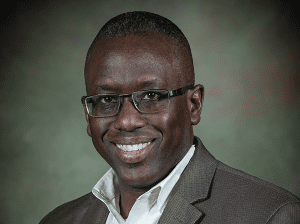
In today’s rapidly evolving business landscape, the role of quality management (QM) is undergoing a significant transformation. No longer just a compliance checkbox, QM is emerging as a strategic asset that can drive continuous improvement and operational excellence. This shift is largely propelled by the adoption of intelligent technologies and the strategic use of data, enabling organizations to gain comprehensive insights into their quality performance and take proactive measures.
In this new paradigm, success hinges on an organization’s ability to efficiently collect and utilize intelligence to analyze data. As our understanding of quality evolves, access to high-quality data becomes a primary indicator of technological advancement. Companies that view quality as a vital tool for continuous improvement and embrace automation are better positioned for greater success.
Current Uses and Implementation Challenges
Organizations are increasingly leveraging automation to enhance their quality management processes, particularly in event management. This broad category includes various incidents such as customer complaint calls, non-conformances, and other high-volume data events that are typically labor-intensive and prone to human error.
Automating the event management process has become crucial. It enables organizations to streamline the capture, triage and routing of cases and non-conformances to appropriate channels, extending to tasks like proper coding. Moreover, companies are exploring how artificial intelligence (AI) can facilitate more efficient and accurate investigations without duplicating previous efforts.
Beyond event management, organizations are seeking a more holistic view of their reporting and understanding of the entire value chain. Rather than relying solely on point solutions from vendors like SAP or Microsoft, there’s a growing trend toward centralized data warehouses or data lakes. This approach allows companies to consolidate data from disparate systems, providing insights into inefficiencies, areas for improvement, and overall operational performance. This can be of particular advantage when working with companies that have a range of differing data sets, data structures, and data sources across their existing systems, for example in companies that have grown through mergers and acquisitions and that have left legacy systems in place, or companies that have a range of different deployment and configurations of the same technological solution (i.e., multiple ERP or PLM systems).
However, as automation and AI become more prevalent in QM processes, organizations face the challenge of determining the right balance between automated and human-driven activities. While most quality professionals agree that automation and AI can’t entirely replace human involvement, there may be specific processes where manual work can be minimized. This human-in-the-loop approach is an ongoing industry dialogue, ensuring necessary checks and balances through human oversight.
Defining High-Quality Data for QM
In the context of quality management, defining high-quality data goes beyond typical considerations like accuracy. The primary factor should be purpose-driven data collection. Organizations often amass vast amounts of data without a clear understanding of what they aim to achieve or improve through data analysis. Establishing a well-defined purpose upfront for the high-quality data being collected is crucial.
Once the purpose is established, data accuracy becomes a critical factor, especially when integrating data from disparate systems into a centralized data warehouse. A robust data cleansing strategy is essential to prevent the “garbage in, garbage out” syndrome that can undermine analytics efforts. Addressing data bias is also key; for instance, can you identify areas of potential bias in your data sets? How can data bias be reduced? What subsets of data are overrepresented or underrepresented?
The reliability of data sources is another vital aspect. In regulated industries like life sciences, validated solutions with rigorous testing protocols are required for systems like electronic quality management software (eQMS). However, not all sources maintain this level of rigor. Organizations must understand this footprint of validated and unvalidated solutions to implement appropriate data governance plans.
The importance of high-quality, accurate data becomes even more critical when training AI models. Misinformation or hallucinations stemming from poor data sources can severely hamper their performance. One of the biggest challenges with AI adoption is ensuring access to the right datasets in sufficient volume and quality.
Fostering a Forward-Thinking, Customer-First Quality Culture
Traditionally, quality management has been seen through a compliance lens – a necessary business cost to meet regulations. To unleash QM’s power as a catalyst for ongoing business growth and customer satisfaction, a fundamental mindset shift toward a more comprehensive, proactive approach is crucial. In the past, quality reporting and data tracking were reactive, addressing issues after they occurred. This fuels a fix-it-later cycle instead of prevention. The needed cultural change is from reactive to proactive QM.
Forward-thinking firms use AI and predictive analytics to foresee problems before they arise, emphasizing prevention and continuous improvement. However, some companies remain regulation-focused due to deep-rooted challenges. Breaking this mold requires realigning toward customer-centricity, building robust systems that prioritize satisfaction and ongoing enhancement, with regulatory compliance as a natural outcome. It’s key to see quality and regulatory goals as aligned to each other and drivers of commercial growth, not conflicting with each other and inhibitors to commercial growth.
