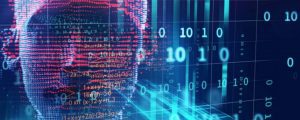
Click to learn more about author Asha Saxena.
You can’t seem to get away from seeing tech headlines about how many companies want to adopt or use AI for their Business Intelligence applications and technologies. From a Forbes article titled ‘“Preparing Your Business For The Artificial Intelligence Revolution” to a Martech advisor one called “How to Reshape Your Business Strategy Around AI.”
But how does a company plan and go about implementing Artificial Intelligence technologies into their business? The first step is to figure out the reason for wanting to use AI in the first place. Digital organizations are beginning to experiment and incorporate different types of AI applications such as Artificial Neural Networks, or Machine Learning into their processes and products for tasks such as image recognition, NLP, sales forecasting, autonomous driving, robotics, patient data processing, fraud detection, personalized recommendations, and chatbots. Forbes contributor Terrence Mills, wrote an article called “Machine Learning vs Artificial Intelligence: how they are different” which provides good overview summary of the what each does.
MIT Sloan review discussed why AI implementation is challenging. The path to implementing AI can be a costly and a long-term investment. It is not as easy as moving your business into the cloud or implementing a new Big Data Analytics tool. One of the main reasons that implementing AI can be cost prohibitive and complicated is due to the fact that every use case or application of it needs different algorithms and technology tools for it all to work within the system or processes to make it successful. There is no one size fits all AI algorithm.
If your company is one of the 84 percent that believe that investing AI will lead to competitive advantages and you are looking to explore your options for adopting cognitive technologies such as AI into your business, then here are a few tips:
- Inform yourself and, your management team: One of the keys understanding the differences between the hype and real potential of AI, is by research. Figure out which processes and problems could be automated or made more efficient by implementing AI.
- Don’t be afraid of experiments: Companies that evolve will try out new tools and technologies to see what works, but conducting experiments using Artificial Intelligence will be nothing like other digital technology experiments. Definitely do a proof of concept, but failure can be just as instructive as success in the end product.
- Assessments and analysis are crucial: Are the first AI initiative results satisfactory? What comes next in your companies adoption of new technologies? AI is a relatively new technology and many corporations are still trying to figure out how to use it effectively.
- What are the priorities for applying AI into the business: Obviously as AI becomes more widespread, the question becomes which business units or projects will reap the most rewards from adopting it? Figuring this out is a crucial part of the process.
- More investigations will be needed: If you really want to implement Artificial Intelligence as a capability into your business, then some important questions will need to be addressed Should you look for ways to incorporate using AI more widely across all aspects of your business or start within one specific process or business operation?
Where is Your Company’s Technology Stack?
Has your company invested in building out some Big Data systems such as Hadoop, and is now wondering how the company can take advantage of AI? Are you looking to make better sales predictions or find latent data patterns? Perhaps you need to do a capabilities assessment of your company’s core technology stack. What capabilities do you have, how can you leverage those tools, and what do you need to move forward? Or, have you implemented a Big Data solution and need a strategy to provide a return on investment?
Moving forward with AI programs or initiatives is different from other technology exploits because every application of it will require a unique set of software tools, algorithms and not every company will be at the same level of AI adoption. Although different industries may use similar technologies and approaches, a Machine Learning algorithm that is written for a pharmaceutical cancer product to scan different medical data sources to detect adverse event reactions would not be interchangeable with one that a Mortgage lender would use to find inconsistencies in loan applications. For companies to move their AI maturity forward they need to choose their project initiatives wisely.
For every application of AI, the system will need a unique training process, as it looks for patterns in the data. Some Artificial Intelligence frameworks take a longer period of time to get right. The key is to iterate and count incremental improvement, rather than expecting a data miracle because you have implemented expensive systems. There have been some great advances made in computer vision (face detection algorithms, image retrieval, biometric identification, surveillance cameras, etc) by using Deep Learning and Convolutional Neural Networks. But these systems need very large data sets and extensive training to improve the accuracy of image recognition and classification. Computer systems can now re-create the facial structure of long dead pharaohs based on facial recognition software, but the initial use case may have been to determine the differences between 2 different faces.
- Inform Yourself and Your Team
According to a global study conducted by Statista, which noted that 75 percent of companies surveyed think that AI adoption will help open up business opportunities. The hype is real, with tons of companies hosting workshops and attending tech conferences to further education. Despite all of this, some corporations and individuals may be a little confused about how AI, Deep Learning and Machine Learning all work. So for stakeholders that don’t have a Ph.D. in computer science, bringing everyone’s data literacy up to the next level to understand how all these all work together is recommended. Such as:
- Deep Learning is a subset of Machine Learning
- Machine Learning is a subset of AI
Another thing to keep in mind is AI is a perpetual cycle, with no start or end. AI will use a mix of different software tools and techniques and when directed towards certain types business issues or processes, it can help to deliver more personalized experiences, at scale. Airbnb uses Machine Learning algorithms to improve its guest search results as well to provide hosts with pricing prediction recommendations (based on historical booking data) all for the purpose of matching more host to guest bookings (conversions).
