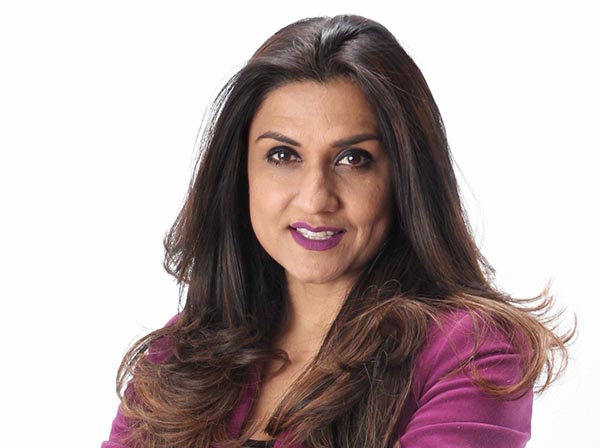
Click to learn more about author Asha Saxena.
It’s 2019, will this be the year your data team starts using and implementing machine learning and AI for your business? If you have data-savvy analytics talent using tools like Alteryx, Tableau, Informatica, Qlik, Microsoft Power BI, TIBCO Spotfire, R or Python, then you have a solid foundation to begin your AI journey. The next step: automated machine learning or (auto ML). Competent, motivated business intelligence and analytics professionals can raise their skill levels to drive better outcomes with automated machine learning platforms. According to Raghav Ramesh, Machine Learning Engineer at DoorDash’s answer on Quora :
“The growth of AutoML like tools which provide powerful machine learning models as a plug and play solution without the need for deep machine learning expertise, is bringing the power of machine learning to more and more industries.”
According to a Forrester, inc report from 2017, ”AI-driven enterprises will steal 1.2 trillion from competitors by 2020.” That is a pretty bold claim, but they are not the only research firm making these kind of predictions. In a 2018 report titled Notes from the AI frontier: modeling the impact of AI on the world economy, Mckinsey and Co. analyzed 400 uses cases in 19 different industries to understand the broad use and significant economic potential of deploying advanced deep learning artificial intelligence techniques. It also found that the companies with faster AI adoption and absorption (Front Runners) are creating big economic gains.
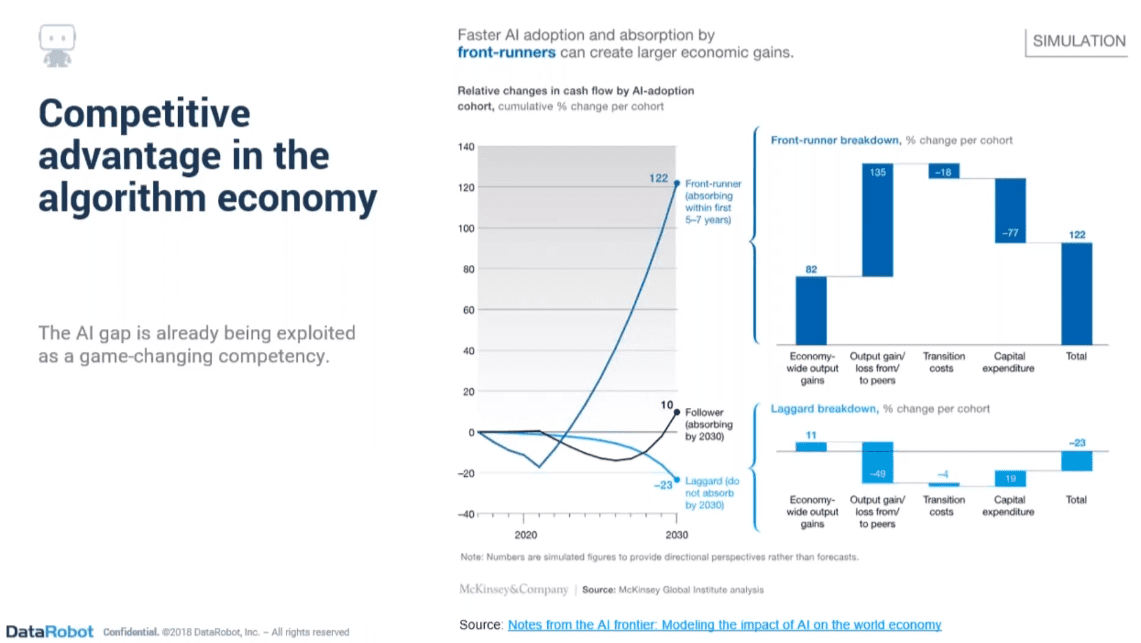
Predictive Analytics has Come a Long Way and Organizations are Taking Note
Jen Underwood, senior director of DataRobot, an automated machine learning software cloud platform recently ran an interesting webinar called From Analytics to AI, where she says that:
“Having been in the analytics space over 20 years, I can tell you that the success stories and the ROI, is … exponential over, some of the ROI’s that I would have had when I developed a data warehouse back in the day. I would have loved to have seen these ROIs that I am seeing, these are the types of uses cases when you start applying advanced analytics.”
