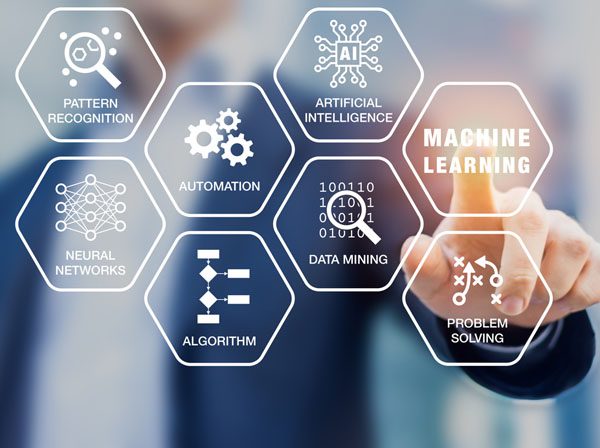
The famous theoretical physicist Stephen Hawking said, “It’s tempting to dismiss the notion of highly intelligent machines as mere science fiction.”
Artificial intelligence (AI), the game-changer technology of the global business world, comprises three distinct sub-disciplines: machine learning (ML), natural language processing (NLP), and cognitive computing. Automated solutions in business analytics use all these sub-technologies, but in varying degrees. Most advanced analytics platforms have incorporated ML or deep learning (DL) techniques to remain competitive in the market.
According to Gartner, 40 percent of all new enterprise applications will include AI technologies by 2021. On the other hand, organizations are flooded with data; the current challenge is extracting competitive intelligence from that “deluge of data.” Businesses that plan on surviving the digital tsunami (big data and IoT), have all put a definite business strategy in place, which connects data, analytics, and AI across the operative landscape.
As AI technology advances, “autonomous analytics” and self-service BI will gradually become the norm rather than the exception. Democratization of Data Science has been a growing demand of business users, and now ML-driven analytics will be one more step toward fulfilling it. Fundamentals of Self-Service Machine Learning points out the possible tussle between embedded ML models and native ML intelligence that users will have to grapple with in all self-service BL platforms.
Insights on Demand: The Background
It all started in 2017, when the market research industry began to think about “democratization” of market-research practices. This way of thinking set a revolutionary trend for the market research experts: the democratic vision of “insights” led to the blending of technology with business processes to extract customer intelligence in real time.
Today, this is not just a market-research trend. It sets a trend for all industry sectors trying to understand the customer. Because the customer is king, using technology with available customer data to better understand customer preferences, tastes, and attitudes is a great idea. Insights on Demand: Building Success in the On-Demand Economy, authored by ITWP CEO and founder, Frédéric-Charles Petit, has lessons for all marketers and business users. This book helps any reader, regardless of business role, understand the heightened need for customer insights in an on-demand (real time) economy.
The essence of ML-driven analytics is “insights on demand,” where ordinary business users must learn to combine available technology with shifting market sentiments to better understand customer needs. The idea is to use automated analytics solutions to access and apply a readymade “insight” for instantaneous decision-making.
The role of big data is rapidly expanding in business analytics, and this is one area where ML-enabled analytics will be most effective. Today’s business users and customers want to feel empowered; ML-enabled analytics promises empowerment, among other things.
Insights on Demand: New Frontiers for Emerging Businesses
The DIKW pyramid (data pyramid), features “data” as the lowest level of the pyramid in terms of value, “information” as the next higher level, “knowledge” above that, and “wisdom” as the highest point of the pyramid. The hidden implication of the DIKW pyramid is: for “data” to transform into “wisdom,” a very smart human or a very smart machine has to be present. In recent times, solutions like IBM Cloud Pak for Data offers that combination of data and smart machine,which has been described as an automated platform for collecting, organizing, and analyzing data. Businesses that lack the financial strength to invest in costly data centers, high-end hardware and application, and expensive personnel, can now turn to cloud solution providers for all their data center needs without any in-house investment, administrative headaches, or operational hurdles. Interestingly enough, the outsourced analytics service providers are generally equipped with enterprise-grade analytics and BI systems, which will provide the subscribed business users with instant access to “insights on demand.”
