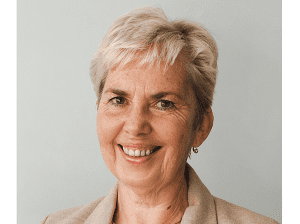
I had the opportunity to conduct a workshop at DATAVERSITY’s DGIQ + AIGov Conference 2024. Events like this provide a chance to stay updated on the latest trends in the global data management field, exchange ideas, and learn directly from experienced professionals in an in-person setting.
In this series of blog posts, I aim to share some key takeaways from the event: my overall professional impressions and a high-level review of the most prominent topics discussed in the conference’s core subject areas: data governance, data quality, and AI governance. Please note that this review provides a general perspective and does not reference specific presentations from the event.
I want to express my gratitude to many data management experts who generously shared their knowledge, developments, and experiences in data governance. This article shares the general observations and trending topics in data governance.
General Observations
Shifting Focus from IT-Centric to People-Centric Governance
Data governance is transitioning from a primarily IT-driven function to one that places people at its center, emphasizing collaboration, accountability, and cultural change. This shift reflects the understanding that successful governance requires stakeholder buy-in, clear roles, and active participation across the organization. Empowering data stewards, fostering data literacy, and integrating governance into everyday business processes have become central themes in building sustainable governance practices.
Advancing Data Governance Across Industries
Many organizations have made substantial strides in implementing data governance, tailoring their approaches to specific industry needs and challenges. The shared case studies highlighted the diversity in governance maturity, ranging from foundational initiatives to advanced frameworks. These examples also provided actionable templates, practical frameworks, and success stories that professionals can adapt to their organizations, offering a roadmap for scalability and sustainability. This wealth of knowledge reflects the growing recognition of data governance as a critical enabler of business success.
Divergent Viewpoints on Data Governance and Data Management
Data management professionals often hold varied perspectives on defining and differentiating data governance from data management, leading to diverse interpretations of their roles and objectives. This diversity spans two extremes.
On one end, some professionals adhere to the definition provided by DAMA-DMBoK2, which states that data governance involves exercising authority, control, and shared decision-making over the management of data assets. The data governance deliverables in this framework include a data management operating model, organizational structure, roles, and accountabilities. Data governance establishes the strategic vision, defining what data management should do and identifying the capabilities required to achieve business goals. Data management, in turn, develops, implements, and oversees the activities necessary to execute this strategic vision. Within the DAMA-DMBoK2 context, data governance is viewed as one capability among others, such as enterprise architecture, security, quality, etc.
On the other hand, some professionals have replaced the DAMA-DMBoK2 definition of data management with data governance, encompassing all related capabilities under the data governance umbrella.
I do not aim to judge which perspective is right or wrong – it ultimately comes down to terminology and definitions. However, this trend of multiple personal interpretations of data governance has created a “Babylonian” language in our community. I recommend my latest series of articles for those interested in exploring this topic further.
Emerging Capabilities in Data Management
The field of data governance is expanding to include new capabilities that address emerging needs and evolving organizational priorities. Data literacy has become a vital focus, emphasizing the need for employees to not only access but also understand and utilize data effectively in their roles. The rise of data storytelling reflects the growing importance of communicating data insights in ways that drive engagement and decision-making, transforming how governance initiatives are adopted across organizations. Furthermore, with the increasing complexity of data ecosystems, capabilities like data product governance have emerged, enabling organizations to manage and standardize reusable data assets tailored for specific business use cases. These innovations represent a shift toward empowering people and aligning governance practices with dynamic business and technological landscapes.
Trending Topics
Challenges with Implementing Data Governance
Challenges mentioned in multiple presentations can be categorized as follows (shown in Figure 1):
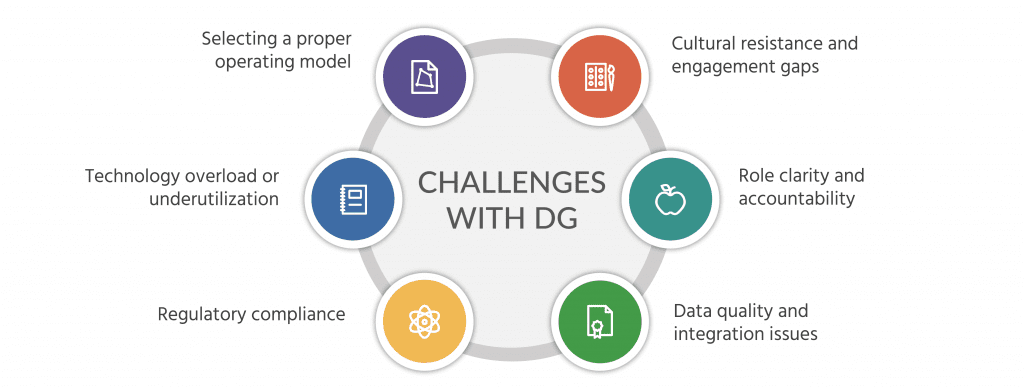
Cultural Resistance and Engagement Gaps
Organizations often face resistance to governance initiatives due to competing priorities, lack of trust, or insufficient understanding of governance value. Employees may perceive governance as restrictive or burdensome, leading to low participation and adoption rates. Building a data-driven culture and ensuring stakeholder buy-in remain critical challenges.