So, when we think about the economy, now into the digital era, its increasingly technically and culturally driven by data. It’s now about taking your organization to the next level of adopting a data-driven culture. That is what these leading companies are doing, and it’s not just reducing time to action, but they are actually using algorithms to optimize outcomes.
The forward-thinking organizations are exploiting the gaps between their competitors and increasing their access to information and capabilities. Some of these companies will try to research and reverse engineer what they think their competitor is doing with an algorithm for their business and then develop a better one. Organizations are getting smarter and are simulating those environments.
Where is Your Organization with Analytics Maturity?
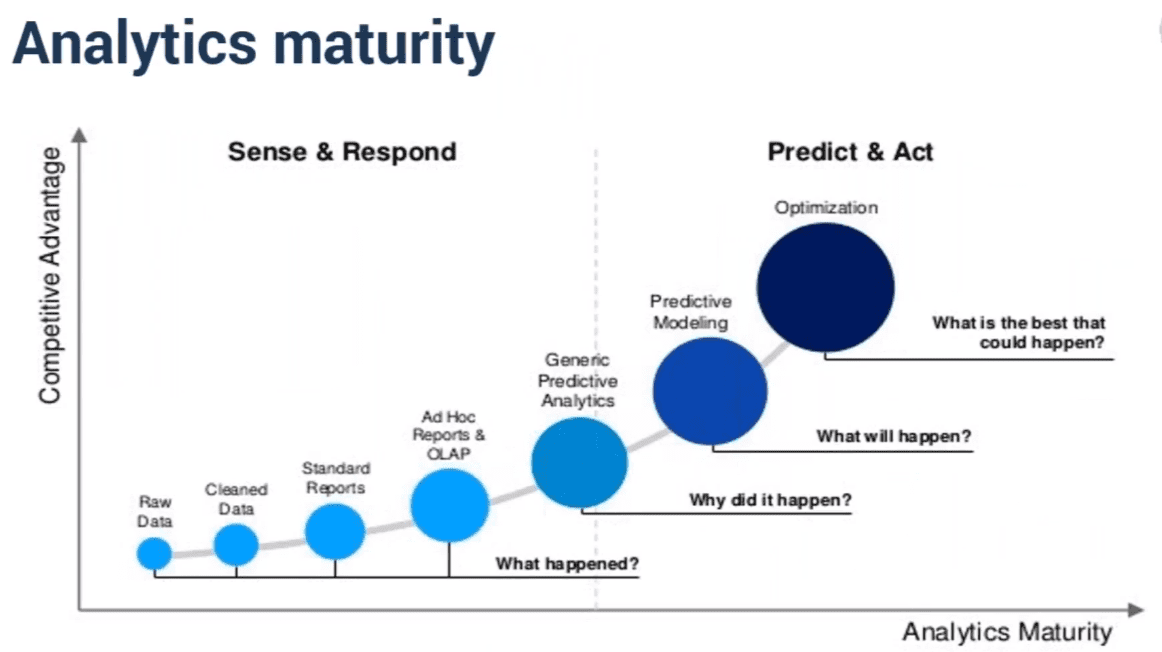
This is the classic analytics Maturity Diagram, where 15 years ago some consultants such as Jen Underwood were dabbling in predictive analytics but the companies were not ready for it back then. Now with the explosion of data and digital economy coming together we are at the “right place and right time” moment for adopting predictive modeling. This has been helping businesses to make better decisions as well as what can be done with data once the information is available.
