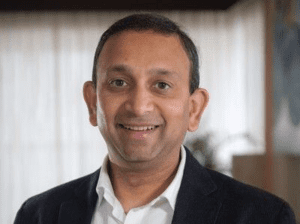
More and more enterprises are looking to automation and AI to deliver new efficiencies and give their organizations an edge in the market. Data is the engine that powers both automation and AI. But data must be clean and user-friendly for these systems to work effectively and deliver on their promise.
Lots of organizations are struggling with their automation and AI projects. Often though, it’s not a problem inherent in these systems – it’s a problem with the data that’s feeding these technologies. Messy, low-quality data can wreck any automation or AI effort, ensuring that any actions or recommendations are unreliable or incorrect. For automation and AI to succeed, they have to perform accurately and provide valuable insights. In order for that to happen, they need to draw on – and produce – clean, user-friendly data.
Here, I’ll discuss why quality, complete data is so important for automation and AI, focusing on this data from an output perspective.
Quality Data Coming from Automation and AI Systems
What is user-friendly data? The most important characteristic is data that analysts, IT generalists, and decision-makers within a company can actually understand. This doesn’t mean that the data is being fully interpreted by a technology for the users. It simply means that there’s a baseline context explaining what value the data really offers, why it’s being presented to them, and what they might be able to do with it.
We’ve all heard the old expression “garbage in, garbage out.” Messy, incomplete data put into automation and AI systems will produce poor outputs that are impossible to make good sense of. To avoid this, organizations need to ensure that their data meets essential quality dimensions:
- Completeness: Data sets must contain all necessary information for accurate analysis and action.
- Consistency: Conflicting records in data can lead to costly automation errors.
- Timeliness: Data must be up-to-date to ensure current and relevant decision-making.
- Accuracy: It’s critical that data correctly represents real-world conditions.
Consider the impact of these principles in various sectors:
- Healthcare: If automation and AI systems have incomplete patient history data, they could make inaccurate treatment recommendations.
- Financial services: Low-quality transaction data could trigger unnecessary fraud alerts or overlook actual threats, impacting both security and customer trust.
- Retail: Automation might reorder inventory based on faulty sales data, leading to stockouts or overstock.
Example: AIOps and Security Alert Monitoring
Here’s an example at the confluence of automation and AI: Imagine a company is leveraging an AIOps platform to automate its IT operations. A big component of the AIOps tech is security alert monitoring. The AIOps platform tells the IT admin that there have been 37 security alerts today. With incomplete historical data (input) training the AI, the output information lacks proper context, effectively rendering it useless for the admin. Are those 37 alerts more than average? Less than usual? Should the IT admin take any action? If so, what should they do?
If the AIOps has complete, digestible historical data that it’s training from, it can fill in the full context and answer such questions for the user. For instance, it might tell the IT admin that the number of alerts is far above average, or that the admin needs to take action to safeguard their organization. It could also suggest taking a closer look at the perimeter cybersecurity systems to ensure they’re not being penetrated by bad actors.
Alternatively, the AIOps could tell the user the exact opposite – 37 alerts is well below average, there’s no immediate concern for security, and no action for the admin to take. In either case, the context is clear.
With user-friendly data, there’s no guesswork or uncertainty about what information means. It’s explainable and actionable, even for non-experts.
Data Governance and Management Practices
To ensure the data that feeds into AI systems is clean and trustworthy, robust data governance frameworks are essential. These can include:
- Data stewardship to monitor data quality and ensure its integrity.
- Data lineage tracking to understand the origins and transformations of data used by AI and automation.
- Automated data validation processes to detect and correct errors in real-time.
Quality Data on the Front End Enables User-Friendly Data on the Back End
Automation and AI will transform most industries in the coming years. But for these technologies to deliver on their potential, enterprises must prioritize the quality and usability of their data. Good data on the front end will lead to clean, user-friendly data on the back end that provides the necessary context for meaningful insights, enabling teams to make informed decisions. By addressing data quality issues, businesses can unlock the full power of their automation and AI efforts.
The Long-Term Business Impact of Quality Data
High-quality, user-friendly data doesn’t just improve AI accuracy – it drives real business outcomes, from improved efficiency and reduced risk to smarter, data-driven decisions that translate into a stronger competitive edge.