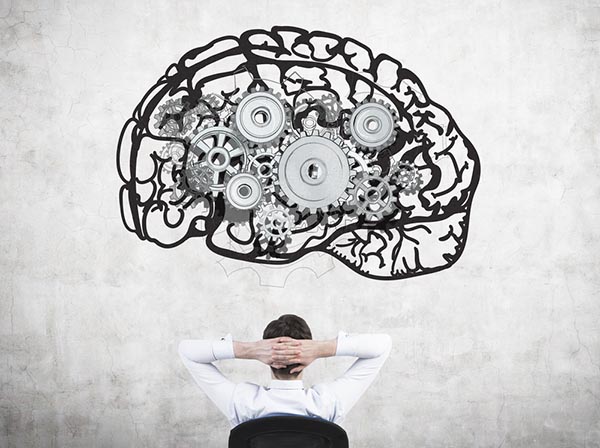
The recent organizational push for self-service Business Intelligence has helped the next challenge for business users become an increasing need. How to tackle the issue of having Machine Learning (ML) models embedded in all major analytics platforms? On the one hand, embedded models offer greater freedom and control over data analysis; on the other hand, confronting the native ML intelligence of these platforms is posing new risks and opportunities for ordinary users.
Without having the requisite background in Data Science or Artificial Intelligence (AI), how will these users or aspiring citizen data scientists prepare themselves for self-service Machine Learning? The Gartner article 5 Ways Data Science and Machine Learning Impact Business discusses how Data Science and Machine Learning together are becoming the core differentiators between businesses that survive and those that don’t.
Arrival of Big Data Accelerated Data Science Across Industries
The wide proliferation of Big Data in the global business climate spurred AI and ML research for years now, which led to revolutionary discoveries in Data Science. Lately, the easy availability of vast amounts of business data and affordable storage technologies triggered smart analytics and data-enabled BI across businesses. Lastly, cloud-facilitated “managed analytics and BI platforms,” spare medium or small-sized businesses from costly investments in in-house data centers or Data Science teams. More and more businesses of all shapes and sizes started choosing cloud-based, IT and data analytics solutions to fulfill their Data Management needs.
In 2017 Machine Learning Trends, it was discussed how Forrester predicted ML will increasingly feed on IoT data as sensors and smart apps take more prominence in our daily lives.
According to a 2018 release from Gartner, Jim Hare, Research Vice President at Gartner sent a note following this release, which indicated that businesses were probably in a rush for AI adoption even before they had figured out their enterprise Data Strategy. In spite of the recent Data Science and AI buzz, this was a massive stumbling block for the businesses to overcome.
The Gartner Magic Quadrant for Data Science and Machine Learning 2018 showed that industry players are going through turbulent times, with significant players missing out and small player like TIBCO making the ranks. This market turbulence points to one thing: that vendors who listened to the customers went up in rank overnight. There has been a huge push for self-service, as more business users have shied away from depending on data scientists for their day-to-day work.
The sudden outcry for self-service business analytics was heard and tackled by several vendors, and these are the ones who made it back into the market while others disappeared. Now that Google and Amazon are vying for top positions in the AI-enabled business analytics space, it is just a matter of time before self-service Machine Learning becomes mainstream in businesses.
The growth of Enterprise Data Management has happened in tandem with the growth of AI and allied sciences. As the science of Data Management improved through Data Quality, Data Governance, data security, and data privacy initiatives, the research and development of AI and Ml grew by leaps and bounds to make Data Strategy and smart analytics ready for each other.
Self-service Business Analytics is Replacing Data Scientists
Gartner predicted that by 2019, self-service analytics platforms would deliver more Data Science than data scientists. The Gartner prediction was widely publicized and debated across the Data Science industry, as evident in the Datanami article Self-Service Analytics Seen Overtaking Data Scientists. In 2018, Gartner also predicted that self-service analytics and BI will outpace traditional Data Science by the end of 2019.
While it’s not yet clear if this prediction will prove true, certainly many analytics activities are now in the realm of self-service Machine Learning. Many business analytics activities like data cleaning, data preparation, and data modeling have been automated, thanks to advanced ML algorithms and models. This new trend not only mitigates the Data Science skill gap, but also indicates the rapid growth of automated or semi-automated business analytics platforms.
So, what is the goal of self-service Analytics or BI? In the modern business world wrought with everyday work and time pressures, users struggle to comprehend, organize, and analyze data even for routine decision-making. self-service analytics solutions offer automated solutions to many Data Management tasks like data collecting, labeling, cleaning, and preparing, so that non-techie business users can quickly make a decision without spending hours preparing the data.
This new generation analytics or BI platform is gradually being picked up among the global business communities, and now refined ML algorithms and models are automating most analytics tasks, too.
The Major Drivers of Self-service Analytics and BI
After years of dormancy in AI research, why have the last five years witnessed a meteoric rise of self-service, business analytics platforms? The reasons include:
- Advances in AI and ML research due to Big Data.
- Arrival of Internet of Things (IoT), ramping up data volume, velocity, and variety.
- Access to cloud data repositories.
- Access to managed analytics and BI platforms (PaaS, SaaS, BaaS, etc.)
Machine Learning has Drastically Reduced the Learning Curve for Business Users
Automated or semi-automated data preparation, data modeling, and data analytics tools have taken hours of hard labor, difficult comprehension, or complicated processes out of business analytics. Now, an average business user can aspire to be a citizen data scientist, pursuing data-enabled analytics or BI activities without help from professional Data Science teams.
The Salient Features of Self-service Macnine Learning Platforms
Here are some commonalities across Self-service ML platforms:
- Removing complicated security and Data Governance concerns from data preparation, ML has aptly utilized “data socialization” techniques to deliver a friendly solution where DS community members and ordinary business users can collaborate for increased efficiency and business decision making, as suggested in KDNugget’s article.
- ML Platforms were in need of automated, data-integration features. In the real world, most Data Scientists spend most of their analytics time on “gathering, labeling, cleaning, and organizing” data, which could be accomplished with automated ML solutions. The Iris Integration Assistant from SnapLogic is an example of self-service, data integration tool.
- In self-service ML, the training and deploying of an ML model is automated, leaving the business user to concentrate on the data.
- Many of the analytics tasks on an ML platform are either semi-automated or fully automated.
- The reporting capabilities are automated with specific tuning features for the advanced users.
- The last benefit of self-service ML platforms is that it not only promises data democratization, but also removes the need for heavily invested data centers or Data Science teams.
Self-service Machine Learning Applications
There are many different capabilities that now exist for self-service Machine Learning, including some of those below:
- Data integrated solutions.
- Customer self-service solution with an AI platform. This trend points toward replacing traditional FAQs with AI-powered bots for automated customer service.
- Big Data enabled smart analytics through a point-and-click interface.
- Unstructured data analysis in support libraries with the help of NLP.
- Data Science end-to-end service platforms..
Is there any risk involved in self-service Machine Learning? Clearly, that is an important question to be answered as the technology moves forward and into the hands of daily users. One of the biggest issues though is that there may be too much data for the average user to know where to start. How to address that problem is critical in moving the rest of the process forward into the future.
Image used under license from Shutterstock.com