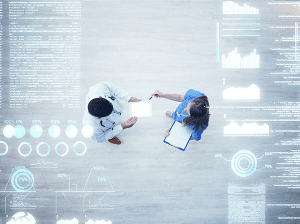
Data collaboration allows organizations to gain insights beyond what their data provides. By sharing information smartly and selectively with partners, companies can uncover new opportunities and insights beyond their internal repository. Moreover, the emergence of large language models (LLMs) applications – like Chat GPT – and cloud technologies, make this approach more attractive.
As businesses become more digitized, they can streamline transactions across fewer organizations, leading to more efficiencies in that industry. Additionally, sharing and exchanging data with subsidiaries, partners, or third parties promises new insights and services with less overhead. Companies need to understand its fundamentals and what it means to leverage data collaboration effectively.
What Is Data Collaboration?
Data collaboration takes data alignment (the agreement among stakeholders) to the next level by recognizing that companies need to supplement their existing data resources. They need to enrich their data by using other companies’ data to fill gaps and gain new capabilities from better insights.
Businesses synchronize and create a joint data ecosystem with a secured and governed data environment. This environment provides access to a diverse set of resources including people, processes, systems, and AI models to industry-specific needs.
Consider Williams-Sonoma and Whole Foods collaborating on data. If Williams-Sonoma notices a spike in pizza oven sales in certain areas, sharing this insight allows Whole Foods to proactively increase the inventory of pizza ingredients like dough, sauce, and vegetables to meet local demand.
Conversely, if Whole Foods sees a surge in whole coffee bean purchases, alerting Williams-Sonoma enables them to effectively market espresso-making classes to those customers. This synergy, where business activities build off one another’s data insights, exemplifies the power of data collaboration.
Core Requirements for Effective Data Collaboration
Partner organizations need to have core enablers to reap the rewards of data collaboration. These components, a Data Strategy and Data Governance, establish agreement on data production consumption, and usage of internal-only and shared assets.
A Data Strategy directs planning and data activities. It supports the big picture of business goals by leveraging data and outlining steps to achieve them through a Data Strategy roadmap.
Developing a solid Data Strategy and its roadmap helps identify and prioritize use cases. Organizations should start with an internal template to understand current capabilities collaboration before exploring partnership with a third-party.
Also, organizations should work through major requirements and provide evidence of value from their Data Strategy before looking to a joint data ecosystem. For example, Whole Foods may identify produce quantity per quarter as a key use case. First, it may need to update its data processes and activities to tackle this objective before addressing a case requiring more collaboration.
Once partners start implementing joint data-driven projects, new opportunities and challenges will likely emerge. To adapt to this reality, each company will need to update its individual Data Strategy. Each partner company must also revisit and re-align their Data Strategy roadmaps together, dynamically, on an ongoing basis.
A Data Governance (DG) program plays a central role in organizations and is essential for aligning Data Strategies and ensuring consistent data practices across information. DG establishes roles, policies, and procedures for using and managing data assets.
Each contributing organization must have an existing DG program supporting Data Management practices. For instance, Williams-Sonoma and Whole Foods both need reliable Data Quality processes.
Partner Governance programs must be able to adapt to new business agreements. When sharing data, companies need dedicated data stewards to maintain accessibility and compliance across organizations.
Additionally, partners will require joint external governance. This kind of program would handle partner relationships, issue resolutions, and coordinate data activities.
Key Technical Capabilities for Data Collaboration
With a solid Data Strategy and Data Governance program in place, organizations must then establish technical capabilities for effective data collaboration. Primarily, partners will need to leverage cloud computing and multi-cloud environments to handle shared big data access and processing. Key technical requirements include:
- Self-Service Analytical Tools: Self-service analytical tools allow partners to access and analyze shared data on demand. They include data catalogs that inventory critical data sets and dashboards that simplify data visualizations. Partners need to enforce and execute good metadata management to properly catalog, describe, and govern access to these shared data assets.
- Data Integration: Operating on shared data requires communication and integration among each partner’s data systems and Data Architecture. A unified Data Architecture that allows seamless sharing and blending of datasets from disparate sources is essential.
