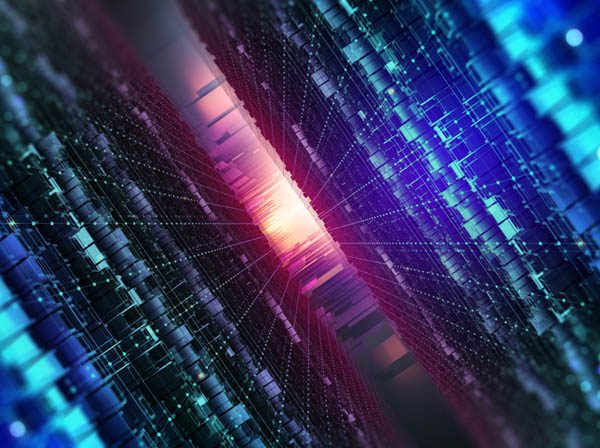
Data-driven analytics continue to deliver sophisticated solutions for manufacturing efficiency, early disease detection, and smart capabilities building in workplaces. Thus, industry operators and leaders continue raise their expectations and demands from data technologies with every passing year.
Brent Gleeson of Forbes, who regularly contributes about organizational excellence, warns that in spite of having the best infrastructure, technological support, and military intelligence, the United States could not stop many attacks against them. This important observation signals the need for speedy, data-enabled, decision-making at a time of crisis.
In The Benefits of Leading Data-Driven Organizational Change, Gleeson points out that a lack of adequate technology preparedness, lack of technology application training, and lack of informed decision-making mindset probably all contributed to the national disaster in September 2001. Transformational decision-making, according to Gleeson, requires a “shift in mindset and culture.”
Data Management Trends in 2022 argues that global businesses often make the mistake of investing too many resources in Data Architecture at the expense of data-analytics platforms. Maybe this is the time to rethink Data Management practices already in place.
Organizations Survive on Data
Data Management: Why Is It Important? shows why a strong Data Management plan must be in place. If today’s industry leaders and operators unanimously agree that modern business survives on data, it becomes easy to understand why Data Management should be at the core of all operational decision-making in businesses. At an operational level, most businesses have data mismanagement or shortcomings of one type or another, which the Stratford Managers Corporation has listed as:
- Lack of process documentation
- Inadequate data storage facilities in terms of technology
- Data silos with duplicated and outdated data
- Poor data organization tools
- Lack of Data Governance, which increases business risks
- Interoperability issues between systems
6 Reasons Why Data Management Is Important to Any Organization shares information on surpassing customer expectations in enterprise Data Management.
What Does Ideal Data Management Sound Like?
In an ideal business world, many different Data Management professionals collaborate and execute best practices to extract the maximum business value from their enterprise data assets. These professionals are data architects, data engineers, data modelers, DBAs, developers, data quality experts, and data governance experts, who work alongside executives and high-level, decision-makers to conceptualize, design, develop, and implement the desired Data Management infrastructure.
Data Management teams often work with real-time data, which requires superior data capture, data integration, data preparation, and data analytics platforms — now available due to AI and ML. Many associated technologies like data fabric, graph processing, IoT, big data, edge computing, and so on need to work in conjunction with each other to make the unified Data Management system work. At a more nitty-gritty, technical level, complex Data Management tasks happen through Metadata Management, Master Data Management, advanced data compliance tasks, and continuous monitoring.
A relatively new Data Management effort creates “data catalogs” to document which data is available where, including business glossaries, data dictionaries, and data lineage records. Data Management teams, responsible for corporate Data Management, are now accountable for data security and potential data breaches. The Data Governance teams typically include business executives with the primary responsibility of creating common data definitions and corporate standards for creating, formatting, and using data.
Core Principles of Efficient Data Management: An Expert’s View
Why You May Need to Rethink Your Investment Data Management Strategy discusses the key principles of enterprise Data Management, which are summarized here:
- Clear Assessment of ROI: Legacy systems often outgrow their needs and new systems often do not operate with old systems. Thus data silos, a lack of interoperability, and lack of unified data view across divisions or departments become nightmares. At every phase of technology upgradation or enhancement, business leaders and technical experts need to collaborate on why a system should be replaced and how. Unless the ROIs are clearly assessed and understood, technology upgrades may not yield the desired results.
- Scalability: This is another pain point because, ideally, the technology infrastructure should be able to support any planned business growth or even downsizing. While looking for data technology solutions in the marketplace, business operators should look for specific (custom) solutions that meet their specific needs rather than going for canned solutions, which may remain unused or under-used in future.
- Data Governance: Data Quality, security, and privacy are all tied up to Data Governance, thus a Data Management system with good Governance will naturally yield more consistent and reliable results. While designing a Data Management infrastructure, industry operators should keep Data Governance at the top of their priority list. A blog post states that The Data Warehousing Institute estimates that “U.S. businesses lose a cumulative 600 billion dollars a year due to problems with data quality.”
- Data Integration Platform: As businesses of all shapes and sizes continue to struggle with data silos and disconnected Data Management technologies, the forward-thinking businesses are looking at data-integration capability as the “topmost criterion” for selecting a Data Management platform. Businesses run on free and open sharing of information and data across silos, thus an efficient Data Management solution will offer flexible integration technologies and tools to reduce data silos.
- Advanced Analytics Platform: The business analytics system is the “brain” of the Data Management system, designed to deliver accurate and timely insights, competitive intelligence, and other decision-support outcomes to aid business. Thus, the analytics and BI platform must be completely trustworthy.
Shift in Core Principles of Data Management in Today’s Digital Economy discusses some core principles of enterprise Data Management including “Data Discovery, Right Data Interpretation, Coverage, Availability and Inter-operability.”
Can Advanced Data Technologies Drive Business Efficiency?
How AI Can Drive Better Data Management Across an Organization? states that business operators need to focus on the following four pillars of enterprise Data Management:
- Data Strategy
- Data Quality
- Data Access
- Data Integration
The article recommends that organizations select and invest in the right AI technologies and tools to efficiently manage the above four pillars.
An Oracle post includes some novel ways of enhancing business efficiency with the help of Data Science. The author goes beyond mere data analytics and describes how Data Science can contribute to product designs, real-time CRM, and business marketing efforts to provide real “value to an existing business model.”
Closing Thoughts
In the webinar Data Governance — Combining Data Management with Organizational Change, industry leaders Nigel Turner and Donna Burbank share an “architecture-based approach” to align business goals with organizational change management.
Image used under license from Shutterstock.com