Role Clarity and Accountability
Defining clear roles and responsibilities for data stewards, owners, and governance teams is a persistent issue. Ambiguity in role definitions often leads to gaps in accountability, making it harder to consistently enforce governance policies and standards across the organization.
Data Quality and Integration Issues
Many organizations struggle with poor data quality, siloed data sources, and inconsistent standards. Ensuring clean, accurate, and integrated data remains a significant hurdle, particularly in large organizations with complex systems and legacy infrastructure.
Balancing Centralized and Decentralized Operating Models
Choosing the right governance model – centralized, decentralized, or federated – poses challenges as organizations attempt to balance uniform standards with domain-specific flexibility. Misalignment between centralized governance policies and localized operational needs can hinder effectiveness.
Technology Overload or Underutilization
While modern tools such as data catalogs and automation technologies are critical to governance, organizations often struggle to select and implement them effectively. Overreliance on technology without proper strategy or training can lead to underutilized solutions and failed initiatives.
Regulatory Compliance and Data Privacy
Evolving regulatory requirements, such as GDPR, HIPAA, and CPRA, pressure organizations to ensure compliance. Meeting these standards often involves overcoming challenges like implementing data security measures, monitoring compliance, and maintaining scalable audit trails.
Key Lessons Learned from Successful Data Governance Initiatives
The key lessons learned from data governance initiatives are summarized in Figure 2:
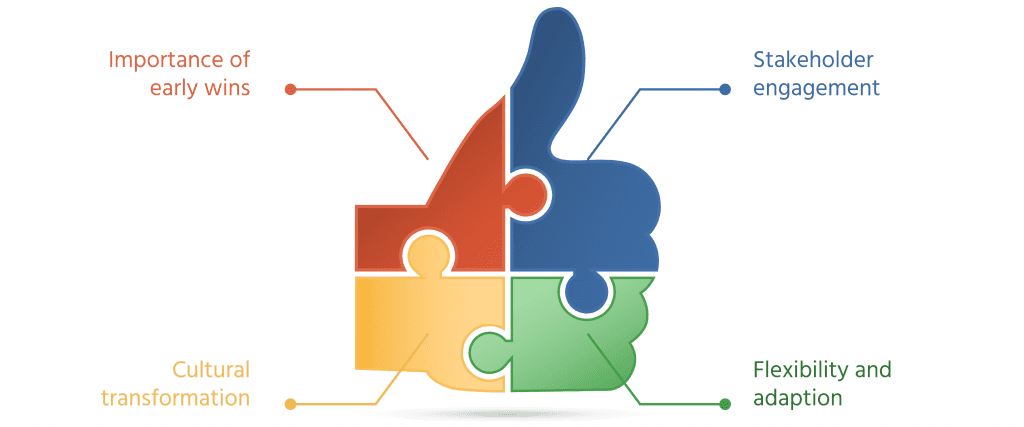
The Importance of Early Wins
Demonstrating quick, tangible successes early in a governance initiative builds momentum and secures stakeholder support. Pilot projects and small-scale implementations provide valuable insights, reduce resistance, and create a foundation for scaling governance efforts across the organization.
Stakeholder Engagement Is Key
Sustainable data governance relies on collaboration and buy-in from both technical and business stakeholders. Continuous communication, role clarity, and alignment with organizational goals ensure governance initiatives are well-integrated and supported at all levels.
Flexibility and Adaptation Drive Success
Organizations must tailor governance frameworks to their unique needs, balancing standardization with flexibility. Adjusting governance strategies based on feedback, evolving business needs, and emerging challenges fosters long-term relevance and scalability.
Cultural Transformation Is Essential
Creating a data-driven culture requires more than tools and policies – it demands sustained efforts to improve data literacy, promote accountability, and embed governance into everyday processes. Storytelling and clear communication about the value of governance can help overcome resistance and drive adoption.