A recent survey from O’Reilly Media stated that among their executive respondents, they indicated that AI and Machine learning were the number one projects, finally surpassing business analytics. What seems to be happening is The great AI divide; the leading companies are moving faster, making a bigger impact, and gaps are only getting larger between companies and progress. So how do you get your company started on track to using AI?
3 Steps to Becoming an AI-driven Organization
- Upscale your existing team
- Apply automated data science machine learning
- Collaborate with expert PHD mentors
If your organization has not already started hiring data scientists, you should start to think about hiring more as well as upskilling your analytics and data teams. According to Gartner and Forrester, this is the next wave in analytics, the AI wave is upon us. So you can begin to upskill your team members to become “citizen data scientists.”
Employees that have BI and analytics experience already, are in a great position to learn some of these new skills of using automated machine learning. The ability of autoML to automate some of the repetitive tasks of ML compensates for the lack of AI/ML experts while boosting the productivity of the company’s expert data scientists.
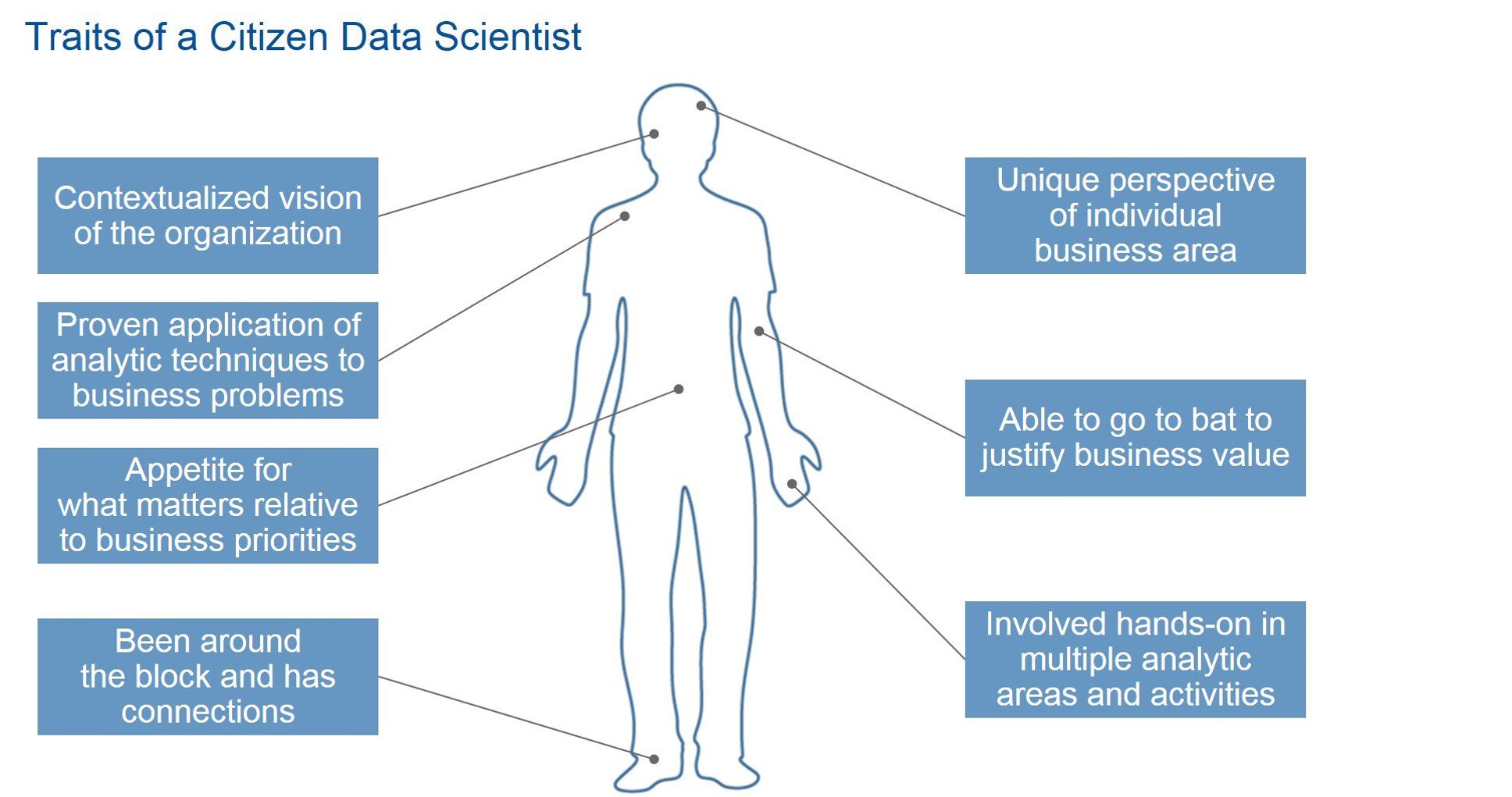
Citizen data scientists will not replace the need to hiring true expert level Doctorate level data scientists, but they may be mentored by the senior level engineers and complement their knowledge. Using automated machine learning to build and train predictive models can be used for a variety of industries. For example, in retail it could be used to improve supply chain forecasting models. The old way of pursuing machine learning, AI models and theory can be very complex, and although some companies may care about having AI for developing research and theory, most want to hire competent people that can it to add value to the business.
