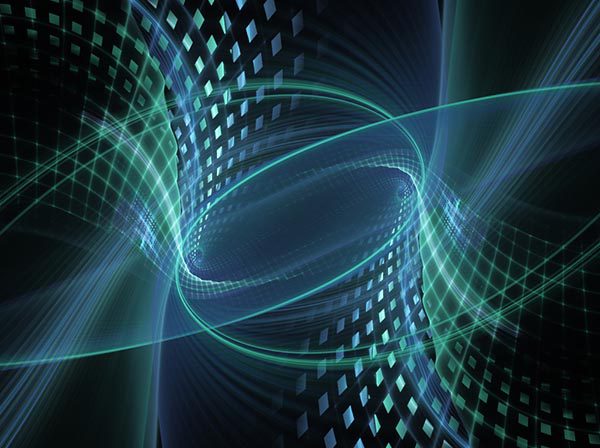
A recent press release reports, “Today Dataiku, the leading Enterprise AI and machine learning platform, announced the release of Dataiku 7, bringing deeper integration for technical data professionals to work on machine learning project development and row-level explainability for white-box AI. Additional feature highlights with this latest release include Kubernetes-powered web apps to expand on the capabilities introduced in Dataiku 6 and a machine learning-assisted data labeling plugin. ‘Collaboration has been at the core of Dataiku since our founding in 2013, and with Dataiku 7, we’re continuing to add features that deepen our philosophy to effectively democratize AI in the enterprise,’ said Dataiku CEO, Florian Douetteau. ‘With this launch, Dataiku 7 is our second consecutive product release that expands features for explainable AI, a critical component for organizations across industries to succeed and understand the impact of their AI model outcomes’.”
The release goes on, “Organizations worldwide are committed to enterprise AI efforts from the top down, but struggle to democratize projects from the bottom up to give more individuals access to actionable data insights. Dataiku 7 brings more people to the table via collaboration and empowers individuals with explainable AI for businesses to use data for day-to-day decisions and build impactful AI projects. With the launch of Dataiku 7, new features include: (1) Support for Advanced Statistical Analysis: Statisticians can now use Dataiku to perform advanced statistical analysis in the familiar worksheet-and-cards format while collaborating with the wider data or analytics team. In the past, advanced statisticians were relegated to siloed tools with no visibility for non-statisticians, creating bottlenecks in governance and AI project deployment. (2) Advanced Prediction Explanations: Traditionally, machine learning models do not include insights into why or how they arrived at an outcome, making it difficult to objectively explain the decisions made and actions taken based on these models.”
Read more at Business Wire.
Image used under license from Shutterstock.com