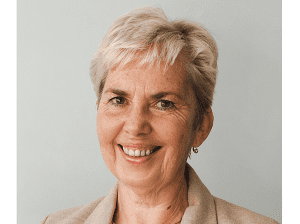
In this series of blog posts, I aim to share some key takeaways from the DGIQ + AIGov Conference 2024 held by DATAVERSITY. These takeaways include my overall professional impressions and a high-level review of the most prominent topics discussed in the conference’s core subject areas: data governance, data quality, and AI governance.
In the first blog post of the series, I shared my observations and described trending topics in data governance. This article will focus on data quality.
Please note that this review provides a general perspective and does not reference specific presentations from the event.
Let me again to start with my impressions regarding the presentations related to data quality.
General Observations
Changing Focus from Common Data Quality to Data Quality for AI
The shift from traditional data quality to AI-specific data quality reflects the unique demands of AI systems, such as mitigating bias, ensuring data relevance, and maintaining consistency across training and future datasets. Unlike general data quality, which prioritizes data completeness and accuracy, AI requires context-aware and domain-specific approaches tailored to each model’s goals. Some presentations highlighted the need for cultural shifts in organizations where AI readiness and data quality standards are tightly integrated. This transition underscores the high stakes, as poor-quality data can severely compromise AI’s reliability and effectiveness.
Focus on Implementing the Concept “DQ by Design” in Various Platforms
“Data Quality by Design” integrates quality assurance into platforms’ architectures, emphasizing prevention over reactive fixes. Platforms like Validatar and Qualytics illustrate this through features like automated rule enforcement, machine learning-based monitoring, and metadata-driven workflows. These innovations ensure data integrity across diverse systems and empower users to address issues collaboratively. The proactive nature of DQ by Design aligns quality management with scalability, making it a cornerstone of efficient data operations in modern enterprises.
Fewer Data Quality Presentations Compared to Data Governance Presentations
As discussed in the previous article, data quality is often regarded as a subset of data governance, leading to fewer dedicated discussions on its unique challenges. While governance addresses broader frameworks for data management, data quality focuses on the operational aspects critical to decision-making and AI readiness. The limited focus on data quality highlights a need to differentiate it more clearly from governance and emphasize its foundational role in ensuring organizational success.
Trending Topics
Specifics of Data Quality for AI
Data quality (DQ) is fundamental to the success of AI systems, as the accuracy and fairness of AI outputs directly depend on the integrity of the data used. Unlike traditional data applications, AI requires accurate, consistent, contextually relevant, and unbiased data. These unique demands necessitate a focused approach to data quality, including the consideration of the following (see Figure 1):

Challenges of Data Quality for AI
AI presents unique challenges for data quality management. Ensuring that training data aligns with future operational data is critical to prevent model drift, which occurs when discrepancies lead to declining model performance. Bias in training datasets is another significant concern, as it can amplify inequities and skew results.
Additionally, AI applications demand comprehensive and relevant domain-specific datasets, requiring rigorous quality checks and tailored governance frameworks. The emergence of large language models (LLMs) and generative AI (GenAI) further intensifies these challenges, requiring data to be curated for semantic relevance rather than solely syntactic standards. AI’s dependency on contextual and structured data makes detecting hidden inaccuracies and filling gaps even more essential.
Problems with Data
Common data issues include inconsistent formats, missing values, and duplication, which can compromise the reliability of AI systems. Fragmented storage and a lack of alignment between data collection and usage often exacerbate these problems, leading to inefficiencies and errors. Semantic challenges such as misaligned data relevance and undetected bias further complicate the task, especially in scenarios requiring the integration of diverse and unstructured datasets. Unchecked issues like these increase the risk of erroneous predictions and undermine AI-driven decision-making.
The Impact of AI on Data Quality
AI is transforming the data quality management landscape, introducing opportunities and new challenges. While core DQ dimensions like accuracy and consistency remain relevant, semantic dimensions such as relevance, objectivity, and believability are increasingly important. AI tools, including LLMs, have enhanced the ability to uncover hidden patterns and automate tasks like data classification and validation rule generation. These tools also bring challenges, including the risk of training on poor-quality data, which can perpetuate inaccuracies in future AI outputs. Moreover, AI has shifted focus to neglected DQ dimensions and expanded collaboration across data teams, emphasizing the need for engagement with business units, IT security, and product management teams.
Specific Data Quality Requirements for AI
AI systems require high-quality training data that is comprehensive, timely, and free from bias. Clear definitions of data elements, such as units of measurement and terminology, are essential to ensure interoperability and consistency. Semantic relevance is becoming a key requirement, as irrelevant or redundant data clutters AI models, reducing efficiency. Bias detection and mitigation strategies are equally critical, requiring sophisticated statistical methods and proactive measures to ensure objectivity and fairness. Integrating privacy safeguards, especially during training with sensitive datasets, is another essential requirement of modern DQ frameworks.
Setting Up Proper DQ Management for AI
Effective data quality management for AI involves defining clear quality criteria, regularly assessing both training and operational datasets, and leveraging automation for scalability. Emerging AI-driven tools enhance this process by automating tasks like validation rule generation, data cleaning, and duplicate detection. Collaboration across business, IT, and analytics teams is vital for aligning DQ efforts with organizational goals. As the use of LLMs grows, organizations must focus on the accuracy of training data to avoid cascading errors in AI outputs. Regular audits and engagement with domain experts can bridge the gap between validation and factual accuracy, fostering trust and delivering impactful results.
Transforming Failures into Success Factors and Lessons Learned
Common Pitfalls and Their Impact
There are several common success factors for data quality programs, as shown in Figure 2.