ML-Enabled Analytics: New Opportunities
The recent advances in automation technologies such as robotics or machine learning has yielded real value in particular industry sectors. For example, automated haul trucks in Australian mines have resulted in a 10 to 20 percent increase in utilization; Google has used an ML solution to cut energy usage by 40 percent; financial services can increase throughput and reduce errors with automated processes.
But how have the global businesses responded to the flurry of automated analytics platforms, widely available in the markets today? Actually, quite favorably. The benefits of ML-enabled analytics are all around you — the Amazon recommender engine, a bank notification about an aborted (suspicious) transaction, or Uber delivering a car to your doorstep are all results of ML-enabled, big data analytics.
Yellowfin, a pioneer in AI and ML-enabled analytics and BI solutions, assures customers that ML-enabled analytics will result in substantial cost savings, time savings, and enhanced rate of innovation across businesses.
ML-Enabled Analytics: Challenges and Hurdles
McKinsey’s Report titled What’s Now and Next in Analytics, AI, and Automation lists the following challenges of ML-enabled analytics:
- Transitioning from legacy systems in large operations
- Huge disparity of adoption within various types of digitization
- Newer data types
- Uneven digitization across sectors — media, financial services, and ICT sectors seem to be leading the automation wave, while labor-intensive sectors like retail are in early stages
- The U.S., France, E.U., Germany and Italy are moving ahead while the emerging economies are far behind
- Cross-border data flow has grown by leaps and bounds, leading to security and privacy concerns
- Segregating useful data sets for relevant insights from huge piles of data
- An autonomous architecture to capture cross-function data within enterprises
- Lack of in-house expertise to handle full range of Data Science activities
- Modifying existing business processes to incorporate insights in the workflow
- Training manpower to properly interpret data-driven insights
- Too much reliance on demographic data where behavioral data is available
- Human biases or errors in a data-rich environment
- A recent McKinsey survey of over 500 executives across sectors has revealed that “more than 85 percent acknowledged that they were only somewhat effective at meeting goals they set for their data and analytics initiatives.”
Use Cases for ML-Enabled Analytics: the BFSI Sector
The Analytics India Mag article titled How Data Analytics Backed by AI and ML Is Transforming the BFSI Sector aptly describes the automation revolution transforming the banking, financial services, and insurance (BFSI) sector.
Due to the widespread digital disruption and stringent regulatory requirements, banking, fintech, and insurance businesses have been forced to modernize their business operations. The result of that modernization, which primarily indicates technology up gradation, is evident in the digitally remodeled banks, financial services, and insurance sectors.
The BFSI sector is perfectly suited for digital disruption due to huge volumes of customer and transactional data and the growing regulatory requirements. The BFSI-sector customers today are not only better informed about the choices they make, but they also feel more empowered in their buying journey. Advanced technologies like Ml-enabled analytics have completely transformed their customer experiences.
The direct benefits of using ML-enabled analytics in the BFSI sector may be summed up as:
- Enhanced customer experience through chat bots, recommenders, and digital assistants
- Tailored advisory service through informed digital assistants
- Fraud detection and timely alerts about suspicious transactions
- Automated regulatory compliance
- Stock predictions
Future Hurdles of Automation
As the frenetic pace of automation technologies confront the modern business world, future adoption will depend on the following factors:
- Technical feasibility study of promised solutions
- Cost of adoption (development and deployment)
- Comparative study of automation vs. labor market
- Assessment of business benefits that go beyond just labor replacement
- Regulatory hurdles
- Social acceptance
A Formidable Test for Insights on Demand: Predictive Analytics
Think of pattern recognition in big data — no, humans can never do that on their own. Take the case of Wall Street investors or stock-market: they thrive on advanced AI tools for predicting market movements. AI tools can also analyze public sentiments (sentiment analysis), which, when compared to historical data, can help predict stock performance. The biggest benefit of using automated tools for predictive analytics is that the “human bias” factor is completely removed.
This Callminer post discusses how ML and DL can be used together for demand, fraud, and failure predictions. In the retail business, retailers often use predictive models to modify product pricing. Car dealers use ML-powered predictive models to predict when auto components will break down to offer timely post-sales service.
The role of Future Business Analysts is described in The Business Analyst in the World of Artificial Intelligence and Machine Learning. In the next 10 years, the human Business Analyst will end up partnering with automated systems to “analyze, measure, and refine business processes.”
Image used under license from Shutterstock.com