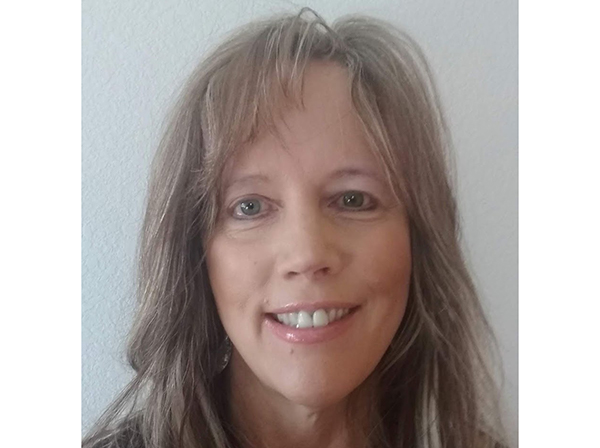
Research scientists and health care professionals are taking part in a new era in medicine. More data is being collected and analyzed than ever before in both clinical and research settings. But as pharmaceutical and health care companies begin to apply AI and machine learning to these, they need considerable computing power (“compute”) and storage. Often, they rely upon services from cloud service providers (CSPs) such as Amazon Web Services (AWS), Microsoft (Azure), Google Cloud Platform (GCP), Oracle, and others, but each provider has its own strengths and weaknesses. The diversity of data and of research objectives makes it unlikely that any single cloud provider’s solution can span all of a typical research organization’s needs and scale, without limit. As a result, the trend in scientific research is to use a multi-cloud strategy.
What Is a Multi-Cloud Strategy?
A multi-cloud strategy is defined by the use of multiple vendors’ cloud services in order to affordably distribute compute resources, improve performance, minimize downtime, and prevent data loss. Organizations can choose the best services from each cloud provider based on costs, technical requirements, geographic availability, and other factors. Companies that adopt a multi-cloud architecture may leverage multiple public clouds in combination with private cloud deployments and traditional on-premises infrastructure.
For research needs like those required for health care and medical science, utilizing a multi-cloud strategy can provide substantial benefits such as boosting innovation power by accessing best-in-class services from the various CSPs, and avoiding vendor lock-in.
A big hurdle encountered by research organizations is issues around latency. Often research data is stored physically distant from the computing resources used for analysis, resulting in slow performance, especially for very large data queries. Because the datasets can be huge, moving or copying them is out of the question because of cost, time, and risk.
In addition to solving cost and latency-driven performance issues, research organizations that are using AI and machine learning have found that each cloud provider’s capabilities are slightly different, and stronger for different purposes. The ability to access the best features of each cloud provider enhances pharmaceutical companies’ and health organizations’ ability to innovate. In fact, using an emerging practice called ensemble learning, multiple cloud providers’ AI algorithms can be leveraged simultaneously to achieve superior predictive performance than is possible with any single provider.
How are pharmaceutical companies and health research organizations using multi-cloud? Here are five key ways.
1. Genomics Research
Genomics is the study of the entirety of an organism’s genes, called the genome. Using high-performance computing and math techniques, genomics researchers analyze enormous amounts of DNA-sequence data to find variations that affect health, disease, or drug response.
Using a multi-cloud strategy enables genomics researchers to select sequence data, transfer, store, and catalog it for reuse. It also helps them store once and access it from any cloud simultaneously, thereby eliminating data movement and realizing cost efficiencies. Researchers can minimize latency by selecting geographically co-centric locations, taking advantage of the best-in-breed tools and capabilities of each of the various CSPs. Thanks to multi-cloud implementations, genomics researchers will uncover novel insights into the biology of diseases and new targets for medicines. Additionally, multi-cloud will aid in the selection of patients for clinical trials and allow patients to be matched with treatments more likely to benefit them.
2. Cell Imaging
In large-scale biological experiments such as high-throughput or high-content cellular screening, the number, and the complexity of images to be analyzed are large and rising steadily. To handle and process these images, well-defined image processing and analysis steps need to be performed by applying dedicated workflows. Multiple software tools have emerged to create such workflows by integrating existing methods, tools, and routines, and by adapting them to different applications and questions, as well as making them reusable and interchangeable.
The Imaging Platform at the Broad Institute of MIT and Harvard, together with industry and nonprofit partners, collaborated to create a massive cell-imaging dataset, displaying more than one billion cells responding to over 140,000 small molecules and genetic perturbations. This microscopy image dataset, which would represent the largest collection of cell images generated by Cell Painting, will act as a reference collection to potentially fuel efforts for discovering and developing new therapeutics.
3. Electron Microscopy
Cryo-EM is a version of electron microscopy that involves freezing samples to preserve biological specimens’ natural structure and protect it from the electron beam. It can uncover detailed images of target molecules and how drug candidates can bind and interact to help guide novel drug discovery. However, processing data on internal platforms often requires complex dataflows spanning multiple networks, ideal for a multi-cloud strategy.
4. Drug Discovery
Similarly, high-throughput screening is used for drug discovery, normally an extremely complex and cost-intensive process. Multi-cloud knowledge graphs have shown considerable promise across a range of tasks, including drug repurposing, drug interactions, and target gene-disease prioritization. A large number of open-source databases are integrated along with published literature to create huge biomedical knowledge graphs.
5. Disease Prediction
Research scientists are leveraging AI and machine learning to generate and analyze giant sets of patient data to highlight key differences between diseased and healthy cells. As a result, they can determine the persistence of treatment and predict disease progression. These processes, however, require long-running GPU compute times in the public cloud, making them costly. And because scientists are accumulating more and more data as they work, the datasets are becoming too immense to be moved or copied while in use. Multi-cloud allows data to be provided via a POSIX layer into the analytics.
As we enter the next age of technology, in which sensors shrink, improve, and proliferate and every patient experience has the potential for informing a future treatment, datasets are growing truly immense. For AI and machine learning to continue to accelerate insight, compute and storage must not be limited by geography or any single cloud technology. The good news is that through a multi-cloud approach, incredible scalability is already possible. The companies and research organizations that arm their scientists with multi-cloud capabilities are likely to be the first to unlock medical science’s most incredible discoveries of the future.