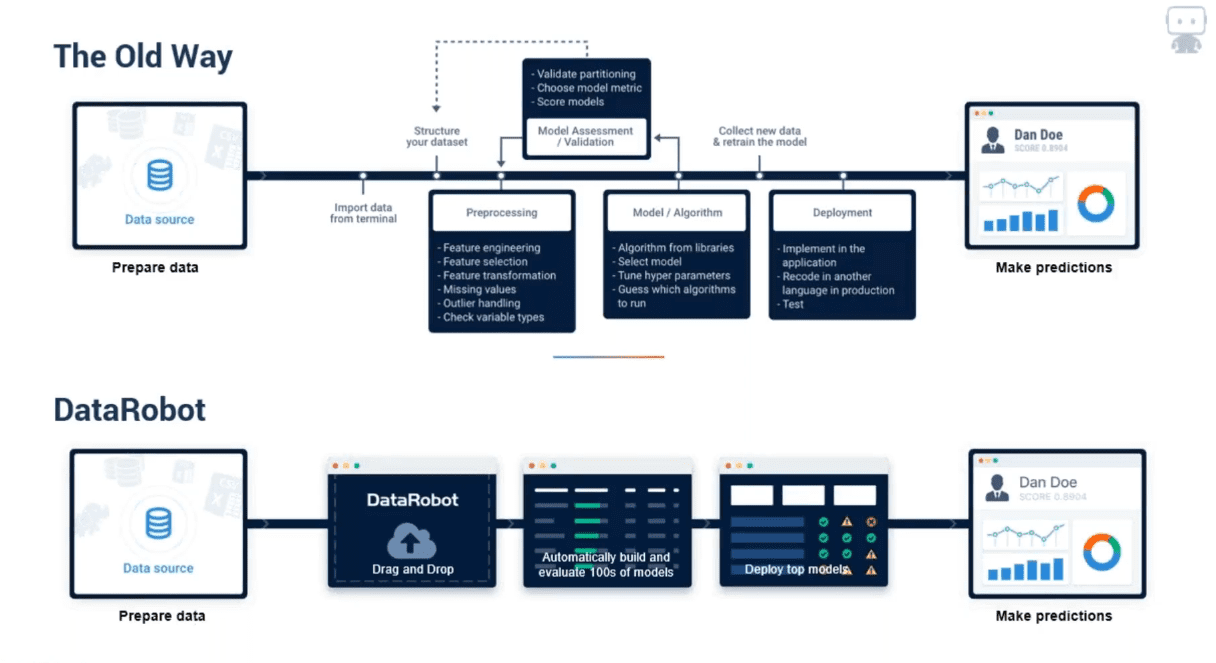
Enterprise machine learning software cloud platforms like DataRobot or Microsoft Azure have recipes or blueprints built into their models designed by real data scientists that know what they are doing. Their software also provides some safeguards (alerting to target or data leakage ) so that when you deploy the models, they will make sure they stay healthy and can be trusted and relied by the business for accuracy and making decisions such credit risk loan applications.
