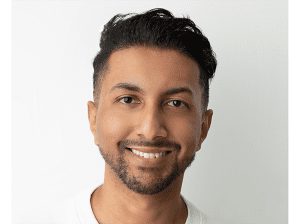
AI’s existential risk is no laughing matter: As much as the tech world tries to make sense of it all, nobody definitively knows whether the dystopian “control the world” hype is legit or just sci-fi.
Before we even cross that bridge (real or not), however, we need to face a more immediate problem. We’re already seeing the emergence of semi-autonomous AI agents responding to their environment and making decisions on their own. Think trading robots that monitor stock market conditions and change how they buy or sell accordingly, or even a work memo or school paper churned out by ChatGPT.
No matter the application, AI’s usual practical issues inevitably pop up. Hallucinated citations, lazy decisions, sudden misdirections, and overall unpredictability continue to hold back trust in these systems – and, diving deeper, trust in the machine learning (ML) algorithms powering critical inferences. ML inferences are often inscrutable to outsiders, and the consequences are seismic. Just look at Minnesota’s lawsuit against UnitedHealth Group for relying on a predictive algorithm that didn’t take COVID into account, or the creditworthiness assessment industry’s massive blunder of bad scores last year.
Trusting unknown inferences is a serious problem, and one that’s all too familiar to those of us in crypto and Web3. For example, imagine the potential profit an arbitrageur could gain from a decentralized exchange by garbling a feed of price information.
Luckily, there is a solution to this trust gap. The answer: Create an inference economy.
What Is an Inference Economy?
While the spotlight has justifiably been on the data powering machine learning’s inferences, what’s missing is a formal arena for nipping any bad outputs in the bud. Grounded in responsible competition, an inference economy commercializes the pursuit of honest, reliable, and secure machine learning production.
At a high level, this comes to life as a marketplace for verifiable machine intelligence, with two layers: a tournament-style competition that allows any data scientist or modeler to improve on an ML model and earn a share of the inference’s generated revenue, and an open marketplace where streams of verified inferences can be consumed.
AI agents or developers building smart contracts or applications will be able to select streams of inferences from a wide variety of proven, community-vetted machine learning models. Oracle risk will be significantly diminished because a bad stream of data would be replaced by a better one in the marketplace, and AI agents would be reacting to well-screened, trusted streams of inferences.
Accountability Starts with Web3
The way this all works is by combining concepts such as Web3 validation staking, streaming payments, and zero-knowledge proofs with the existing, limited competitive ML model. Zero-knowledge machine learning, in particular, allows for both proof of integrity and modelers to protect their models.
Why does this matter?
It comes back to the existential issue of dealing with AI. Trustless systems require battle-hardened technologies and extraordinary security measures. That’s why blending Web3 design philosophies is the only way to hold AI accountable. Grounded in verified machine intelligence and an open-sourced pool of brilliant minds (data scientists and modelers), the inference economy presents a real, attainable antidote to p(doom).
Simply put, a better marketplace of ML models produces higher-performing and more verifiable inferences that businesses can buy and deploy for their own operations. From credit scoring and NFT recommendations to crypto price and sports predictions, organizations of all kinds can unlock massive value from an inference economy that puts trust back into machine intelligence.