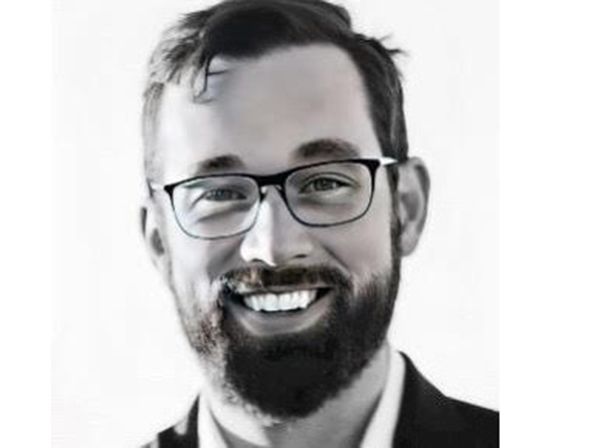
In an era where large language models (LLMs) are redefining AI digital interactions, the criticality of accurate, high-quality, and pertinent data labeling emerges as paramount. That means data labelers and the vendors overseeing them must seamlessly blend data quality with human expertise and ethical work practices. Crafting data repositories for LLMs requires diverse and domain-specific expertise. As such, this is an opportunity for data vendors to commit to building a solid team of experts and value the transfer of their knowledge throughout a data labeling project, as well as the people behind the data.
The future of AI-driven innovation will continue to be shaped by the individual contributors “behind” the technology. Therefore, we have a moral responsibility to promote ethical AI development practices, including our approach to data labeling.
Given this recent sea change and focus on LLMs, we have seen (at the very least) five critical trends that are the foundational pillars for the future of AI as we consider the human impact on emerging technologies.
1. Commitment to data excellence: The concept of data quality over quantity continues to be relevant in an age when data labeling requirements are about precision, protection, and practice. Data collection and annotation must be supported by top-tier anonymization processes with minimal bias. Bias minimization can only be achieved through comprehensive annotator training backed by regular audits and feedback cycles powered by the latest application systems to reinforce data integrity and reliability.
2. Fine-tuning and specialization for domain specificity: Every industry has specific language and labeling requirements and specializations, e.g., a medical diagnostic chatbot. Domain-specific fine-tuning aligns data annotation practices with the nuances of specific industries, such as health care, finance, or engineering. To be effective, machine learning models and analytics must be grounded in domain-relevant data in order to drive superior results with actionable insights.
3. Applying Reinforcement Learning with Human Feedback (RLHF): Human-in-the-loop feedback is essential to ensure the iterative evolution of machine learning models. The computational strengths of AI must be tempered by the qualitative judgment of human experts to create a dynamic learning mechanism that results in robust, refined, and resilient AI models. This dynamic learning mechanism merges the computational strengths of AI with the qualitative judgments of human experts, leading to robust, refined, and resilient AI models.
4. Respect for intellectual property and ethical data foundations: Respect for intellectual property is fundamental in the digital information age. As organizations continue to craft datasets for commercial contexts, it will be increasingly important to prioritize data authenticity and promote the highest ethical standards. AI models must be trained using genuine and ethically sourced data. This approach aligns technological advancements with moral responsibility.
5. Use of diverse annotation teams to promote global relevance: AI operates in a global marketplace where data annotation demands a global perspective. Data labeling requires a diverse pool of (human) annotators spanning different cultures, languages, and backgrounds, ensuring representation across varied linguistic, academic, and cultural backgrounds. Applying diversity to data labeling captures global nuances so AI systems are more universally competent and culturally sensitive.
Emerging AI data labeling practices mark a new convergence of technology and the human-in-the-loop approach. Therefore, it is important that today’s data scientists to champion data quality, ethical practices, and diversity while inviting stakeholders to join us in shaping an inclusive and innovative AI future.