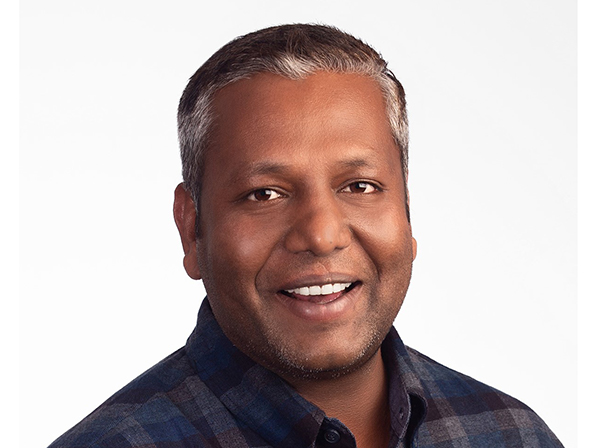
Even for the most effective marketing teams, it is impossible to achieve sustainable growth with poor customer retention rates. While companies are always eager to increase their pool of viable prospects and convert them into new opportunities for sustained growth, they need to nurture and deepen relationships with existing customers to survive in competitive markets.
The primary goal of any business leader is the one-two punch of customer expansion and retention. One key factor to watch for is customer churn, which occurs when customers cease doing business with the company. The higher a company’s churn rate, the slower it will grow. It’s just common sense. A business cannot maintain or increase its market share if it loses customers at a higher rate than it gains them. That’s why it’s important for company leaders to understand and monitor their churn rates.
Traditional data analytics tools merely inform companies of the customer loss. At best, the tools suggest insights based on typical customer pain points, such as improving onboarding processes or more frequent client outreach. Unfortunately, they cannot predict the tell-tale signs of a customer ready to abandon a business for another before they churn. That is where proactive retention through analyzing predictive behavior comes in.
Using Behavioral Analytics to Increase Customer Expansion and Retention
Analyzing behavior patterns helps business leaders better understand customers’ historical interactions with products and services and connects them with their present behaviors. That information helps company leaders make more accurate predictions about their clients’ intentions.
Algorithm support enables leaders to use customer behavior data to extrapolate predictions. When those predictions, such as similarities in behaviors, are analyzed through advanced machine learning techniques, they become more accurate. Leaders who use machine learning and algorithm support data to deterministically interpret the information are more likely to cultivate happy customers.
Six groups of predictive behavioral data can help business leaders preempt adverse customer outcomes and steer toward positive results more accurately.
- Dependencies: This information helps leaders determine proactive customer approaches such as the value of sending an email.
- Clustering: This data finds relationships between customer and cohort behaviors, such as purchasing decisions and lifestyles.
- Similarities: These details highlight relative paths and patterns, so leaders can identify and repeat what works and avoid what doesn’t.
- Matching: This technique elucidates dependencies to understand the consequences of specific actions.
- Flow: This information helps companies discover the best intervention methods to prevent adverse outcomes and cultivate more favorable ones.
- Centrality: This data gives leaders an understanding of which behaviors are most relevant for more efficient resource use, leading to better outcomes.
While two seemingly unrelated pieces of information might appear unconnected with traditional analytics tools, behavioral analytics enables leaders to make those connections promptly. The technology creates simple applications that leaders and non-specialists can use to their advantage by illuminating possible interaction benefits and risks for improved customer retention and expansion.
Course Corrections Through Pattern Recognition and Prescriptive Analytics
Pattern recognition capabilities empower business leaders to analyze client and cohort data and calculate the probabilities of customer journey trajectories. Leaders can use that information to make recommendations and course corrections to create positive interactions and outcomes as the customers’ journeys unfold.
In addition, businesses win and retain more clients by learning similarities and linkages between customer and cohort behaviors, such as their cars, housing, and banking institutions. This information reveals consumers as they are and predicts where they will be in the future so business leaders can build effective marketing, sales, and engagement strategies for them.
Predict Customer Purchasing Behaviors and Upsell Them
Machine learning (ML) can help business leaders quickly and accurately determine customers’ future purchasing decisions using their historical data. This information assists leaders in predicting a consumer’s buying journey and formulate a plan to upsell, offer discounts, or grant a free consultation before customers consult with competitors.
Collaborative filtering and matrix factorization help leaders accurately recommend products and services to consumers. They combine predictive data from behavioral analysis groups for several customers, such as clustering and centrality, to unmask similarities between their purchasing behaviors. This information is used to make recommendations for each consumer based on their purchases and reviews.
In turn, these technologies increase the business’ retention and revenue because customers prefer products and services tailored to their preferences. Amazon is a prime example of the successful use of this technology. According to Forbes, the company attributes 35% of its sales to accurate product recommendations.
Prevent Churn with Proactive Retention
Companies that make accurate product and service recommendations cultivate happier customers. The timely and precise product suggestions save customers the time and effort required to find the products and services they already need and want. When customers experience positive outcomes with a business’ recommendations, they have a better overall purchasing experience and are more likely to return.
By analyzing behavioral clusters, companies are promptly alerted when customers initiate a user journey that has historically led to churn. In turn, business leaders can discover customers’ potentially adverse actions and use that information to cultivate a positive intervention.
Leaders can examine consumers’ actions and categorize predictive outputs and inputs using sub-graphs, which update in real time. That helps them discover the best flow, such as more frequent emails or incentivization, to prevent customers from continuing down the path to churning and learn more about the issues customers are experiencing.
Retaining customers is simpler when intervention outcomes are more predictable. If a customer is dissatisfied, an automated ML solution can evaluate a company’s options and suggest optimal actions graded by probable success and cost to the company. For example, doing nothing costs $0, but it decreases churn risk by 0%. Scheduling a phone call costs $1.50 and reduces the customer’s churn risk by 18%.
Understanding Customers’ Behaviors Leads to Growth
The data collected from behavioral analytics is a real-time process. Quickly and efficiently, it helps business leaders learn, understand, and predict their customers’ actions. Then, leaders can identify complex connections so sales and marketing teams can cultivate better outcomes with those using their products and services.
Despite the increasingly competitive market, business leaders can use behavioral analytics to develop proactive retention strategies that effectively expand and retain customers. This decrease in churn rates and associated costs leads to faster company growth, better product-market fit, more efficient marketing techniques, and an overall improvement in customers’ user experience.