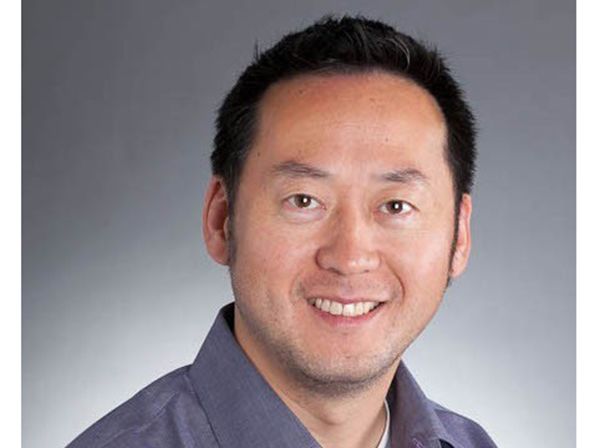
Today’s business landscape is more unpredictable than ever for IT leadership. Between the adoption of more sophisticated technologies and an ever-growing list of digital transformation initiatives, IT operations have become frustratingly complex. This is especially true when it comes to managing data flows and ensuring the precision of analytics insights.
At a time when analytics stands as a vital enabler of business strategy, the volatility, uncertainty, and ambiguity of modern data infrastructure management and engineering has extended time-to-insight, preventing organizations from operating efficiently.
In fact, MIT Sloan Management Review says that a leading view among Fortune 1000 executives is that faster time-to-insights is critical for business success. This view has transformed investments that improve the efficiency of data operations – and especially those that enable real-time analytics – into strategic priorities. But some organizations are still trying to figure out what real-time analytics means for them, or if real-time analytics is even possible for how they do business.
Two Types of Real-Time Analytics
Gartner defines real-time analytics as “the discipline that applies logic and mathematics to data to provide insights for making better decisions quickly.” Written more practically, real-time analytics is about using data to derive insights for decision-making as soon as that data is collected.
But “as soon as that data is collected” doesn’t always mean the same thing for every business. For some use cases, real time means that analytics is completed within a few seconds or a couple minutes after the arrival of new data. In other cases, “real-time” might mean sub-second, or even several minutes. Because “real-time” can be a matter of perspective, it’s important we focus this discussion on a general definition of the two types of real-time analytics we’re talking about when we talk about “real-time”:
- On-Demand: Users or systems wait to execute a query and to analyze the results.
- Continuous: Alerts users or triggers responses as events happen using predefined business rules.
The challenge for most organizations looking to deliver on-demand or continuous “real-time” analytics is in building an infrastructure capable of efficiently integrating data from all the various sources across IT into a single source.
This single source could be a data lake or data warehouse, with data models querying from this single source of truth to derive insights. Because of this, minimizing latency must be the goal if a real-time analytics experience is to be achieved. If not, the problems presented by legacy data systems will persist, or even worsen as infrastructure expands and becomes more complex.
In a hyper-competitive business atmosphere these delays can be costly, as a late decision is a bad decision. Even modest time deltas can make a big difference, especially when dealing with critical services and other time-sensitive business opportunities. As British naval historian Cyril Parkinson said, “Delay is the deadliest form of denial.”
Five Steps to Real-Time
Consider common use cases like credit card fraud prevention or personalized incentive marketing for e-commerce and social media; each depends on automating precise decisions in real-time. If an organization is not able to harness data quickly, the quality of the decision will either be sub-optimal – or irrelevant.
The key to achieving either on-demand or continuous real-time analytics lies in reducing the latency or the response times when bringing the data to the data warehouse and executing the query. Getting there requires five key components, each operating together in one virtuous cycle:
- Data Culture: The collective behaviors and beliefs of people who value, practice, and encourage the use of data for improved performance in business operations, compliance, and decision-making
- Data Literacy: The ability to understand and communicate data and insights.
- Data Quality: Data that is accurate, timely, and fit for use in operations, compliance, and decision-making
- Tools and Technology: Devices, systems applications, services, and other configuration items architected to store, move, and process data efficiently
- Data Governance: “The specification of decision rights and an accountability framework to ensure the appropriate behavior in the valuation, creation, consumption and control of data and analytics.” (Gartner)
Success for each step in this cycle is built upon an organization’s commitment to the previous steps. A healthy data culture gives way to better data literacy, improved data literacy leads to superior data quality, and a focus on data quality naturally drives organizations to invest in the right tools and technology to ensure that quality. Ultimately this system can only be maintained with good data governance, which incentivizes a healthy data culture to be sustainable.
Dependencies for Success
A failure to follow this model will almost certainly result in failure for an organization’s analytics ambitions, and it is the reason why Gartner predicted that only 20% of data analytics solutions will deliver satisfactory business outcomes for the companies that adopt them.
Similarly, other industry research has found that 87% of analytics projects never even make it to production. That is because, while there are many options for implementing analytics programs, success depends on creating a culture that encourages and supports “citizen analysts” and empowers them with the tools and knowledge to maximize their experience and outcomes.
As with any digital transformation effort, having and achieving an objective requires a vision for what success looks like and a roadmap for getting there. Making smart investments in people, processes, policies, and technologies – and especially with technologies capable of reducing friction and overcoming traditional barriers to data latency – are necessary for actualizing the ideal of operating a real-time analytics program.