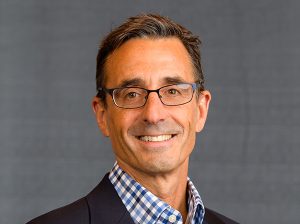
We’re seeing a lot of convergence in the market between observability vendors and companies positioned as artificial intelligence (AI) companies. It’s a natural marriage, since AI has the potential to significantly improve what observability does. The question is how to make the best use of AI to support observability in discovering an organization’s unknowns, providing actionable insights, and performing remediations.
Observability combines monitoring, visibility, and automation to determine the state of a system. It then quickly delivers actionable insights on remediating issues. That process would take countless hours if done manually. AI adds an adaptive intelligence layer that provides end-to-end automation and actionable insights in real time to improve decision-making.
A unified observability platform makes use of AI via AIOps, which applies AI and machine learning (ML) models to collect data from throughout the enterprise – from logs and alerts to applications, containers, and clouds. It performs tasks ranging from root cause analysis and incident prevention to advanced correlation. And although AI has already proved valuable, its impact is about to become considerably more pronounced, fueling observability in the near- and long-term future.
AI’s “Holy Grail”: Automated Remediation
The quest toward automated remediation is underway, but there is the potential with AIOps to do much more.
One example would be with a digital experience management platform. Currently, platforms like this have a variety of remediation scripts based on expert input that service desk agents use to either perform one-click remediations or to recommend self-service options to users. The next step is having AI begin to model decision-making like an expert would.
Via constant monitoring, an AI could ingest incoming data and detect an anomaly or some other activity that exceeds preset thresholds. It could then perform a series of actions, similar to what happens with remediation scripts, to resolve the problem.
Just as importantly, if the AI model doesn’t resolve the problem, it would automatically open a ticket with the platform used for managing issues. It will give the service desk everything they need to proceed, including the location of the issue (application, device, and network), relevant insights the model has derived, and the level of priority the issue demands. That information will enable a service desk agent to resolve the problem quickly.
Because of all those capabilities, AI automation can deliver both remediation and actionable insights to users.
How Do Organizations Prepare for the Coming AI Storm?
Organizations and IT teams that want to take advantage of AIOps should start preparing now. Here are a few important steps.
Assess the current state of the enterprise. Take a look at the monitoring and observability tools you have in place, and get a clear understanding of where your data sources are. You also need to understand your goals and objectives. What are the primary drivers for using AIOps? Is it about improving system performance? Predicting failures? Reducing downtime?
Look for a full-fidelity observability platform. AI and ML models need data to work well. And part of assessing your environment is identifying the visibility gaps in your organization. A unified observability platform can provide visibility into the entire enterprise and how everything within it is connected.
Understand the types of observability data these platforms collect. A unified platform ingests data from the full stack, including users, applications, infrastructure, network, cloud and so on. Other platforms may integrate with third parties. It’s also helpful to understand the techniques the platforms use for AI/ML. Different platforms may have different benefits.
Be more proactive than reactive with AI. Organizations may want to prioritize the transition to AIOps, perhaps starting with mission-critical applications where they could deploy an AI model, instead of runbooks, to generate intelligent tickets. IT teams also could identify recurring problems that are taking up a lot of operational cycles to see where using AI could significantly reduce the man hours spent on repetitive or recurring tasks. Applying intelligent automation or anomaly detection could identify emerging problems at a very early stage and address them proactively, rather than wasting operational cycles on being reactive.
Best Practices for AI-Fueled Observability
If you’re looking at platforms to see if they offer full-fidelity data, you should check to be sure that it’s a converged platform with strong visualization that is capable of intelligent automation or AI-driven capabilities. That’s where a platform’s actionable insights come from.
And keep in mind the importance of those insights. AIOps is about more than allowing an AI model to resolve issues. Ensuring that it generates comprehensive ServiceNow tickets is extremely critical to the process. AI is very powerful, but it isn’t infallible; the more complex the systems and processes, the more likely it is that AI can make a mistake. You don’t want to have black-box AIOps. You need to see those insights and understand as much as possible what an AI model’s decision is based on.
AI to Shape the Future of Observability
There’s going to be a greater expansion of AIOps capabilities, and more vendors will expand their use of AI in their observability offering.
A likely next step will be applying more specialized knowledge, with AI models running automations, including decision workflow automations, based on information generated for service tickets. This would give service desk agents more precise information to work with.
Other innovations are around the corner – whatever takes shape, AIOps is going to become increasingly important to organizations, and AI will be driving those new developments.