There are a number of automated machine learning software providers on the market, (some are open source like Auto-sklearn and AutoKeras) besides DataRobot (which I have no association to) but for the purpose of this article to educate IT professionals on the benefits of using autoML. I have referenced many slides from the DataRobot webinar. I recommend you try a number of platforms before making a decision as to what is the best choice for your organization.
If you want a more detailed comparison of the techniques, training frameworks, architectures of some of these platforms. I suggest you check out the article titled Auto is the new black — Google AutoML, Microsoft Automated ML, AutoKeras and auto-sklearn.
Google’s Cloud Auto ML is a:
“Suite of machine learning products that enables developers with limited machine learning expertise to train high-quality models specific to their business needs, by leveraging Google’s state-of-the-art transfer learning, and Neural Architecture Search technology.”
Some of the Common Team roles in AI
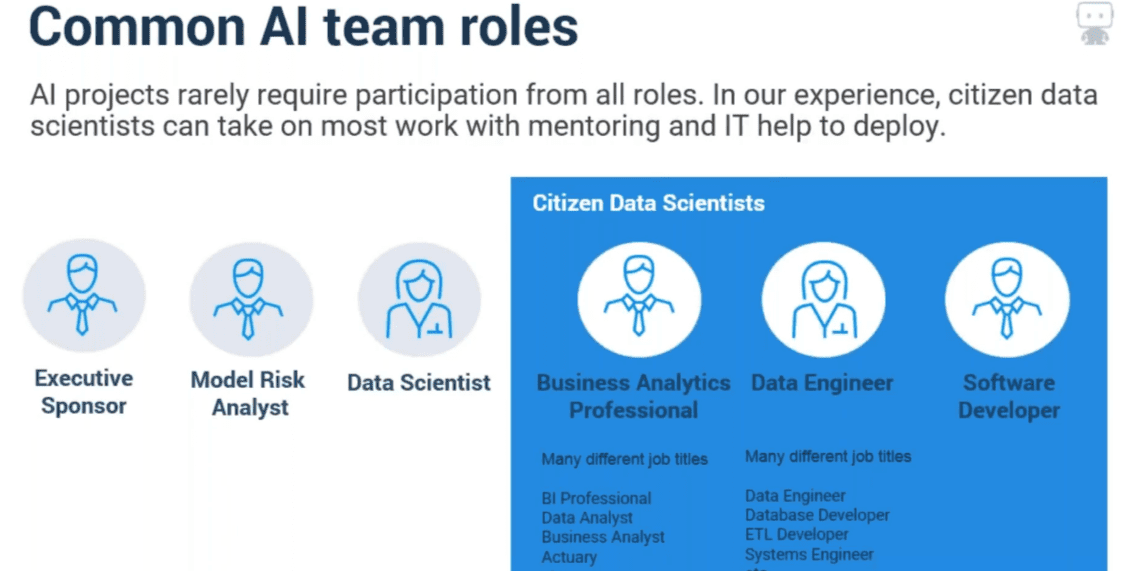
In many organizations as Jen Underwood points out in her webinar, in AI team projects you will have several different types of people involved. There usually is an executive sponsor that will be used to be get budget and by in on the AI or ML initiatives. Especially in regulated industries such insurance, banking or healthcare you may have model risk analyst. Who will looking at compliance and best practices are being applied.
They spend a lot of time on governance standards and documentation. And then you have the data scientist who creates the predictive models. And you will also have professionals in organizations who are Business Intelligence Director or a VP of Analytics.
Companies are starting to have the senior level data scientists start to mentor other teams’ members and establish some of the foundations and best practices. Basically, to start training people to become successful with the group. The learning curve can be little high when you start to analyze, understand and interpret the ML modeling results for the business or data analyst.
And then when we talk about the “citizen data scientists”, a term as mentioned earlier coined by Gartner, has not caught on as a specific role or job title per se in companies yet. What it means is that someone is not a traditional statistician or data scientist, but they are now starting to apply machine learning in their roles. So, the types could a data engineer, database developer, software developers, who are infusing AI into their applications.
