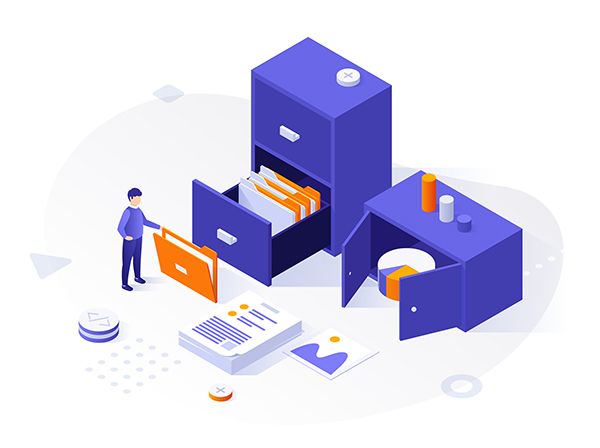
Metadata powers effective action on information by providing context, content, and structure. When this information is managed well, data consumers can find and get the correct data they need. As Atlan Prukalpa Sankar states, “Metadata is the glue that can bind the modern data stack together, the layer that will allow increasingly diverse, siloed tools and people to collaborate effectively.” Grasping the who, what, when, and how of organization-wide data means doing metadata management well.
With the growing role of artificial intelligence (AI) in the marketplace, advances in big data analytics, and changing regulations, companies must look at managing their data more effectively through metadata. Grand View Research estimates that the market for metadata management solutions was $8.05 billion in 2022. Expect this value to quadruple by 2030.
In DATAVERSITY®’s Trends in Data Management research paper, about 66% of those surveyed used Data Governance as a main metadata management use case. Add to this reality an increase of corporate data in the cloud, a hunger for new insights, a demand for data catalogs, and a need to deliver data faster, and better metadata management will move to a top Data Management priority.
In the past, metadata management meant inventorying information passively logged by applications. These days, active metadata assists people and algorithms in analyzing data and detecting patterns, connecting the dots for dozens of use cases, and creating recommendations. Now, more than ever, metadata management understanding is necessary for good data creation, transformation, usage, and delivery.
What Is Metadata?
According to Gartner, metadata is “information describing various facets of an information asset, improving its usability through its life cycle. It provides an understanding that unlocks the value of data.” This understanding comes from putting the data in context, allowing it to be reused and retrieved for multiple business uses and times.
Metadata exists in various structures: table headers, legacy applications, configuration files, business terms, metrics, lineage, and data models. Likewise, companies categorize metadata according to different uses. Some include:
- Technical: Technical metadata is commonly used with passive metadata for data operations (DataOps) to improve data communications, integrations, and automation. It includes:
- Operational: Information about data movement and usageProcess: Details about loading the data into storage
- Structural: A basis for creating and maintaining data dictionaries
- Business: A common language that is easily understood by non-technicians
- Provenance: Data traceability and origin
- Administrative: Data access and usability
- Social Metadata: Information about dataset popularity and authors
Good Metadata Management
Creating or pointing out more metadata does not make that information useful. Instead, good metadata management must be based on a solid foundation.
Properly managed metadata, whether from an old-fashioned card catalog or a computer application, simplifies resource descriptions and provides a common vocabulary to understand relationships between data.
Good metadata management, as noted by David Kolinek, “creates the context for other data elements, providing a complete picture of the data.” This holistic view allows for organizing and locating data, understanding its meaning, and maximizing its value.
Critical components of metadata management include:
Metadata Strategy
The 89% of organizations adopting or planning to adopt a digital-first business strategy will want to include a metadata strategy. A metadata strategy ties into the larger Data Strategy, a pattern for making Data Management decisions.
A good metadata strategy needs to include why a business should track metadata and prioritize key data components. Critical considerations in implementing a metadata strategy also include business drivers and motivation, metadata management maturity, and metadata sources and technologies.
Metadata Capture and Storage
Good metadata management requires identifying all internal and external metadata sources and what the business is trying to capture. These tasks tie into Data Governance, a formalized practice connecting different components that increase data’s value.
Data Governance focuses on balancing data accessibility with security. Knowing where to find data with metadata underlies an enterprise’s inventory of its data and how each dataset is described. This information informs metadata integration and publication.
