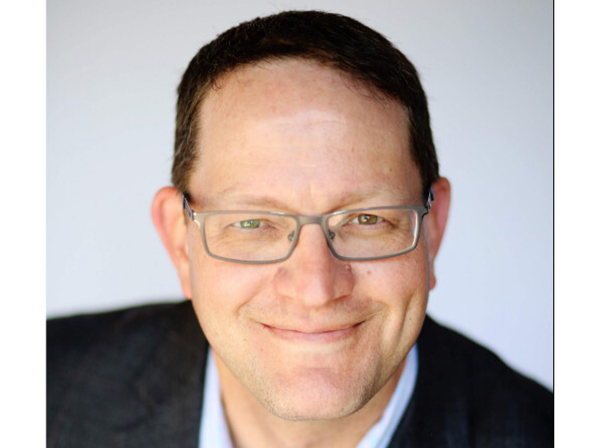
There’s no shortage of buzzwords and phrases to define how an organization approaches and uses its data – with two of the most popular being DataOps and data fabric. For years, large enterprises have struggled to distinguish between the two and decide which is the right first choice to enable them to compete with data and execute digital innovation. While both DataOps and data fabric can be the centerpiece of an organization’s Data Strategy – or used in tandem –they offer unique values and are two entirely different, but still related, approaches.
To determine which is the best for your organization to kick off your digital transformation, leaders must first decide which elements of their business and data infrastructure – from volumes and speed to data types and silos – can offer the best mix for data utilization and success. While the concepts are fairly new and are primarily used by organizations that are experiencing bottlenecks and barriers in delivering their current data and analytics services, these organizations need to truly understand and dig deeper into how they use data to its fullest potential before deploying a new approach.
Understanding Both the DataOps and Data Fabric Approach
As you consider what approach to initially adopt, the first step is breaking down the two to understand the true benefit of each or both:
DataOps: The concept of DataOps was born out of DevOps, which already exists somewhere in most organizations. Unlike DevOps, DataOps is all about taking common practices in modern software development and applying them to data projects. These can include concepts like continuous integration, source control management, unit testing, integration testing, and automated deployment.
Together, these allow data engineers and users to assemble data pipelines from end-to-end and centrally manage deployment. Typically, this goes hand in hand with an iterative agile approach as opposed to traditional waterfall models, which are still common in certain data projects.
When an organization leverages DataOps correctly they gain faster analysis to insight and greater overall agility. DataOps also allows data teams to innovate more quickly and have the confidence to make changes without worrying about affecting Data Quality or creating late delivery incidents – where the business doesn’t get the data they need on time. According to a recent study, a DataOps approach can reduce an organization’s total cost of data operations by 39%.
Data Fabric: At the most basic level, the role of a data fabric approach is to provide a better way to streamline and handle enterprise data. Gartner defines a data fabric as “an emerging data management design concept for attaining flexible, reusable and augmented data integration pipelines and services in support of various operational and analytics use cases delivered across multiple deployments and orchestration platforms.” A data fabric is able to support a combination of unique and different data integration styles in order to augment the data integration design and delivery process. While a data fabric is a relatively new concept, it’s ideally adopted by organizations that are heavily invested in modern technology and have mature data stacks, and are seeking increased flexibility. This approach is all about having metadata on all existing and new data coming into your ecosystem and using that metadata to take action. These actions are automated using semantics and graph technologies to streamline the end-to-end integration processes.
If an organization wants to get an end-to-end picture of the lifecycle of their data and centrally manage it, they need to be able to see all the information from across their data estate and more importantly automate the process. This is the benefit of a data fabric: It’s a unified view of an organization’s data and puts the data at its highest quality at the fingertips of analysts and data scientists with very little work from the data teams themselves. Data fabrics are essentially driven by the need for automation and speed of onboarding new datasets as well as the entire ecosystem working together to share metadata, knowledge about the data, and in a typical data stack, have all the tools involved have additive knowledge about the core data.
Where to Start: DataOps or Data Fabric?
Every organization is unique, so every Data Strategy is equally unique. There are benefits to both approaches that organizations can adopt, although starting with a DataOps approach is likely to show the largest benefit in the shortest amount of time. DataOps and data fabric both correlate to maturity.
It’s best to implement DataOps first if your enterprise has identified setbacks and roadblocks with data and analytics across the organization. DataOps can help streamline the manual processes or fragile integration points enterprises and data teams experience daily. If your organization’s data delivery process is slow to reach customers, then a more flexible, rapid, and reliable data delivery method may be necessary, signifying an organization may need to add on a data fabric approach. Adding elements of a data fabric is a sign that the organization has reached a high level of maturity in its data projects.
However, an organization should start with implementing a data fabric over DataOps if they have many different and unique integration styles, and more sources and needs than traditional Data Management can address. An organization that needs to build highly automated integration pipelines with minimal manual intervention can reap the benefits of a data fabric and the data fabric architecture includes the DataOps practices as well.
How DataOps and Data Fabric Can Work in Tandem
A DataOps approach is considered a needed layer to the overall data fabric, meaning once an enterprise has successfully implemented DataOps into their organization and set up a strong Data Governance practice they can easily build on a Data Fabric to advance their Data Strategy. The data fabric approach uses semantics and knowledge graphs to build augmented data integrations, which then DataOps processes are built from the output of lower-level pieces of the data fabric, allowing the two to work in tandem with one another. Once an organization has decided to go down the path of leveraging both approaches to maximize the use of its data, IT leaders should follow these steps.
- Instill the discipline of DataOps. It’s a discipline rather than a technology, and when you apply the technology without the discipline, you risk failure. So, start with a data platform to give yourself future growth potential.
- Make metadata available to everyone. Every tool in the business can benefit from it.
- Invest in best-of-breed tools and crucially integrate them using metadata. Get the basics in place, such as data ingestion, ETL, analytics, catalogs, and crucial end-to-end testing of the pipeline.
- Choose ingestion tools that maximize the capture of metadata at the entry point. Ensure the business doesn’t lose the context of the original source; where data originates from is often one of the most crucial pieces of metadata applied to a dataset.
Once these steps are working, you can build your fabric by adding tools to help with cataloging data, centralizing the sharing of metadata, reporting on lineage, governance, and handling of PII data. As you can see, DataOps is considered a needed layer to the overall data fabric approach, making these approaches stronger when implemented together.
Organizations should be asking the question, “Where do I start?” rather than “Which strategy should I implement?” in order to give their business users back the power they need to innovate with data and make decisions.