As Underwood says:
“Finding and retaining data scientists is often the hardest part of implementing AI and machine learning in an enterprise. With automated machine learning, you empower data analytics professionals and software engineers to create advanced predictive models (all without having them to learn to code or understand when and how to apply certain algorithms) and embed AI into applications – all while making existing data science personnel more productive and satisfied.”
Some common objections that can delay companies from getting started with AI are:
- We don’t have a data scientist
Historically you would have needed one. And you will eventually need to hire an expert one, but automated machine learning platforms such a DataRobot and others discussed here offer resources and training for getting started. The automation removes a lot of the tedious work. Where you can start to focus more interpreting and analyzing results.
- We don’t have enough Big Data
This might be a bit of a myth, but you not need extreme volumes of data to get high value results. Meaning many companies have millions of records and data points, you don’t need billions records in massive Hadoop data lakes/stores to find insights and start using machine learning.
- We don’t have completely clean data
Now clean data is important, and the machine may assume it is correct. However, if it is not perfect, software can find and optimize the data for algorithms. And it will tell you what was done so you can continue learning.
- Change management is hard
Yes, change can be uncomfortable. The famous Moneyball Movie, Billie Bean changed the way the Baseball world was recruiting and picking players from gut feelings to using stats and data metrics, Now the sports industry is very data driven. To get buy-in from any new technology, it is idea too good to start answering the unknowns and get some quick wins. Bring AI into the tools they already know.
- IT won’t support us
This has been a struggle for enterprise organizations for decades even in the self-service BI world tools years ago. Getting people to start using and experimenting with more cloud offerings Starting point to show the promise and value.
- Executives don’t understand AI
There is a lot of hype and misinformation out about what AI is really. That some CEOs don’t get it and will just shut down. It can be helpful look at it from the perspective of hiring people “5 data scientists will cost x amount etc”, or how the CEO is measured and how you can make him perform better as the leader with outcomes and revenue.
TD Ameritrade Machine Learning Example
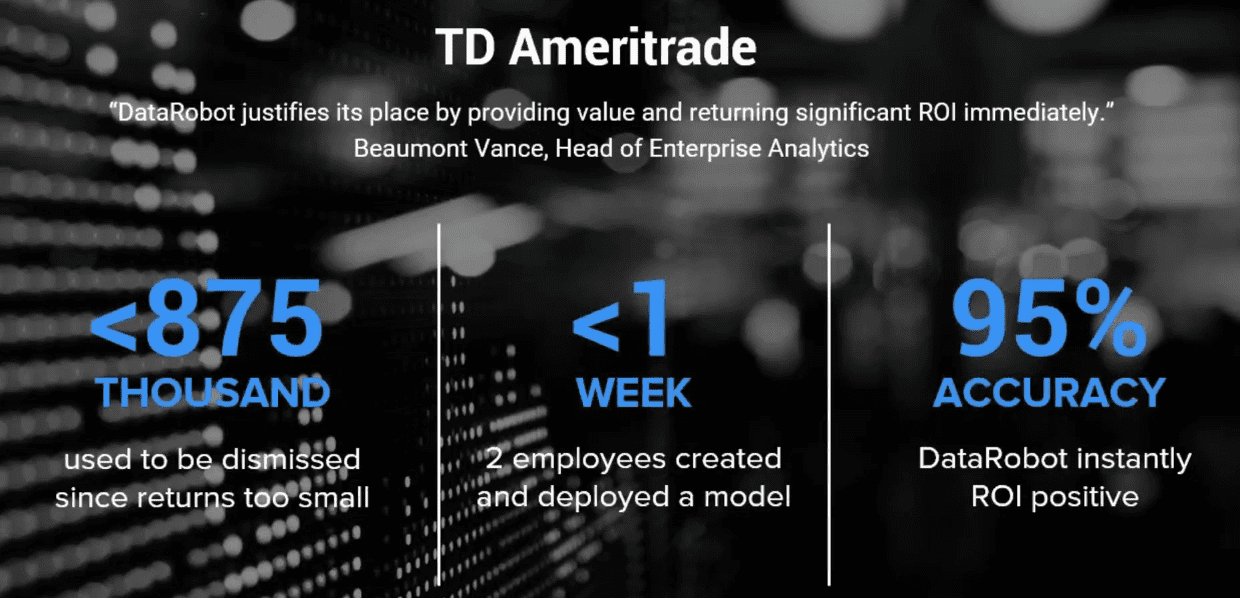
The financial industry has been an early adopter of using new technologies, they are very geared towards improving the bottom-line to get an edge. One example was from TD Ameritrade and any new projects that got sent to their existing data science team, had to have at least $875,000 in value or it was just to small and not worth their time.
