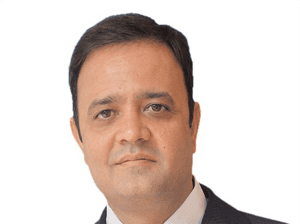
In an era where data stands as the driving force behind the sweeping wave of digital transformation and GenAI initiatives, FileOps is emerging as a true game-changer. Defined as a low-code/no-code methodology for performing and streamlining file operations, FileOps enables organizations to expedite their digital transformation and GenAI initiatives by empowering them to effectively manage data from a multitude of file formats including file servers and cloud object storage.
Traditionally, managing routine file operations required intricate code-based solutions that were custom-built and operated by data teams. However, at terabyte and petabyte scale, across millions of files, this approach proved to be too resource-intensive and slowed down an organization’s ability to fulfill its digital initiatives.
The Challenge of Data Integration at Scale
Traditional code-based file operations exacerbate data integration challenges. Developing and sustaining custom-coded scripts or programs for file operations requires a significant number of resources and can be a time-consuming process. It demands a specialized skill set, leading to high labor costs. Moreover, as data volumes grow exponentially and because data teams must be able to respond quickly to changing business requirements, these manual coding efforts become increasingly error-prone and difficult to scale.
For example, consider a scenario where a data team in a life sciences organization needs to prepare a data product to be used by a machine learning model that detects potential adverse events associated with drugs and thus improves patient safety. Here, data might reside in a data lake where it is encrypted for security reasons and compressed to save archival costs. The data might be stored in an open format such as parquet, while the ML model requires CSVs. Additionally, the data may need to be pre-processed to remove duplicates and null rows or replace some special characters. Other details may need to be addressed as well, such as detecting partitions at the source or providing a custom schema to read the files. The data team might also choose to enable parallel processing to speed up data processing.
To ensure the data product created meets the requirements of the model, business-specific Data Quality rules need to be applied to detect problems as early in the pipeline as possible to help preserve the data team’s valuable resources. It is a complicated and time-consuming process. Now, imagine doing the same thing for millions of files, across scores of use cases, within ever-demanding timelines from the business. Writing code can take the team only so far, leaving business users to wonder why it takes so long to prepare data products for their ML models.
At the enterprise scale, data teams are struggling to explain the challenges of meeting user demand and now realize that there must be a better approach to handle the repetitive tasks that take up exorbitant amounts of their bandwidth. This is where the concept of FileOps shines because it is designed specifically to anticipate the needs of the data teams to handle a wide variety of file processing requirements, at scale.
The Low-Code Revolution
Low-code/no-code methodologies are transforming the landscape of Data Management by offering an efficient alternative to complex, code-based solutions. By enabling organizations to streamline data operations and accelerate their digital initiatives, these methodologies provide business agility through quicker development and deployment of data-driven use cases in production. They also free up valuable resources for more complex work by automating routine work and improving the productivity and ROI of data investments.
In this landscape, FileOps stands out as a groundbreaking low-code solution tailored specifically for streamlining file operations. It is designed to simplify the complexities associated with tasks such as file copying, merging, encryption/decryption, file format conversion, and compression/decompression.
FileOps at Work: Criteria to Amplify Results
To optimize the effectiveness of file operations, enterprises need specific tools that comprise several key components including:
- Drag-and-drop functionality: An intuitive drag-and-drop interface simplifies the process of creating pipelines for frequently performed file operations. Data engineers can design workflows that automate these operations, reducing the need for manual coding. This capability also enhances the overall efficiency and agility in handling file-related operations and saves valuable time for data engineers.
- Scalability: FileOps functionality is designed to handle massive data volumes, making it suitable for organizations dealing with terabytes/petabytes of data. The ability to scale seamlessly ensures that file operations are done efficiently, regardless of the data volume, enabling organizations to successfully implement their data and GenAI initiatives.
- Data Management in multi-cloud environments: Organizations are increasingly turning to a multi-cloud approach to meet their unique requirements, utilizing various cloud providers tailored to specific use cases. In multi-cloud environments, integrating low-code FileOps simplifies the onboarding of data from diverse sources into different cloud platforms, enabling seamless data flow across multiple platforms.
- Embedded Data Quality: The quality of the output can be determined at each stage of a complex data transformation pipeline. By addressing Data Quality early in the development cycle, this “shift-left” approach helps to detect problems early and saves considerable time for the data team.
- Integrated monitoring: Integrated monitoring provides a single pane of glass to monitor pipeline operations and troubleshoot in case of any errors.
The Impact on Digital Transformation
FileOps will have a profound impact on an organization’s digital transformation journey. It simplifies and accelerates the execution of file operations and removes the complexities that often hinder progress. By leveraging a low-code FileOps solution, enterprises can amplify their digital transformation initiatives and gain significant momentum when routine file operations cease to be bottlenecks.
Data engineers, armed with FileOps, can enhance their productivity too by redirecting their efforts toward higher-value tasks rather than spending excessive time on manual coding. This boost in productivity can also ripple throughout the organization, resulting in a more efficient and empowered workforce.
Because FileOps offers a unified, low-code solution for managing a diverse data landscape it simplifies data integration, enhances data quality, and ensures data flows seamlessly across various sources. FileOps can also be used to help with an organization’s GenAI efforts, given that a substantial portion of the data used to train enterprise-wide GenAI models is predominantly sourced from datasets in various file formats.
FileOps functionality plays a crucial role in seamlessly integrating data from diverse file-based systems, ensuring it is readily accessible in the desired format. Lastly, it helps to significantly lower costs and improve efficiencies. By reducing the time and resources required for manual coding and file operation management, organizations can allocate their budgets more effectively, ensuring that resources are optimized.
How FileOps Is Reshaping Digital Transformation Efforts
FileOps signifies a pivotal leap forward in the realm of data and GenAI endeavors. It not only streamlines the intricacies of data operations but also expedites their execution. With its inherent scalability, adaptability, and ability to manage data across multiple cloud sources, FileOps empowers organizations to tap into the full potential of their data stored in various file formats.
In a time where data fuels innovation and provides a competitive edge, FileOps equips organizations with the tools and capabilities they need to take the lead in reshaping what’s achievable in this digital age. Equally important, it propels them toward a future defined by unparalleled success and transformation.