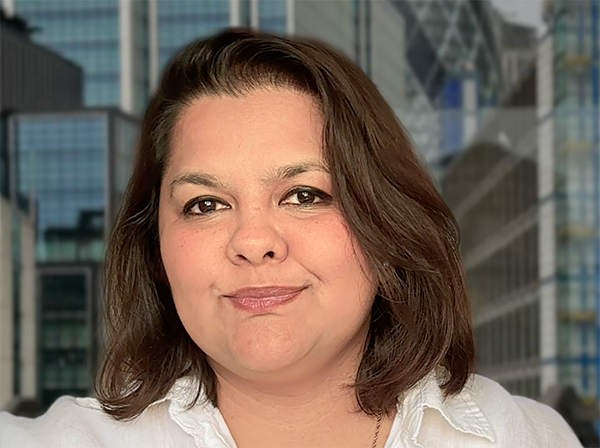
It’s a data-driven world, yet most businesses are struggling with dirty data; worse, many are still unable to perform basic tasks like deduplication and record linkage efficiently (and affordably).
A recent report from my company, “Inside 2024’s Data Quality Challenge: Insights from the Frontlines,” reveals some eye-opening insights drawn from real-world conversations with customers.
Companies are eager to train AI models and win the proverbial race of titans; however, dirty data is the Achilles heel slowing them down and making it hard to accelerate progress.
Here’s why it’s important to address dirty data in 2025.
The Escalating Crisis of Dirty Data
Messy, duplicated, and fragmented data is no longer a minor inconvenience; it has evolved into a full-blown crisis affecting organizations across industries. As AI initiatives gain momentum and the pace of CRM and ERP migrations accelerates, the dirty data dilemma poses a direct threat to business goals and the success of digital transformation projects.
Business leaders increasingly report frustration with data that hasn’t been cleaned or updated in years, duplicated name and address data in the thousands, and information that is no longer valid or correct. The consequences are far-reaching – duplicate records create confusion in customer databases, impede operational efficiency, unnecessarily inflate storage volumes, increase processing times, and introduce critical errors in reporting and analytics.
These challenges manifest in several specific ways that companies must address to maintain competitive advantage in today’s data-driven economy.
Top Data Quality Challenges Businesses Face Today
Organizations across industries confront several significant challenges when managing their data quality that extend beyond the common issues of duplicate and inconsistent records.
These challenges represent systematic problems with processes and technology implementation:
- Integration Across Multiple Data Sources: Difficulty in consolidating data from various systems creates significant barriers to achieving a unified, comprehensive view of organizational data.
- Lack of Proactive Data Cleaning and Governance: Many businesses struggle with outdated data due to years of neglect, inconsistent cleaning routines, and the absence of formal governance frameworks to maintain data integrity.
- Manual, Time-Intensive Processes: Labor-intensive manual methods – including data scrubbing, field matching, and database maintenance – remain commonplace and represent a significant source of frustration and inefficiency for data teams.
- Variations in Names and Addresses: Many businesses encounter difficulties making sense of variations in business names, personal names, and addresses, which complicates customer identification and communication.
- Data Standardization Gaps: Most organizations face ongoing challenges with inconsistent data formats that substantially reduce matching accuracy and complicate data integration efforts.
- System Integration and Workflow Challenges: Organizations increasingly require tools that integrate seamlessly with existing systems like Salesforce, Oracle, and various database platforms to ensure data quality across the entire technology stack.
The Real-World Cost of Poor Data Quality
The consequences of dirty data extend far beyond mere inconvenience. Consider these real-world scenarios:
- Marketing campaigns suffering 30% return rates on direct mail due to inaccurate contact information, resulting in wasted spending and diminished ROI
- Financial reporting discrepancies caused by duplicate customer records, creating confusion during quarterly reviews and misleading business intelligence
- Supply chain disruptions during system migrations when duplicate and inconsistent records cause delays, misdirected payments, and customer service challenges
These examples demonstrate how data quality issues can silently undermine business performance until they become impossible to ignore.
These examples also illustrate how dirty data can silently undermine business performance until it becomes too big to ignore.
Beyond Manual Processes: The Evolution of Data Management
Despite growing data volumes and complexity, approximately 65% of organizations still rely on manual methods for data cleaning and deduplication, according to our research. Teams spend countless hours in spreadsheets applying formulas, highlighting discrepancies, and cross-referencing datasets – approaches that are both time-consuming and error-prone.
As data volumes continue to expand exponentially, these manual methods become increasingly unsustainable, creating a widening gap between modern data challenges and organizational capabilities.
The Five Pillars of Effective Data Quality Management
To address these challenges effectively, organizations should consider implementing a comprehensive data quality strategy built on these core principles:
1. Establish Clear Data Governance
Develop standardized policies and procedures for data collection, storage, and maintenance across the organization. Assign responsibility for data quality to specific roles and create accountability mechanisms to ensure ongoing compliance.
2. Implement Automated Quality Controls
Move beyond manual processes by implementing automated tools for data validation, deduplication, and standardization. These solutions can dramatically reduce the time and resources required for data maintenance while improving accuracy and consistency.
3. Adopt a Data Quality Mindset
Foster a culture where data quality is recognized as everyone’s responsibility. Provide training on data hygiene practices and highlight the business impact of quality issues to encourage better data management at all levels.
4. Prioritize Integration Capabilities
Select tools and establish processes that facilitate seamless data integration across different systems and departments. This approach helps prevent data silos and supports a unified view of information throughout the organization.
5. Measure and Monitor Data Quality
Implement metrics to track data quality over time, such as duplication rates, completeness scores, and accuracy measurements. Regular monitoring allows organizations to identify emerging issues before they impact business operations.
Conclusion
The stakes around data quality have become too high for businesses to rely on manual or outdated methods. Organizations that proactively cleanse, consolidate, and standardize their data stand to maximize their investments in CRM systems, AI analytics, and compliance requirements.
For companies to make the most out of their data, data quality needs to be an ongoing process, supported by the right tools. By embracing modern data quality management solutions, businesses can transform their data from a liability into a valuable asset that drives growth and success.