But using this platform they took one or employees, such as someone that does Tableau or Microsoft Power BI work, and those folks in less than a week, created a model with 95% accuracy. When you can find quick wins like this it can get more excited investing more resources or people. “DataRobot justifies its value by providing value and returning significant ROI immediately” said Beaumont Vance, Head of Enterprise Analytics for TD Ameritrade.
Steward Healthcare Example
Another case study example that Jen Underwood mentioned was healthcare organization named Steward Healthcare, start using the machine learning platform and they were able to find $10,000,000 in annual cost savings for 8 of their 38 hospitals. By finding small reductions of just 0.1% in the length of stay for each patient.
Having data that helps predict expected length of stay for certain diagnoses allows doctors to take proactive measures and get patients home sooner, without sacrificing quality of care.
“We are using DataRobot to make some pretty huge decisions at Steward Health Care. DataRobot is very much a part of our growth strategy. It’s uncharted territory for healthcare.” said Erin Sullivan Executive Director, Steward Health Care.
The Path to Becoming AI-Driven
There are a few different starting points, it all depends where your company is in its big data AI journey. Usually a cultural change has to happen within the organization. In this data world, at some point management needs to make the determination to say ‘hey we need to use AI to help us make the best decisions and compete in the tech infused world’.
At first someone is skeptical of it, or they getting outperformed by competitors, or they see a competitors earnings report and wonder what are they doing so well. So usually a company will start with exploring or developing an AI proof of concept (POC) for an enterprise application. From there many companies will prioritize a good business use case to start, and for the business and executives to understand.
Then once you some results from one, people will usually say “wow that was interesting, what else can we do?” After some results have been proven. Then the next step typically is the c-suite wanting to expand this out into other parts of the enterprise, into some of their existing governance processes, and their analytics center of excellence can start to add “citizen data science” into those strategies.
And then working closer and closer different units of the business. Some companies are secretive, Lending Tree used this process and actually put out a press release in 2017 about how LendingTree Builds the 200 Millionth Predictive AI Model Delivered on the DataRobot Cloud. So Lending Tree uses the cloud to deploy machine learning models to uncover hidden opportunities and predict future outcomes of the likelihood of a consumer taking a loan from vast amounts of data.
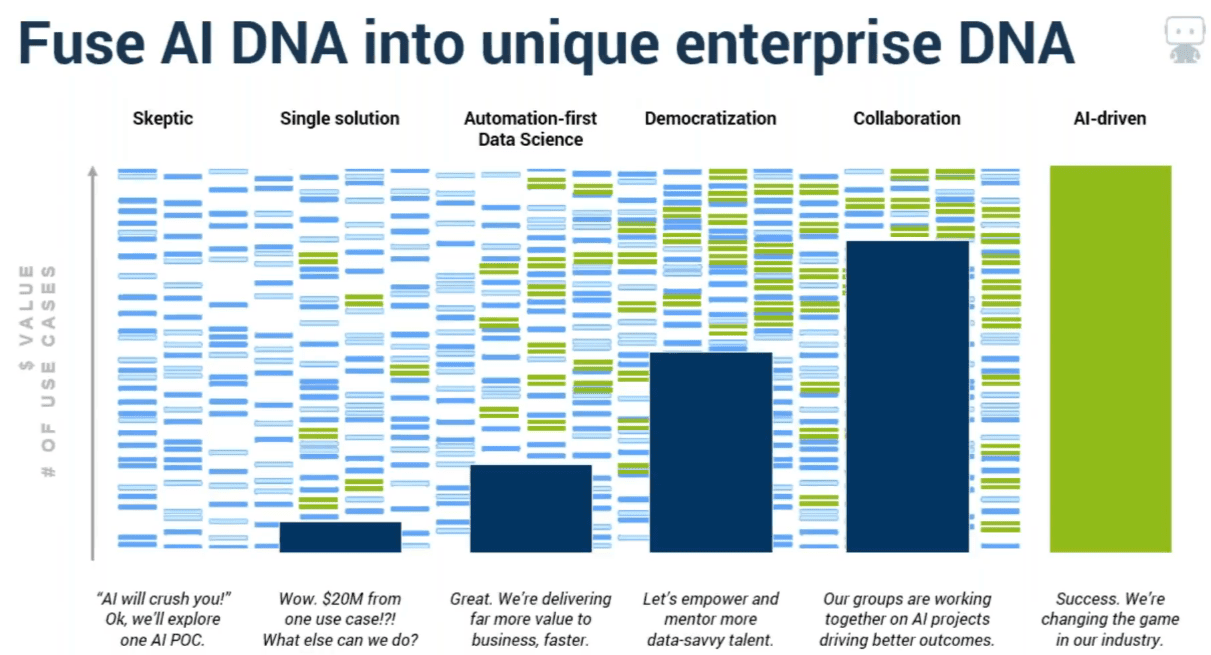
What Do You Need To be Success with Starting AI Initiatives?