2) Don’t be Afraid of Experiments
In order for startups and organizations to make AI work for them, they need to be open to finding new and unconventional business needs. The starting point for experimenting with AI technologies is to really look at data from a historical viewpoint. Are there any elemental or hidden causes for the results in the data? How can the characteristics or signals be tracked in the network system? Additionally, a culture change often needs to happen to encourage stakeholders to trust machine intelligence. The way work is being done is always changing and companies need to figure out new business processes that complement these market shifts and internal process and regulatory changes, as well as seeing opportunities to increase performance and grow revenue. Many companies have done a pretty good job of embracing digital technologies and experimenting with new tools & systems, but to have success with AI there a few areas that will differ:
- The network architecture setup and support: Just as Facebook coined the mantra “Move fast and break things” a similar thinking needs to applied to embrace AI. Just as fast you run experiments and fail, you need also be learning from them equally as quick. Iterate, iterate, iterate. Some companies do not invest fast enough in building out all the necessary supporting IT server storage infrastructure and processes necessary to support a Machine Learning product test. Sometimes the AI experiments can take only a few weeks to start showing some results, and the AI network architecture framework needs to be ready when it is rolled out. Companies should take advantage of the Cloud environment and also research all the AI technology platforms that are out there. Co-development partnerships can make sense.
- Focus outcomes that will bring the best bang for your buck: How will running an AI experiment impact your business’s bottom line? Often times the AI programs tend to focus on algorithms and technologies and not enough on actual benefits or results. Get in the habit of measuring the business value of the experiment, meaning implementing an AI test may have worked (from a tech point of view) but the results in cost savings or increased revenue are not enough for it to warrant a change management overhaul, or for implementing the full solution for the initial test process. What’s important is having a framework to determine if the implementation of AI is worth the cost.
- The more you fail, the more you learn: Get in the habit of realizing that with AI programs, not all of them are going to be considered a successful test. Just look at Google with their Moonshots projects, some fail and some bets work out. Sometimes the experiments or projects can be considered an initial failure, but it can sometimes be the spark for a new idea. According to an Economist article about companies embracing and learning from failure states “Intuit, in software, and Eli Lilly, in pharmaceuticals, have both taken to holding failure parties.” Sometimes a failure with an AI initiative can lead to a company experimenting with a hypothesis about predicting “why customers sometimes switch solution providers”, which can lead to learning more information, that can be put into new data sets for new ML experiments.
3) Assessments and Analysis are Crucial
Sometimes it is hard to make a determination as to whether a Machine Learning program has produced definitive results. Or should you extend the testing time period for further iterations or bring in more datasets? You have to be willing to stop or cut an AI project if the cost-benefit analysis does not make financial sense for continuing it. It can get harder after the initial assessment is completed and before implementation of the process in question. The key is following a process in order to ensure that AI will add value. Once a company has developed a successful pilot experiment, how can it proliferate that experience throughout the rest of the company?
Within many startups, this assessment stage can be a bit of wrestling match with departments. Engineering and IT teams probably want to push AI projects forward to show expertise in using leading edge technologies. On the flip side to that coin, the management may want to wait to get a better understanding of the impact of the implementation. Here is a way to please both – try promoting a more transparent testing of approaches and technologies. Inviting organization stakeholders to come and observe AI projects wins as they occur in the lab. This can be a great way to get buy-in from management, to move it out of the lab and into the business divisions. Some companies will establish a separate team within an innovation function while others may set up an AI council that will span across IT and business units. Some companies will add the AI as a mandate to the data divisions since the AI feeds off of data. A solid governance structure helps to get everyone on the same page and to help ensure that teams do not repeat mistakes. Multifunctional participation across matrixed organizations is important. AI is not an island, and you don’t want it to be a skunkworks project either. It’s not like other technologies such as quantum computing or Blockchain which are still in the nascent stages. Google’s DeepMind win on the board game Go and IBM Watson’s win on Jeopardy has helped show and advance AI from being a cool new tech to showing it can be used for business solutions.
4) What are the Priorities for Applying AI into the Business?
Now that AI is becoming popular, it’s common to discover different business units within a corporation, operations, technology and product divisions—each experimenting with their own AI initiatives. With use cases all around, learning to prioritize is more important than ever. Here is a question to think about. Which pilot initiatives share a common AI core or framework that more than one function can leverage? The final goal is to establish AI as a viable business tech capacity that can be brought out of the lab into put into production deployments. This is where a governance structure is indispensable and the difference between success and failure can often be traced not to the technology, but to the change management and governance procedures.
To foster AI experimentation while also imposing strict disciplines on the prioritization process, CIOs and executives should ask a few key questions. Does this pilot project deliver limited, incremental value, or is it just reinventing a process through a smart use of technology and data? Obviously, if you can show an early success with some positive business results or measurable improvements in productivity, management will embrace the program as more than just the next shiny tech object that may sound cool but does not have big impact on the company.
After the business value questions, probe and assess how difficult your program will be completed from a technical standpoint. Does this prospective AI system have a large enough data it needs to learn the patterns? Are there any biases in the data? Is the tech infrastructure able to handle and process different kinds and very large volumes of data? If any these questions answered with a no, then the feasibility of the program may not work so well.
5) More Investigations will be Needed
This last step is where companies are usually deciding does it make more sense to continue focusing their AI experiments within a single functional area, or to apply them more broadly across other business units. Leaders also must pay attention to any new government regulations that may impact liability and compliance as legislative and judicial branches begin to tackle AI related issues.
The key takeaway from this is to consider how your processes, can better evolve and organize themselves with AI as a component or tool. Set up a strong governance process. Measure the processes that you will be improving and get stakeholder buy-in. Ensure that failures as well as successes are part of the learning process. Iterate and be agile. With the proper preparation, AI can be a powerful tool to enhance the capabilities of a business unit, a division, or an entire organization.
Many companies are still in the early stages of figuring all of this out and getting a pilot program out of the innovation lab.
Image used under license from Shutterstock.com