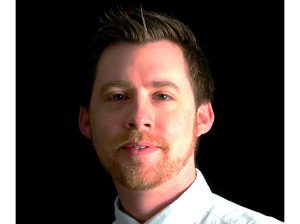
I have written before about the 5Ws of data and how important metadata – data about data – really is. This knowledge helps connect and contextualize data in ways that previously would take hours of knowledge and information mining. We have the tools now to automate this process and display it in a knowledge model of the data, to aid in the search and discovery of it. It becomes a digital model of your world – a contextualized model of your business or organization and the world it exists in.
This isn’t a new idea but it is one that not only is achievable with modern data platforms and data-focused tools but also helps with data literacy. Data literacy isn’t just understanding the data, it is how to operationalize this data in the modern enterprise – knowing that it’s not just the data and metadata but the data that resides inside of it and its place in your organization. It’s about democratizing it, making it discoverable and applicable to more. It’s about helping to solve challenges that come along with it: data bias, security, governance, compliance, risk identification, and mitigation. It goes far beyond the 1s and 0s that people have traditionally viewed data as.
Defining Data and AI Literacy
Data literacy equips employees at all levels with the skills to interpret, analyze, and leverage data in their decision-making processes. This not only enhances individual productivity but also fosters a culture of data-driven decision-making across the organization. When employees understand the data they work with, they can identify patterns, predict trends, and make informed decisions that align with the company’s strategic objectives. Moreover, data literacy helps in recognizing and mitigating potential risks, such as data bias or privacy issues, so that the data used is accurate, relevant, and ethically managed.
On the other hand, AI literacy empowers teams to effectively deploy and interact with AI tools, understanding both their capabilities and limitations. With AI literacy, employees are better equipped to integrate AI into their workflows, enhancing efficiency and innovation. They can critically assess AI-generated outputs, recognize when AI is appropriate for a task, and identify potential pitfalls, such as algorithmic bias or the risk of over-reliance on automated systems. AI literacy also involves understanding the ethical implications of AI, so that its use aligns with both legal standards and the company’s values.
Beware of Only Thinking AI
Now let’s look at AI and consider specifically GenAI and LLMs. These models are trained on vast amounts of data but rarely is this data in the state described above. This data is publicly available and found across the internet.
The backbone of the AI solution is the “intelligence.” I use this term in quotes as these systems are not yet or maybe never will be what we consider intelligent. But it is the model that you use when you prompt the GenAI and it is the model that analyzes your prompt and compares it to what it “knows” about the world and then generates a response.
Here is why taking an AI-first approach might fail you. You need to understand AI like your data, improving AI literacy in the process. The AI tools people are using, such as ChatGPT, Gemini, or Anthropic, have never seen your proprietary data, behind your firewalls, disconnected from the internet. So, the AI doesn’t really “understand” your world. As such, it might not return an answer. Or it may give you the wrong answer, “hallucinate” as it has become known or provide an answer where PII information is displayed. None of which helps with the prompt. So, someone, somewhere mentions that you could use retrieval augment generation (RAG) to improve these results. Or perhaps a vector search. Or both.
Use your proprietary data along with the prompt to improve the results, reduce hallucinations, increase accuracy, etc. But many organizations can now discover if you do this with a disconnected, disjointed data environment, where data is shoved to the GenAI as-is with no consideration for the data. Not only might this not solve the problem but now you’re presented with new problems. The GenAI or LLM is not “seeing” your proprietary data with the proper controls. It is feeding data that might ultimately not be important to the query. Or it is struggling to find the correct data that relates to the prompt.
AI and Data First
This is why you need to take an AI-and-data-first view when it comes to these solutions. Data is not only the foundation of AI, but it helps to create the scaffolding for it as well. It should provide the AI with the context for your organization. It should present a model of the world your organization exists in. Frame the prompt and the desired response with the data that is most important to the question being asked – all the while governing the data that the AI has access to and monitoring the response so that there is no data leakage or responses that contravene your internal controls and external regulations and standards.
When combined, these literacies create a synergistic effect. Data literacy ideally means that the data feeding AI systems is accurate, well-governed, and contextually relevant, forming a solid foundation for AI applications. Meanwhile, AI literacy allows employees to maximize the value derived from this data, using AI to uncover insights that might otherwise remain hidden. Together, they enable organizations to develop more robust, innovative solutions that drive growth and transformation.