Unified Steps to Implement Data Governance Programs or Business Cases
Several presentations highlighted the authors’ methodologies for successfully implementing data governance initiatives, programs, and use cases. The following is a summary of the common steps involved (see Figure 3):
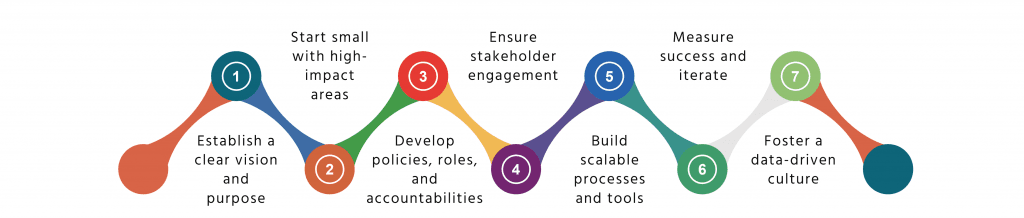
- Establish a Clear Vision and Purpose
An organization should define the strategic objectives of its data governance program and align them with business goals. A compelling narrative should be crafted to secure leadership support and stakeholder buy-in. This vision should be communicated clearly across departments to ensure alignment.
- Start Small with High-impact Areas
An organization should begin with key domains or critical data elements that align with strategic priorities. Pilot projects can demonstrate early successes and refine processes before scaling governance initiatives.
- Develop Policies, Roles, and Accountabilities
An organization should create policies and frameworks that clarify governance processes and establish accountability. Assigning roles such as data stewards and owners ensures clear responsibilities and consistent execution.
- Ensure Stakeholder Engagement and Collaboration
An organization should actively involve business and technical stakeholders to foster collaboration and trust. Regular communication and training should improve data literacy and ensure effective participation.
- Build Scalable Processes and Tools
An organization should design governance processes that are scalable and adaptable to growth. Automation tools should be leveraged to improve efficiency without compromising flexibility.
- Measure Success and Iterate
An organization should define KPIs to track governance progress and identify areas for improvement. Regular feedback and reviews allow for continuous refinement and adaptation of governance efforts.
- Foster a Data-Driven Culture
An organization should emphasize the importance of data as a strategic asset and integrate governance into its culture. Celebrating successes and showcasing results can drive adoption and long-term commitment.
Core Components of Governance Programs
Multiple presentations focused on the critical aspects of implementing data governance programs, sharing playbooks and approaches for developing strategies, defining roles, establishing operating models, crafting policies, designing processes, managing performance, and creating comprehensive roadmaps, as shown in Figure 4.
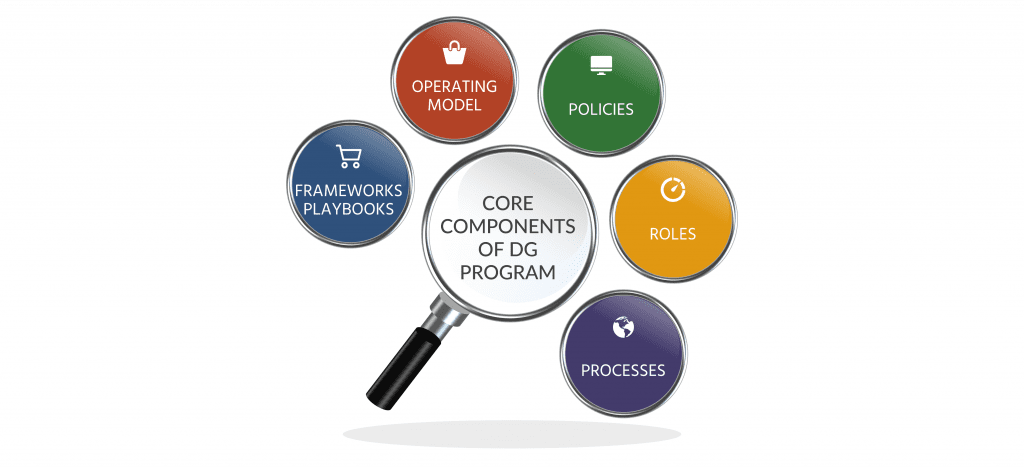
Frameworks and Playbooks
It was interesting to observe that, alongside references to industry guidelines such as DAMA-DMBoK2 and DCAM, many experts shared their own practical methodologies, frameworks, and playbooks for implementing data governance. They provided structured, actionable guides for implementing data governance programs, particularly for organizations starting with limited resources.
