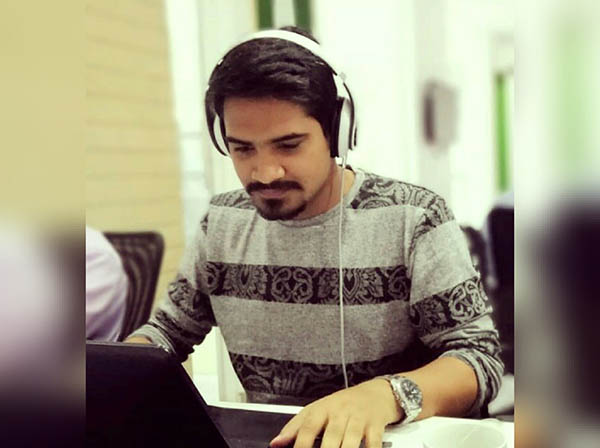
Your data strategy is only as good as the framework supporting it. If you don’t have a framework in the first place, you won’t have a strategy. This isn’t something that is necessarily complicated to plan out, but it can be confusing to know what exactly constitutes a good data foundation. With that in mind, below is a step-by-step guide you can use to build your data strategy framework and execute it successfully.
1. Determine business needs
The first step is to determine your business needs and then create an overarching data strategy that aligns with those needs. You can then work on building a framework that will support your overarching strategy. Those needs could vary from hiring the right experts to expanding server size.
Think of this step as a business plan, but for your data. How are you going to collect and use what you need?
2. Define your needs based on existing data
The second step is to define your business needs. You will need to understand the data you currently have, how it’s stored, what value it provides, and what gaps exist.
3. Start on your framework
Now that you have defined your business needs, you can start building your overarching data strategy. The first thing to do is determine who you are targeting; do this by defining your target audience or persona, which will help you understand their goals and challenges as well as provide insights around the type of content they might want/expect from you. This helps map out expectations and know where analytics fits in among those expectations (e.g., providing insight into understanding them). These personas should map out what you currently have and help define the gaps.
4. Understand data compliance
Before you start building your framework, it’s a good idea to understand what data standards exist within your industry or community – this will be especially important if your organization collects data from other organizations. Standards help ensure that your data is interoperable with other systems and allow for better integration between different tools.
5. Rely on open source
At this stage, you need to start thinking about how to build or leverage existing open-source frameworks/tools that can support those needs, as well as those of the personas you have mapped out. This includes building custom code where necessary, as many projects built using commercial off-the-shelf products are not designed for enterprise-level scale or integration.
You should also think about how data is collected, stored, and visualized – the idea is that it’s easily accessible to everyone within your organization so they can find what they are looking for quickly.
6. Define your metadata schema
You will need to define a metadata schema along with guidelines on what file names should look like so you can start collecting data in a structured way.
You’ll want to think about ingesting this data into your analytics tool. Keep in mind that there are multiple ways to collect data depending on where it lives (e.g., CRM, IoT devices) and how often it changes (e.g., files uploading into Dropbox every day vs. every hour), as well as the size of the dataset (most analytics tools have limits on the amount of data they can ingest). In addition, think about how often your team will be using that data and then choose a time interval for collection.
7. Implementation
Now that you have defined what your organization needs from a data perspective and mapped it out with a strategy in mind, the next step is to implement it – this means building or enhancing existing projects in-house or by leveraging commercial products.
This may include modifying open-source projects, integrating them with an analytics tool, or creating something entirely new. You’ll want to look at solutions across the entire technology stack when making these decisions – from where the data lives to how it’s collected, stored, visualized, and analyzed.
8. Monitoring
Finally, you’ll need to continually monitor the performance of your data strategy and framework. You can do this by looking at how happy your users are with what you’ve built – both end-users as well as developers who might be maintaining these projects. Keep in mind that one of the biggest mistakes organizations make is not investing enough time in setting up monitoring for their data solution to look at health, usage, and performance.
Conclusion
The key takeaways are knowing your audience, understanding the standards in your industry or community, thinking about how you will get/consume this data (e.g., open-source frameworks/tools), structuring it and ingesting it into your analytics platform (how often), monitoring its performance, and iterating on any of these steps if necessary to meet both organizational needs as well as the personas mapped out. Do these things well, and you will make full use of your data.