Large-scale projects, such as ERP implementations, often fail due to poor data quality, leading to missed deadlines, budget overruns, and limited utilization of new system capabilities. Contributing factors include weak stakeholder engagement, inadequate data governance, and failure to address the root causes of data defects. These shortcomings can derail even the most well-intentioned initiatives, resulting in inefficiencies and organizational frustration.
Turning Challenges into Success Factors
Proactive approaches can transform these failures into critical success factors. Figure 3 demonstrates some examples.

Early identification and resolution of data issues through iterative testing cycles are essential to refine accuracy and mitigate risks. Fostering collaboration between technical and business teams ensures shared ownership of data quality, aligning project goals across all stakeholders. Embedding data quality practices into every project lifecycle phase enables organizations to meet deadlines, control costs, and achieve operational success.
Lessons from Experience
Several common lessons learned are shared across the presentations, shown in Figure 4.

Experience from real-world projects highlights the value of starting with small, manageable use cases to build momentum and demonstrate success. Iterative approaches, such as continuous testing and monitoring, help to uncover and resolve data defects early, minimizing disruptions. Teams that embrace challenges as opportunities for growth and adapt strategies based on real-time feedback achieve better results. Furthermore, viewing data quality as an organizational priority rather than just a technical objective fosters a culture of accountability and drives long-term reliability in operations and decision-making.
By addressing these common pitfalls and applying these lessons, organizations can transform data quality challenges into opportunities for sustainable success, ensuring projects are delivered on time, within budget, and with maximum impact.
Approaches to Measuring Data Quality
Measuring data quality is a multifaceted process requiring a combination of strategies to ensure datasets meet organizational and project-specific standards. Organizations must adopt diverse approaches to address technical accuracy and user confidence in data.
Objective Measurements
Objective methods focus on quantifiable metrics such as completeness, accuracy, consistency, and timeliness. These involve automated tools and predefined rules to assess datasets against established benchmarks. For example, automated checks can identify missing values, duplicates, or format inconsistencies. Advanced monitoring frameworks evaluate data quality in real-time, providing immediate feedback on deviations. These methods are particularly effective in high-stakes environments like AI and ERP systems, where scalability and reliability are crucial.
Subjective Measurements
Subjective measurements focus on stakeholder perceptions and their confidence in the data. Surveys, interviews, and collaborative workshops gather feedback on how data quality impacts decision-making and operational workflows. These insights often reveal usability concerns or trust issues that may not surface through objective assessments alone. For instance, stakeholders may highlight inconsistencies across systems that erode confidence in data reliability, prompting further investigation and alignment of governance processes.
Holistic Data Quality Assessments
Combining objective and subjective methods creates a comprehensive data quality assessment. Iterative testing cycles track defect resolution over time, while stakeholder feedback captures qualitative improvements in trust and usability. This dual approach identifies technical issues and aligns data quality initiatives with organizational goals, ensuring long-term success.
The next blog post in this series will discuss the trending topics in AI governance and management.