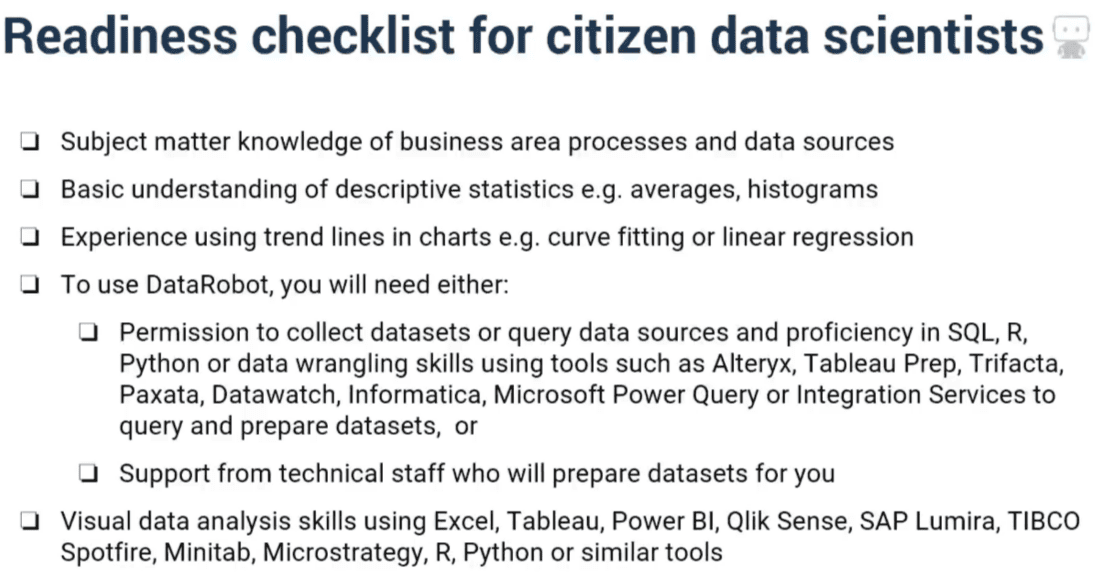
Guidance on how to Identify AI Use cases and measure results based on what outcomes can be achieved, You also need data savvy talent to collect and properly prepare data. Finding the right “citizen data scientist” you should look for experienced data engineers or analytics professionals, and ones that are tasked with solving complex problems with data.
Conclusion
Training and ongoing mentoring to understand, interpret, and effectively explain AI results and new use case opportunities to the C-suite, and managers of the business. And support from technical data science professionals to deploy and integrate machine learning models into production systems. Ultimately “Executives and technical people need to work together to fully operationalize and deploy machine intelligence within the enterprise.” says Nir Kaldero, Head of Data Science and Vice President at Galvanize, Inc. You can subscribe and listen to my Dive With Data podcast interview with Dr. Anthony Scriffignano, Dun & Bradstreet’s Senior Vice President and Chief Data Scientist, about “How companies Can Learn from Big Data”
All images are credited to DataRobot