The ultimate organizational change is abandoning the outdated cost-avoidance mindset that sees compliance efforts merely as “trouble prevention” with the target to “meet minimum requirements.” This defensive stance stifles quality’s potential to drive productivity, efficiency, and continuous improvement. Success in this new era will depend on a company’s ability to nurture a proactive, customer-driven quality culture that relentlessly pursues operational excellence beyond basic compliance. Improved product quality drives improved customer engagement, which is a positive when it comes to commercial performance.
Quality Management’s Automation Triumphs
Companies are revolutionizing QM through automation, with event management leading the charge, delivering dramatically improved by automating parts of their complaint handling. Efficiency-wise, automating complaint intake, triage, and routing has cut manual work and human errors. This is particularly valuable given the high volume of incidents to manage. The result? Significant cost savings in these labor-heavy areas. By smartly applying an automated, data-driven QM approach where it fits, while maintaining crucial human oversight, many enterprises have seen productivity soar. The drive to more efficient, effective, and consistent workflows yields benefits for customer focus, regulator relationship and engagement from company quality and regulatory professionals.
While event management has been the initial focus, the entire eQMS landscape offers vast automation potential. Currently, areas with the most pressing issues are drawing the most automation efforts. But as successes mount, expect automation to spread across more QM domains over time.
Quality Management’s Future: AI, ML, and LLMs Lead the Way
The tech landscape’s evolution, featuring AI, machine learning (ML), and advanced large language models, holds great potential for QM’s future and data-driven choices. A steady rise in these emerging technologies’ adoption is seen across sectors, from big enterprises to smaller firms. A trend toward holistic data analytics, including data warehousing and real-time analysis, is growing. AI and ML are set to be key in this shift, though they may lag behind wider business adoption due to QM’s inherent caution, especially around regulatory compliance.
The FDA and EMA are expected to issue AI and ML guidelines for QM, shaping their adoption. Balancing efficiency with regulatory compliance is a complex challenge, particularly in highly regulated fields like life sciences. Moving to a predictive model, tackling issues before they grow, is the next goal. Yet, this shift will likely be gradual, as quality experts consider impacts on established practices and patient safety. Step-by-step changes and pilot programs offer a smart way to blend AI and ML into QM, enabling thorough testing without risking operational stability or compliance.
Seamless Quality Management Integration: Unlocking Business Insights
Merging QM data with other enterprise sources is now critical. Data warehouses and lakes act as bridges, connecting isolated QM systems with the broader business landscape. An eQMS with robust tools that unify various data sources enables richer reporting, driving significant business enhancements.
These data hubs enable two-way information flow, vital for a comprehensive QM approach. This interconnected system affects not just quality but also key areas like manufacturing. By analyzing data holistically, beyond quality alone, companies can uncover wider process improvements, boosting automation, analytics, and AI capabilities.
For firms embarking on this integration, the first step is assessing current systems. This means understanding existing setups, reporting tools, data governance, and cleansing issues. Next, develop a thorough reporting and analytics plan, involving data scientists skilled in managing data models and integration. Equally important are training teams and setting clear, measurable success metrics.
Breaking Down Barriers to Data-Driven Quality Management
Traditionally, quality has been seen as a cost, not a profit driver. Shifting this view requires showcasing how QM boosts efficiency and business performance. When firms integrate QM with CRM and ERP systems, they quantify its benefits, fostering a more comprehensive perspective.
This holistic view is key, examining QM at system, process, and data levels. Seeing quality as core to business operations underscores its interdependence with other functions, supporting overall success.
Many companies generate extensive reports but struggle to get the right data to the right people for informed decisions. The focus should be on using data to drive change, tailoring information to each stakeholder’s needs.
QM’s risk-averse culture is another hurdle. While regulatory concerns are valid, embracing AI, machine learning and other advanced tech is crucial. Companies that adopt these tools will thrive; those resisting change will lag behind.
Sharing success stories and pilot projects builds confidence. Demonstrating new approaches’ tangible benefits gains buy-in across all levels. In today’s competitive life sciences sector, quality can be a true differentiator by ensuring treatment efficacy.
As QM evolves, organizations integrating data and emerging tech will lead. Overcoming data-centric barriers means changing perceptions, leveraging AI and machine learning, and fostering continuous improvement. This shift unlocks operational excellence and customer satisfaction, making QM a standout feature in modern business. Ultimately, this evolution in QM will enhance the key imperative – the provision of safe and effective global healthcare solutions.