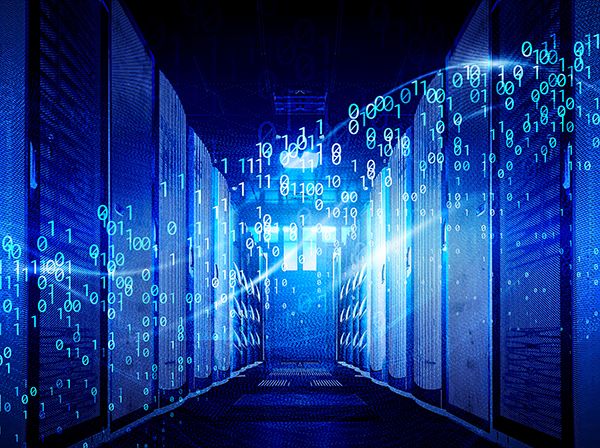
The phrase “data warehouse vs. data lakehouse” offers an exciting topic for ongoing debate in the global Data Management world. While businesses have relied on traditional data warehouses for storing structured and semi-structured data for years, the more recent technological solution of the data lakehouse is growing in importance because of its unique ability to provide structure to raw data.
Data warehouses and data lakehouses have emerged as two prominent adversaries in the data storage and analytics markets, each with advantages and disadvantages. The primary difference between these two data storage platforms is that while the data warehouse is capable of handling only structured and semi-structured data, the data lakehouse can store unlimited amounts of both structured and unstructured data – and without any limitations.
Data lakehouses meet the needs of organizations that require “more flexibility in their data platforms,” which is absent in traditional architectures of a data warehouse. Data lakehouses try to address the limitations of both the data warehouse and the data lake. The lakehouse offers a storage platform for both structured and unstructured data in one location while also accommodating BI, AI, and ML-driven analytics.
A common example of such a solution is Databrick’s open-source project, Delta Lake, which provides options for storage architecture that meet your organization’s needs.
Data Warehouse vs. Data Lakehouse: Relative Advantages
Traditional data warehouses have long supported all types of business professionals in their data storage and analytics endeavors. This approach involves ingesting structured data into a centralized repository, with a focus on warehouse integration and business intelligence reporting.
Enter the data lakehouse approach, which is vastly superior for deep-dive data analysis. The lakehouse has successfully blended characteristics of the data warehouse and the data lake to create a scalable and unrestricted solution. The key benefit of this approach is that it enables data scientists to quickly extract insights from raw data with advanced AI tools.
Data Warehouses: Pros
- The data warehouse enables collaborative decision-making by gathering, storing, and analyzing data from multiple data sets in one central location
- The data warehouse offers businesses the opportunity to centralize and analyze multi-source business data at one location
- Cloud relational databases provide scalable solutions for managing large amounts of data with ease
- Data warehouses are ideal for all types of transactional data, which is used for queries and reporting purposes
- Easier but powerful Data Management environment suitable for all business users
- Improved security
- Greater scalability
Data Lakehouses: Pros
- The data lakehouse offers low-cost storage over traditional cloud solutions
- Data lakes support both structured and raw data (unstructured) in native formats
- The data lakehouse allows storage of all types of raw or varied data in one place
- The various metadata storage options provide easy access for client applications
- Data lakes can store large amounts of raw data in real time from diverse ML and IoT devices at one location
- In the lakehouse, storage cost is mitigated by providing storage for large volumes of new data in real-time
- The lakehouse supports both traditional BI as well as more advanced analytics platforms like AI and ML
- The data lakehouse is ideal for supply chain analytics because of its instant predictive capabilities and tools
- Lakehouses allow organizations to fulfill cloud infrastructure needs and serve businesses that require the agility of diverse application development
Example of a Business Benefiting from Data Lakehouse
One example of how a company has benefited from migrating to a data lakehouse is Walgreens. When Walgreens migrated its system to Delta Lake, the company was able to improve its machine learning capabilities and perform with better accuracy by using visualizations to analyze its supply chain operations.
Data Warehouses vs. Data Lakehouses: Relative Disadvantages
Below, is a list of the downsides of using a data warehouse vs. lakehouse.
Data Warehouses: Cons
- Data warehouses usually require high setup and operational costs
- The data warehouse exists separately from operational systems, which results in more complex maintenance and deployment processes
- The context of the data can be lost when it is transferred to the warehouse, making it difficult for business decision makers to accurately analyze the information
- Data retention is a significant problem with the warehouse, as long-term storage of historical data costs more
- Potential issues with compatibility or integration of existing systems, but solutions like Azure Data Factory are available
- With its structured query approach, it is limited in deep data analysis
- During the ETL process, the data warehouse generally rejects some raw data that could be used for future analysis
Data Lakehouses: Cons
- The data lakehouse is designed for data scientists and not the average business professional
- Data lakehouse stores transformed structured data, which makes Data Management require more effort and resources to build a metadata layer
- SQL clients may be ineffective in a data lakehouse environment
- Traditional BI tools may struggle to find meaningful insights from the vast amounts of unorganized and disparate data within teams or web applications
Data Warehouses and Data Lakehouses: A Reality Check
Data warehouses have long been used for storing and managing vast amounts of data in a structured format. However, the primary disadvantage of using a data warehouse is that it can store only structured and semi-structured data, which can severely limit the types of data that can be included.
Although a data warehouse supports BI use cases and provides a “single source of truth” for analytics and reporting purposes, it can also become difficult to manage as new data sources emerge.
The data lakehouse has redefined how global businesses store and process data. Unlike traditional SQL databases or data lakes, data lakehouses allow users to store all forms of raw and structured from diverse data sources. This makes it easier for businesses to connect various types of information and use different approaches in processing data.
Additionally, with the use of additional analytical platforms, businesses can easily understand key differences between their datasets. The pros of using a data lakehouse are that it can handle a lot of work and store various types of data without the need for strict schema management.
Additionally, a data lakehouse acts as a single-view repository for all types of organizational data. This enables easy access to data, which is later used for quick decision-making.
Now the reality check on data lakehouses: BI and reporting tasks can become challenging without the presence of appropriate tools to support SQL queries. Users may frequently confront poor data quality and governance problems. The non-availability of data lakehouse case studies makes it difficult for potential business clients to assess this solution’s applicability.
Which is better – data warehouse or data lakehouse? In the end, it depends on your specific business capabilities and needs.
Image used under license from Shutterstock.com