions to hide laundered money. To identify AML violations, AI algorithms need a wide variety of permutations built into the data they’re fed.
Synthetic data is the only solution for financial firms since these data sets can be generated quickly, and multiple scenarios can be built into them. The result is an algorithm that learns faster and can be put to use faster. These fraud detection algorithms also have applications in the insurance industry, helping firms unearth claims fraud.
Healthcare
Medical diagnoses contain a ton of patient identifying information that can never be used to train algorithms. However, healthcare providers must develop technology that can help detect the early onset of disease and prevent the formation of disease clusters. For example, AI chatbots can help patients self-diagnose and reduce the burden on hospital clinics.
Bots such as the ones created by Babylon Health currently help patients but don’t provide diagnoses due to liability limitations. However, synthetic data can help advance the development of these bots to the point where they can realize their full potential.
Robotics
Robotics applications have come a long way, and companies are using synthetic data these days to train their robots to react to real-world situations accurately. Synthetic data sets can be tailored to fit use cases accurately and don’t contain the randomness that real-world data possesses.
While real-world data is the ultimate test of effectiveness, it isn’t practical for training purposes. Designing data sets that allow algorithms to learn their environments’ step-by-step results is a better approach. Much like how children are taught their ABCs before expecting them to read entire books, ML algorithms need a tailored approach to learning.
Synthetic, but Accurate
Synthetic data cannot fully replicate real-world data, but this doesn’t mean it’s limited in any way. Through careful parameter definition and scenario planning, ML algorithms can use synthetic data to learn scenarios faster and more efficiently. As consumers begin expecting better experiences from AI applications, synthetic data holds the key to progress.