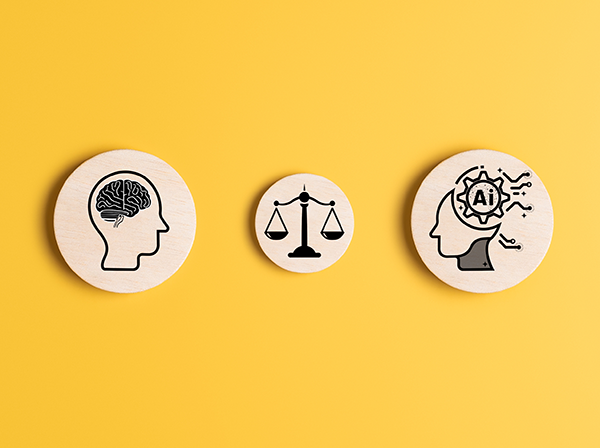
The AI revolution isn’t coming: It’s here, and organizational governance structures are woefully unprepared. While 64% of businesses expect AI to increase productivity, most AI models fail. This result highlights a stark gap between potential and reality.
Getting timely and usable data sets for AI training and analysis is crucial for becoming data-driven. At the heart of this multi-faceted challenge lies a fundamental question: How can organizations evolve their existing data governance (DG) programs, defined as the execution and enforcement of data policies, to meet AI’s unique demands?
To understand and address this dilemma, data governance experts Kelle O’Neal and Christine Haskell led an open discussion at a recent Data Governance & Information Quality (DGIQ) conference. Their session, “Contemplating Governance in the AI Era,” uncovered key insights.
This discussion identified breaking points and offered valuable insights. It highlighted how organizations can start to bridge this governance gap. This article explores these discussion points and offers ideas for adapting data governance strategies to harness AI’s power, while managing its risks.
Understanding AI Governance Constructs
To start off the session, Haskell and O’Neal ensured a clear understanding of AI and its governance constructs. Haskell described AI as “computer systems that can mimic human intelligence and cognitive abilities.”
Building on this definition, O’Neal fleshed out related ideas for the AI era. She outlined three key governance constructs:
- AI for Data Governance: AI for governance covers all the tools that improve the effectiveness of governance. O’Neal sees generative AI as a big breakthrough in this governance construct. Its capabilities mimic human cognitive behavior in different data activities and connect technical and business requirements. These functions free up humans to govern more productively.
- Governance of the AI Processes: This topic spans execution and enforcement around AI processes. The governance of AI processes includes “guidelines, guardrails, and discussions around content that goes into the AI models and algorithms.” This idea encompasses governance and ethical discussions around the use of what the AI produces.
- Governance of Different AI Components: AI comprises different elements, like models, code, learning style, and algorithms. Organizations need to manage these components in the AI Model Lifecycle, from creation, training, maintenance, and retirement stages. Governance covers all these various aspects.
Data governance underlies all three types of AI administration. AI is used in DG, ensures quality data for AI use, and guides decisions when managing different AI components. Identifying each of these governance perspectives helped frame the rest of their session.
Data Governance and the AI Model Lifecycle
O’Neal said, “Since AI models rely on data, data governance plays a significant role in governance in the AI era.” She illustrated this point through the AI Model Lifecycle (AML), the steps to get the AI output.

As the diagram shows, data governance is present at every stage of the AI lifecycle, adapting its role to meet the specific needs of each phase. This intertwining of data governance and AI processes demonstrates a path for evolving governance practices to better service AI models.
While the diagram outlines the theoretical framework of data governance in the AI Model Lifecycle, Haskell’s observations brought a practical dimension to the discussion. She emphasized that “Governance exists everywhere, even in organizations that reject formal governance structures.”
Then she proceeded to a real-world example: Amazon. Haskell stated:
“Amazon, known for its ‘customer first’ policy and anarchic approach, implements activities associated with governance. Different groups develop guidance, then disband and use these suggestions to solve customer problems. This process replicates data governance activities seen in more formal settings.”
Amazon’s flexible approach to governing data, by developing guidance and then applying it to solve customer problems, mirrors the iterative nature of AI development and deployment. It showcases how existing governance practices can adapt to suit different organizational cultures and technological needs.
Transforming Data Governance for AI
While AI governance incorporates existing data governance principles, it must adapt to meet the specific challenges posed by AI models, such as handling unstructured data and addressing ethical concerns. O’Neal proposed a framework for evolving existing data governance to meet AI governance needs, as illustrated in the following diagram.