Metadata Integration and Publication
Metadata integration and publication describe an implementation of the metadata strategy for data stakeholders and consumers. Prioritizing fields through an established metadata standard and emphasizing cohesion among diverse metadata collections make metadata integration and publication easier.
As this Getty article mentions, metadata standards affect how easily people and machines can find data. Companies with good metadata management use these standards to help data consumers better locate and obtain information with the following tools:
- Data Catalogs: Data catalogs centralize access to an organization’s available assets through a data inventory captured through metadata. Consider data catalogs as a one-stop shopping place to locate needed data sets and get guidance on them. In addition to data, data catalogs facilitate metadata improvements. Some systems use data catalogs to enrich metadata to handle DataOps better. For example, smart platforms can use enriched metadata, rerouting data flow for faster delivery.
- Business Glossaries: Firms use business glossaries as a common way to align data producers and consumers on internal terms and their definitions. Metadata managed in a business glossary becomes a backbone for ensuring technology and business understand each other. This resulting metadata layer enhances shared communication, exchange, and understanding of the business glossary. Add machine learning capabilities to the business glossary and company-wide data platforms, and algorithms can identify suggested terms and phrases to add or existing ones to modify.
- Data Lineage: Data lineage describes information on data origins, movement, and characteristics. Published feedback on data lineage enhances regulatory compliance by showing who has accessed the data and the likely sources of problems. Furthermore, data lineage helps show the interrelationship of diverse types of metadata, clarifying their relationships with business processes and information security. As data ecosystems have become more complex, organizations tap into active metadata used by algorithms to improve DataOps.
Metadata Management Needs Governance
Enterprises need holistic Data Governance, including governed metadata to support metadata management. Such governance activities need to focus on metadata roles, responsibilities, standards, lifecycles, statistics, and how operational activities and related Data Management projects integrate metadata.
Without governance, metadata is unhelpful when describing data because no one can interpret it due to confused meanings. Good metadata governance, with formal processes that execute and enforce its management, helps achieve and maintain metadata quality.
Fundamentally, metadata is only as good as its quality, according to Bob Seiner, president and principal of KIK Consulting and Educational Services. To get to an adequate level, Seiner suggests focusing metadata governance on three areas: the quality of the definition, the production, and the use of the metadata.
Use good metrics that validate metadata governance activities in these three areas. Making comparisons over time systematically identifies strengths and improvements needed. In addition, this kind of feedback contributes to the company-wide Data Governance by backing up its ability to perform an impact analysis, construct an audit trail for compliance, and provide trusted data.
“Just Enough” Metadata Management
Give “just enough” consideration to metadata management. Spending too few resources on it “will progressively compound retrieval issues and further stress organizational efficacy,” says Donna Burbank, managing director of Global Data Strategy Ltd. Throw too much at metadata management and product fundamentals, and business stakeholders will suffer. Consider the following:
- Cost: Too much metadata management results in overspending. Beware of shelling out lots of money for the new shiniest metadata tool to inventory enterprise data and having it sit unused. Considering that 80% of metadata users come from the business units, get an application its members will operate. Too little money allocated for metadata management, especially on organization-wide metadata creation and usage, causes more significant issues. For example, if a machine learning application misses enough metadata that describes an image, it can fail spectacularly with critical data elements (e.g., labeling a muffin as a puppy).
- Irrelevant: Nothing can be more disheartening than initially investing in metadata management only as a one-time project. Internal and external users then ignore the firm’s metadata, relegating it to the dusty corners of a bookshelf or the dark recesses of a computer’s archives. Without an ongoing commitment to knowing data’s inventory, lifecycle, characteristics, relationships, and roles within a business, the resulting metadata becomes outdated, even with automated discovery.
Conclusion
Executives and managers must manage metadata effectively. Compliance through Data Governance requires it.
Furthermore, big data needs metadata management for its handling, value, and delivery, while AI and machine learning need metadata management for training algorithms and successful task automation.
Good metadata management becomes critical to entrust, secure, and make valuable business data. Auditors, governments, customers, and other stakeholders demand this.
Image used under license from Shutterstock.com