Operating Models
Governance operating models define the structure and approach to managing data governance, and numerous use cases demonstrated how different models are tailored to fit specific industry needs. From centralized to federated approaches, the presentations highlighted the importance of aligning the operating model with an organization’s size, complexity, and goals. These real-world examples showcased how adaptable operating models can drive successful governance across various sectors.
Policies
The presentations highlighted diverse approaches to developing data governance policies, reflecting organizations’ varying needs and priorities. Some emphasized top-down, centralized policy creation to ensure consistency and compliance across the enterprise, particularly in highly regulated industries. Others advocated for collaborative, domain-driven policy development, enabling flexibility and relevance to specific business units or functions.
Roles
The differences in how organizations define the scope of data governance versus data management significantly impact how roles are designed and implemented. Presentations highlighted varying approaches to assigning responsibilities to the roles of “data steward,” “data owner,” and “data custodian.” The omission of the “data user” role, which is vital as the end consumer of governed data, was notable across the discussions. These variations underscore how an organization’s interpretation of governance responsibilities influences the naming, scope, and hierarchy of roles, creating challenges in achieving clarity and alignment.
Processes
The scope of processes discussed in the presentation varied based on how organizations define data governance versus data management. Broader definitions tend to encompass operational activities such as data access and usage monitoring, metadata management, data quality, etc., while narrower interpretations focus primarily on policy enforcement and compliance. Integrating governance processes into daily business operations is key to ensuring they are effective and not treated as isolated tasks.
Use Case Studies
Data governance implementation varies widely across industries, with each sector tailoring its approach to address unique challenges and organizational needs. Below is a summary of key strategies and outcomes observed in different industries (Figure 5):
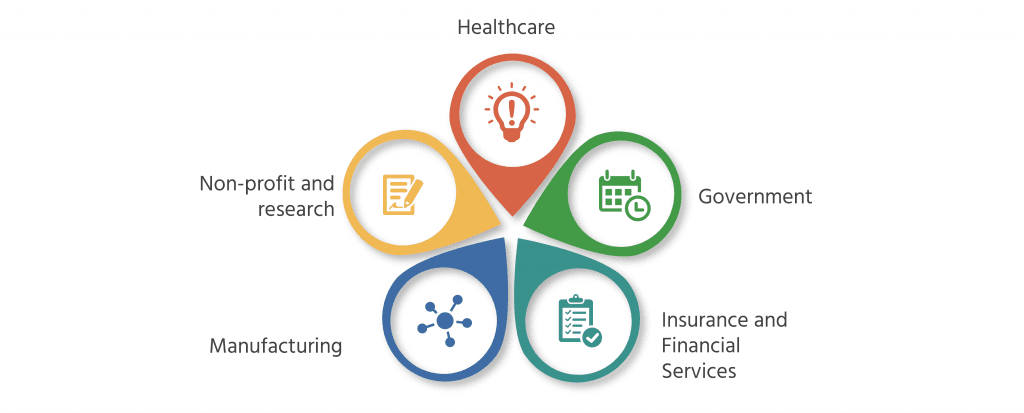
Healthcare
The healthcare sector highlighted challenges like data silos, inconsistent reporting, and a lack of transparency in analytics. Solutions included implementing data catalogs, aligning metrics with strategic goals, and fostering cross-departmental collaboration. These approaches led to improved data accessibility, better decision-making, and increased trust in analytics systems.
Government
Government case studies emphasized addressing fragmented data systems and meeting regulatory requirements. Initiatives included establishing governance councils, adopting federated models, and implementing stewardship programs to enhance data sharing and compliance. Successful implementations balanced central control with flexibility to cater to unique departmental needs.
Insurance and Financial Services
The insurance and financial sectors focused on compliance with privacy regulations and improving data quality for strategic decision-making. Efforts included automating metadata management, streamlining data workflows, and ensuring robust governance processes. These initiatives delivered time savings, enhanced operational efficiency, and ensured regulatory adherence.
Manufacturing
The manufacturing industry faced challenges like managing global data standards and integrating governance across diverse regions. Governance programs emphasized master data management, data quality monitoring, and stewardship practices to improve consistency and operational efficiency. These measures supported better decision-making and streamlined operations.
Nonprofit and Research
The nonprofit and research sectors demonstrated a shift from reactive to proactive governance models. Efforts included establishing centralized data policies, creating standardized datasets, and encouraging inter-departmental collaboration. These initiatives improved data reliability and supported broader organizational objectives, such as better engagement and operational alignment.
The following article will discuss the trending topics in data quality.
Want to learn more about DATAVERSITY’s upcoming events? Check out our current lineup of online and face-to-face conferences here.
