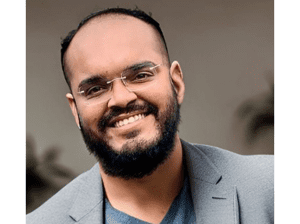
In the rapidly changing world of financial technology (fintech), fraud is a developing area seething with vigor. Digital banking and online financial services are booming every day, bringing with them new techniques for thieves to ply their trade – not ones that can be easily dismissed. Fintech firms must now relentlessly deploy data and artificial intelligence (AI) to combat these threats. This blog post discusses the latest situation concerning fraud risks in digital banking and how AI and machine learning are being applied to fraud detection. We will also discuss specific AI techniques such as anomaly detection and pattern recognition, as well as the ethical considerations involved in developing those techniques – along with a bit of prognosis for the future where AI-led fraud prevention is concerned.
Digital Banking Current Fraud Risks
Digital banking has turned the world of finance upside down, becoming a real boon for those 100 million users around the globe who are its customers. Banking this way, however, brings fresh possibilities for fraud crime. Some of the main fraud risks in digital banking are:
- Phishing and Social Engineering: Here, fraudsters use emails, messages, or phone calls that appear to be from your bank or a financial institution. They are a modern, dangerous form of attack: You’ll never know if it’s real until they clean out your account. Letting people know how to spot and avoid phishing attempts is crucial to mitigating this risk.
- Account Takeover: This means taking charge of other users’ accounts by stealing their security information or exploiting a security hole. Once the fraudsters get in, they’ll be able to run up big bills on the user’s behalf (without asking!), make transfers that should never be made, and turn the account into a center for yet more fraud. Using multi-factor authentication (MFA) and looking out for unrecognized logins at once can help to avert this problem.
- Identity Theft: Someone uses stolen personal information to set up fake accounts and/or make illicit transactions. This can cause serious financial loss to the victim and ruin their credit rating. Financial firms must conduct investigations as soon as they receive an application for credit or account creation, continuously monitor their database of users’ personal data to learn if there is any evidence that any has been stolen – and keep that monitoring system always open in the future: No announcement may ever be made that it is being closed down.
- Payment Fraud: Using payment systems to steal funds or make unauthorized transactions. Credit card fraud, wire transfer fraud, and unauthorized ACH transactions all fall within this category. Payment fraud can be detected and prevented to a great extent by features such as real-time transaction monitoring and strong authentication.
- Synthetic Identification Fraud: Combining real and fake information to create new identities for fraudulent purposes. This kind of fraud is especially difficult to detect because it produces completely new, even though seemingly authentic, identities. Advanced data analytics and machine learning can help determine whether an identity is synthetic by examining the data for inconsistencies.
How AI and Machine Learning Help Prevent Fraud
Artificial intelligence and machine learning are increasingly playing key roles in the fight against financial fraud. By analyzing large amounts of data, these technologies can find patterns that may indicate fraud or detect abnormalities that suggest follow-up investigation. Some current examples of how AI combined with machine learning help detect fraud include:
- Real-Time Transaction Monitoring: AI algorithms scrutinize transactions in real time. If any are suspicious, let alone clearly fraudulent, they are flagged for closer scrutiny. This way, banks can act promptly to stem potential fraud; this minimizes losses and keeps customers safe.
- Behavioral Analysis: Machine learning models can recognize regular as well as irregular user behavior. For example, if the spending patterns of a user suddenly change, it suggests their account may have been hijacked.
- Risk Scoring: AI can assign scores to transactions or accounts based on several different factors. This helps identify those at high risk, who are therefore more critical for investigation; there is less urgency about tackling low-risk accounts.
- Automatic Alerts: AI systems generate their own alerts as signals for potential fraud, which speeds up response times. Such warnings can be tailored to the specific requirements and risk tolerance of individual companies.
Anomaly Detection and Pattern Recognition
Two key AI techniques used in fraud detection are anomaly detection and pattern recognition.