The diagram, a continuum, illustrates that both data and AI governance policies share common focal points. Moreover, AI governance needs are greater because they include all aspects from the existing data governance and extend the foci.
- Guiding Principles: Guiding principles form the foundation of data and AI governance. O’Neal said, “We are all accountable for the data we create and use. As we move to AI governance, we need to consider ethics.”
- Op Model Organization: This focal point considers participation and roles across the enterprise. She noted: “Since AI is embedded in applications, used across an organization’s business processes, the operating model needs to expand. Constituents that have not needed to participate in data governance may need to be involved in AI governance.”
- Governance Subject: Data governance covers critical data elements (CDEs) created internally. O’Neal advised that we need to extend AI governance to administer more components, data sets, and AI models. Moreover, these elements can originate internally or externally to the organization.
- Typical Data Types: Most organizations already govern structured data. Companies need to expand data governance to cover semi- and unstructured data to meet AI’s training and analysis requirements.
While this framework provides a good starting place, evolving data governance to meet AI needs promises to become complex. For instance, current regulations hold companies accountable for protecting personal data as part of data governance and AI governance. Now, AI poses ethical concerns such as potentially biased outputs and the handling of sensitive data.
Consequently, AI governance must discover ways to address potential non-objective outputs and private information. This approach may involve giving guidance and assigning roles to determine what information can go into AI and recognizing its questionable responses. As organizations navigate a wide range of AI governance complexities, they must remain adaptive and continuously improve their strategies to keep pace with AI advancements.
Data Quality for AI Readiness
While evolving existing data governance to meet AI needs is crucial, many organizations need to advance their DG first, before delving into AI governance. Existing data quality does not cover AI requirements.
As mentioned in the previous section, currently DG programs enforce roles, procedures and tools for some structured data throughout the company. Yet AI models learn from and use very large data sets, containing structured and unstructured data. All this data needs to be of good quality too, so that the AI model can respond accurately, completely, consistently, and relevantly.
Companies frequently struggle to determine if their unstructured data, including videos and PowerPoint slides, is of sufficient quality for AI training and implementation. If organizations don’t address this issue, Haskell said, they “throw dollars at AI and AI tools,” because the bad data quality inputted gets outputted.
For this reason, the pressures of data quality fundamentals and clean-up take higher importance over the drive to implement AI. O’Neal likened AI and its governance to an iceberg. The CEO and senior management see only the tip, visible with all of AI’s promise and reward. However, beneath the surface lies the vast majority of the iceberg, e.g., all the data that no one has bothered to understand or its lineage.
In the meantime, without adequate data quality as governed by data governance, the C-suite steers the corporation into the iceberg, which is an expensive accident. Consequently, evaluating the existing data governance program and applying data quality best practices may give organizations the best chance for AI readiness.
The Future of AI Governance: Key Takeaways and Next Steps
As AI continues to reshape the business landscape, organizations must adapt their governance strategies to stay ahead. O’Neal and Haskell offered critical insights for navigating this AI era:
- Strengthen existing data governance practices with a strong data quality foundation for AI models.
- Leverage existing data governance best-practices as a foundation for developing effective and productive AI models.
- Expand what works in DG to cover new areas specific to AI, including ethics, broader data types, and AI components.
- Foster collaboration across the organization to develop governance strategies that align with both business requirements and cultural implications of AI adoption.
- Consider why an AI tool is needed, the cultural implications, and necessary administrative transitions.
Foremost, companies need to evaluate existing DG structures to see what is available and how to grow them. Corporations need to foster a culture of continuous learning and adaptation.
While these takeaways provide a solid starting point, the rapidly evolving nature of AI governance demands ongoing learning and adaptation. Attending conferences and trainings offers opportunities. Professionals can dive deeper into these topics, learn from industry experts, and gain practical insights and strategies for implementation.
Want to learn more about DATAVERSITY’s upcoming events? Check out our current lineup of online and face-to-face conferences here.

Here is the video of the DGIQ presentation: