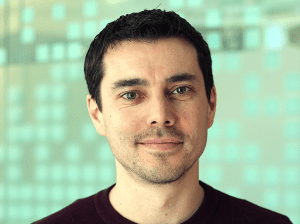
Like any new technology, a lot of people are keen to use generative AI to help them in their jobs. Accenture research found that 89% of businesses think that using generative AI to make services feel more human will open up more opportunities for them. This will force change – Accenture also found that 86% of companies thought they would have to modernize their IT and technology infrastructure.
The challenge with this is that enterprise generative AI projects will take time to design, test, build, and scale. Even with the fast path to production that new generative AI stacks offer, the risk is that people will take things into their own hands. This will lead to generative AI deployments that are off the books and outside the realm of IT, termed shadow AI. These unauthorized shadow AI deployments will take place when companies don’t engage in conversations early around generative AI and provide teams with the low-friction tools they need to succeed.
As an example, say a sales team wants help with writing their email prospect letters and wants to use generative AI in their prospecting activities. Putting data into a public large language model (LLM) might help that team be more productive, win more deals, and then deliver growth for the business. The argument will be why should they stop, and risk other companies getting ahead?
Get Ahead of Generative AI Demand
Businesses should engage with their departments on how they are thinking about generative AI and what they want to improve. This can provide opportunities to engage, listen to what business teams want, and then plan to provide a fuller strategy. It can also be an opportunity to advise teams on what is possible, go into the benefits, and debunk any hype or misapprehensions.
These conversations can provide team members with an opportunity to discover more about the business problems that their colleagues face, and then look at how to design and build generative AI services that will fit those needs. An essential part of this will be how businesses can take the data that their teams already have and combine it with generative AI to make that even more useful to them.
In the example of a sales team, how can you get information about your products ready so that a generative AI system can use your terminology and precise selling points in the responses it provides? Rather than using only the data the LLMs have been trained on, adding your data to the mix can deliver that improvement in productivity, reduce potential AI hallucinations, and deliver effective personalization. At the same time, you can keep any sensitive material under your control, rather than handing it over to a third party.
Differentiation with Data and Generative AI
Generative AI should help you differentiate what your company does. However, using public LLMs alone will not deliver this, and you will sound the same as everyone else. Companies can make their generative AI strategies more effective and tailored for them and for employees by bringing their own data to the table using retrieval augmented generation, or RAG.
RAG takes your own data, gets it ready for use with generative AI, and then passes this data as context into the LLM when your employee asks for a response. RAG is part of solving problems like hallucinations, and it also makes results more relevant for your organization and your customers, rather than getting similar results to other companies that are asking for the same kinds of questions. This is something that you have to do for your organization and customers, as no other company will have the same depth or combination of data that you can provide.
To implement this, you will have to combine various tools from vector data stores and AI integrations to build a RAG stack that makes it easier and faster to get started. Delivering this quickly will help you prevent some of those “off the books” deployments that teams might try to do for themselves while they wait for central IT. Techniques like RAG also reduce the risks of data leaks by allowing you to leverage company data for improved context without training it into the LLM.
Over time, you may want to make generative AI services available to more users within your organization by embracing low-code and no-code approaches to building services. Adopting a “center of excellence” approach, where you can offer guidance and support rather than running full implementations, increases the chances to make these technologies accessible to everyone without being slowed down by central IT, while still having the right guardrails in place for how these services get used in practice.
Building a Mature Approach to Generative AI Over Time
Looking more broadly, companies will have to come up with their own generative AI maturity models, where they look at the technology elements alongside issues like data privacy and compliance, social impact, and team culture. These elements don’t happen in a vacuum, so thinking about them early gives you a better chance to ensure that you take the right approach over time, making it easier to comply with any relevant rules and regulations that are developed.
Alongside this, you should temper expectations and level set around what generative AI is and can really deliver. For instance, generative AI will not let you replace swathes of staff with AI. Instead, generative AI can deliver better and more productive staff that can use tools in their working lives to compete against other companies that either don’t have generative AI, or that have vanilla LLM tools at their disposal. AI-powered staff can get more work done, to higher levels of quality, and start to address items on your backlog that you previously did not have the bandwidth to address. With so much potential for these tools, we must get ahead of the potential pitfalls, including shadow AI.
As Peter Parker in “Spiderman” is always told, great power comes with great responsibility. In the case of generative AI, harnessing this power will be table stakes for all organizations. Taking responsibility for quickly putting generative AI in the hands of those who can really take advantage of that power will be where organizations can differentiate themselves and avoid the pitfalls of shadow AI.