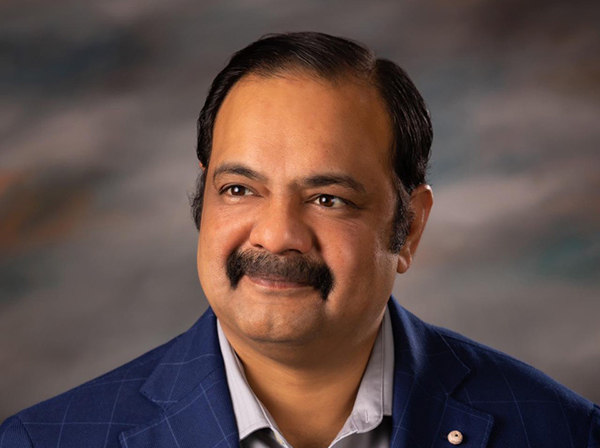
Everyone wants to increase the adoption of their AI programs, but there are several “chicken and egg” problems to overcome: How do we get the resources to build out the technical infrastructure required to use AI at scale, when we have not proven the value yet? How do we create a culture of data-driven decision-making while we’re still building algorithms? The list goes on and on.
At a high level, AI success is predicated on two concurrent processes that go hand in hand to deliver the technical framework and the organizational culture required: continuous delivery of value proof-points relating to AI, and constant maturation of the data and analytics strategy and governance framework in line with the organization’s maturing AI capability.
In the initial stages of AI implementation, it is important to plant the AI seed through proofs of concept, but a solid data and analytics strategy is also needed to communicate the desired end state. The strategy needs to include milestones and the investment in people, tools, and processes to get there.
As the organization increases the maturity of its program, it is important to invest in data and analytics literacy across the organization. Many of the structured and unstructured data points that AI and ML models rely upon are generated by frontline staff, so it’s important that there is a broad understanding and appreciation of the importance of data quality at all levels of the organization. Data and analytics literacy training are also vital for dispersing the AI vision and strategic roadmap throughout the organization and creating culture change.
Increased data and analytics literacy throughout the organization will result in a greater demand for advanced analytics and AI outputs, so it’s important to quickly follow up with some AI deliverables. The most obvious low-hanging fruit is usually about fixing internal problems or inefficiencies. These could lead to automating processes, as opposed to focusing on augmented or personalized customer experiences. Internal processes are more controllable and often follow standard procedures, so they are a good target while the organization learns how to produce and present AI outputs effectively. Additionally, letting staff interact with outputs and experience the benefits of AI first-hand is critical for AI adoption and culture change.
As institutions move up the AI maturity curve, they should also develop their AI strategy and governance. With a more data-literate workforce and some AI experience, organizations should prepare to take the next step towards building AI at scale by translating their corporate strategy and values into AI principles.
This is a very critical step in the progression toward AI at scale because it gives employees the design principles they need to innovate, implement, and iterate with AI models without needing arduous approval and governance processes. On the other hand, failing to translate and understand corporate values in the context of AI can lead to significant unintentional damage to an organization’s brand as well as customers, staff, and society at large.
With an AI strategy and principles in place, institutions will be ready to tackle more difficult AI challenges such as automated and personalized product and service recommendations. At this point, human-centered experience design principles are critical to ensuring that AI outputs are appropriately connected to the context of customers and front-line staff. Through the combination of technology, experience design, and AI, you can better deliver personalization at scale without breaking the bank.
The themes of this article are explored in Demystifying AI for the Enterprise, a book written by Prashant Natarajan, Vice President, Strategy and Products at H2O.ai, along with Bob Rogers, Edward Dixon, Jonas Christensen, Kirk Borne, Leland Wilkinson, and Shantha Mohan.