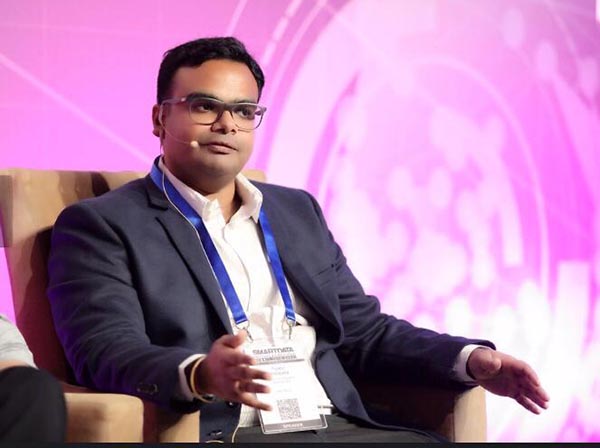
As part of a well-desired culture change of data awareness in an organization, data democratization is a concept that enables easy access to data by anyone. The ease of availability and access to data allows for direct and indirect data monetization, thus improving revenue streams. This concept can be realized through an internal marketplace as a tool in conjunction with a catalog.
While a marketplace cannot provide free access to all data, there can be risk-based controls that need to be actively managed. Some of these controls include data privacy, security, authentication, encryption, entitlements, user access management, device management, and data rights management.
The second aspect, where much time is spent churning out insight from the model, is having to store the required data as a warehouse, which can then be modeled further as a report or insight. The scope of these activities has now become a formalized science called data engineering during consumption. Integrating data engineering tools makes it possible to translate searchable elements into being available in the storage of choice, like a data lake, or a feature store.
Data Protection on the Heels of Data Democratization Enables Responsible Data Consumption
Data protection comes into play, usually in data engineering as well, when the coverage of data includes the personal data of customers. Certain questions can be asked by a data engineer, such as “Who is authorized to process this data?” “Is there consent required to process?” and “Will data need to be anonymized?”
Moreover, when looking at a catalog, information such as the privacy classification, data owner, data and privacy stewards, and associated entitlements can be absorbed into engineering analysis. We cannot overstate the need for a workflow tool, which can instantiate a pull-based service for data authorization and enrichment of information by stewards.
Additionally, if all these activities are governed manually through push-based mechanisms, a toll gate is preferred during this phase of data engineering. It could, however, be organized by a consuming data steward for that domain, who could route requests based on needs such as enhancing Data Quality, masking, or checking consents. As a domain expert, the data steward can help determine the right data coverage to be picked up for a reporting attribute or model feature.
Governing Data Through Formalized Processes and People Collaborating Through Toolsets
To complete a data journey, personnel, including data platform analysts, data engineers, data analysts, business data stewards, and privacy stewards, have to actively collaborate. These can be direct interactions with stakeholders and rely on their endorsement based on experience, expertise, and judgment on a Data Governance toolset.
Data Governance guides personnel in better managing data. The guidance is ensured through policy and ownership of data within an organization. The emphasis is on formalizing the Data Management function along with the collaboration.