Anomaly Detection
Anomaly detection is about identifying data points that deviate significantly from the norm. For example, in the context of fraud detection, this might amount to spotting unusual transaction amounts, unusual login locations, or odd spending patterns. Techniques used for anomaly detection include:
- Statistical Methods: Using statistical models to define what normal behavior looks like and spot cases that don’t fit it. These methods can be simple but effective in the identification of anomalies in transaction data.
- Machine Learning Models: Training models on historical data to recognize normal patterns and spot anomalies. These models can then adapt over time so that their accuracy gradually improves as they see more data.
- Clustering Algorithms: Grouping similar data points together and pointing out the ones that don’t fit into any cluster. This helps unearth hidden patterns in the data that might suggest fraud.
Pattern Recognition
Pattern recognition focuses on identifying recurrent patterns or trends in data that could indicate fraudulent activity. This includes things such as:
- Sequence Analysis: Examining the sequence of transactions in order to discover unusual patterns. For example, a run of small transactions followed by a large withdrawal might point to someone testing out their fraud before entering heavy water.
- Graph Analysis: Examining relationships between objects (e.g., accounts, transactions) to tease out connections that indicate fraud. This can help uncover networks of fraudulent activity which don’t show up in individual transactions.
- Neural Networks: Using deep learning models to recognize patterns in large datasets. These models can handle huge amounts of data and pick up on subtle patterns that a traditional approach may not notice.
Challenges and Ethical Considerations
While AI and machine learning bring tremendous benefits for fraud detection, they also raise challenges and ethical considerations.
Challenges
- Data Quality: The effectiveness of AI models depends on the quality and completeness of the data they are trained on. Inaccurate or incomplete data can lead to false positives or missed fraud.
- False Positives: High false positive rates can lead to unnecessary investigations and customer dissatisfaction. Balancing sensitivity and specificity is crucial to minimize false positives while effectively detecting fraud.
- Adaptability: Fraudsters continuously evolve their tactics, requiring AI models to be regularly updated and retrained. Staying ahead of these evolving threats is a constant challenge for financial institutions.
- Scalability: Implementing AI solutions at scale can be resource-intensive and complex. Financial institutions must invest in the necessary infrastructure and expertise to deploy and maintain these systems effectively.
Ethical Considerations
- Privacy: The use of personal data for fraud detection must comply with privacy regulations and respect user consent. Financial institutions must ensure that their data practices are transparent and that they have the necessary permissions to use customer data.
- Bias: AI models can inadvertently perpetuate biases present in the training data, leading to unfair treatment of certain groups. It is essential to regularly audit and test AI models to identify and mitigate any biases.
- Transparency: Financial institutions must ensure transparency in their use of AI, providing clear explanations of how decisions are made. This helps build trust with customers and regulators and ensures accountability.
Future Developments in AI-Powered Fraud Prevention
The future of AI-powered fraud prevention looks promising, with several exciting developments on the horizon:
- Explainable AI: Enhancing the transparency of AI models to provide clear, understandable explanations for their decisions. This can help build trust with customers and regulators and ensure that AI systems are used responsibly.
- Federated Learning: Enabling multiple institutions to collaboratively train AI models without sharing sensitive data, improving fraud detection capabilities while maintaining privacy. This approach allows institutions to benefit from shared knowledge without compromising data security.
- Advanced Behavioral Biometrics: Using sophisticated techniques to analyze user behavior (e.g., typing patterns, mouse movements) for more accurate fraud detection. These methods can provide an additional layer of security by verifying the identity of users based on their unique behavioral traits.
- Integration with Blockchain: Leveraging blockchain technology to create immutable records of transactions, enhancing the traceability and security of financial activities. Blockchain can provide a transparent and tamper-proof ledger, making it more difficult for fraudsters to manipulate transaction records.
Conclusion
As fintech grows further, so does the need for advanced fraud detection technology. Fintech companies can use big data and AI to stay ahead of increasingly sophisticated fraud attempts. There are still challenges to face and ethical considerations to be addressed, but the future of AI-driven fraud prevention looks bright. Financial institutions, by taking on board these technologies and continually adjusting their strategies, can protect their customers and remain people with whom to do business in cyberspace.