- Knowledge-Sharing Tools: Data collaboration relies on organizations’ abilities to connect with stakeholders and converse about and interpret the data. Knowledge-sharing tools give these functionalities through centralized references like business glossaries. They also include project management platforms that alert data stewards about issues and provide process flow to resolve issues and handle change requests.
Implementing these key technical capabilities related to self-service analytics, data integration, and knowledge-sharing is very important for a successful data collaboration partnership.
Implementing a Data Collaboration Partnership
Upon combining core requirements and key technologies, organizations can start putting together a partnership with another company to share data. Steps include:
- Choosing prospective third-party partners: Choose prospective partners based on the Data Strategy and initiate a business relationship to share data. It takes time to establish agreements, understand each party’s data policies, and build trusting relationships. Data exchange platforms, like Gaia-X, Dawe-X, and Transformers can facilitate connecting with potential collaborators.
- Defining the collaborative framework: Partners must enable the shared data ecosystem by aligning combined roles, processes, and technologies with individual strategies and Governance needs. Common frameworks include the construction of a joint Data Management policy. These can take many different forms, such as using a trusted intermediary or creating a data pool.
- Ensuring technical capabilities: A unified Data Architecture is needed to publish, integrate, and consume shared data assets. This requires self-service analytics tools, data integration pipelines, security protocols, knowledge repositories like business glossaries, and AI functionalities. Individual partners and the collaboration framework must have these functions.
- Starting with a proof of concept: Begin by targeting a narrow and high-value business use case, to pilot data collaboration. Implement an iterative approach, using relevant metrics to measure progress and optimize processes over time based on feedback.
By methodically going through this implementation process and establishing an effective collaborative data ecosystem, companies can unlock new value and benefits that would not be possible by going it alone.
Unlocking New Value Through Data Collaboration
Data collaboration promises many benefits. Here are some of them:
- Better AI model training: Data collaboration allows organizations to share current and accurate data with third-parties. This provides a wider range of up-to-date, contextually relevant data for training AI models.
- Data enrichment: Data enrichment, a type of data integration that appends existing datasets to fill in missing details, improves Data Quality. For example, Williams-Sonoma may have customers’ credit scores that Whole Foods could use. Then Whole Foods can access insights on customers ordering pizza fixings on a budget.
- Targeted use of resources: Companies can streamline their Data Management and make it more effective. As an organization accumulates more data quickly, it runs the risk of increased errors, security breaches, and inaccessibility. Data collaboration allows companies to concentrate resources on their most critical internal datasets. For example, Williams-Sonoma could focus on customer data for cooking workshops, while accessing Whole Foods’ data on those same customers’ grocery purchases — preventing duplicative efforts.
While promising, data collaboration also presents significant hurdles that partnerships must be prepared to overcome.
Data Collaboration Challenges
Data collaboration can prove difficult. Partnerships can struggle with issues, such as:
- Data Literacy gaps: Low Data Literacy among individuals and organizations can hinder data collaboration. Misunderstanding or mistrust of data can lead to misuse, avoidance of data, and confusion over what is shared between partners.
- Security and Privacy Risks: Sharing data with third parties raises security challenges. Organizations must carefully safeguard against exposing personal or sensitive information through improper data handling. Storing and accessing externally shared data also creates the potential for privacy breaches or unauthorized access.
- Cross-Functional Alignment: As more stakeholders interact with the shared data, maintaining clear communication, aligned priorities, enforced ownership/accountability, and flexibility becomes crucial but difficult. Overcoming this requires dedicated programs and strong partner relationships.
Through proactive measures like improving Data Literacy, enforcing stringent security, and enabling cross-functional collaboration, organizations can overcome these data-sharing obstacles and build an enduring data collaboration ecosystem.
Conclusion
Interest in data collaboration will continue to grow in the next few years, especially with cloud and AI technologies. Companies will capitalize on data capabilities that have been unavailable to them and see their effectiveness improve.
Setting a good foundation with core requirements and technical capabilities will set the path for reaping the benefits of data collaboration. They will also mitigate the challenges that can make data collaboration frustrating.