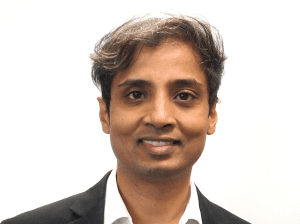
How will data visualization evolve in the era of AI/ML? While AI is rapidly evolving, it is ironic that business users are still using “dumb” dashboards. The challenge is to move beyond these unintelligent dashboards to a genuinely transformative visual analytics solution that harnesses the power of AI/ML. While some vendors offer a ChatGPT-like querying experience where users can query data using natural language, this approach does not realize the full potential of AI/ML. Simply querying data is not equivalent to analysis. Do humans still have to analyze on their own? Can an intelligent AI algorithm analyze instead and communicate the insights back to users? Any transformational analytics solution should be more than a mere ChatGPT querying experience. Before exploring potential solutions, it’s essential to understand the problem more thoroughly.
Any organization’s data visualization use cases can be classified into three distinct areas.
- Data Monitoring
- Data Analysis
- Storytelling with Data
The illustration below summarizes how data visualization tools currently solve data visualization use cases and the role of humans in the process.

As illustrated above, current visualization solutions still involve significant human effort. On the visualization creation side, humans must design static dashboards for monitoring, build interactive visualizations for analysis, and create visualizations with narratives to tell stories. On the visualization consumption side, humans need to monitor dashboards constantly and routinely interact with visualizations to analyze. Let’s look at how AI can completely transform the whole process, significantly reducing human effort while improving outcomes.
The illustration below summarizes how AI will solve data visualization problems in the future.

Evolution of Data Monitoring with AI/ML
How can data monitoring evolve in this era of AI/ML? I predict that the number of people who have to look at a dashboard to monitor data will slowly diminish over time. It is inefficient for humans to constantly monitor data on a dashboard to take corrective actions when AI can continuously monitor the data and alert users when human intervention is needed or take corrective actions without human interference.
Dashboards have inherent limitations in visualizing data for monitoring. Because of their limited real estate, a visual dashboard usually only allows for the visualization of five to six metrics, while the amount of data to be monitored rapidly increases. Relying on humans to look at static-layout dashboards to monitor data will become unfeasible in this age of big data.
A recent example of using AI/ML for monitoring is in IT operations. The system performance of IT apps, which was once visually monitored via dashboards, is now systematically monitored with intelligent data observability tools. These tools not only proactively detect anomalies but can also perform root-cause analysis.
Evolution of Data Analysis with AI/ML
“Analyst” is a widespread job title in many organizations. Large organizations have numerous data analysts who analyze data, develop insights, and communicate the insights to the right stakeholders.
Data analysis is primarily a manual process involving querying and interacting with data. After analysis, analysts must communicate their findings to business stakeholders by manually creating data stories that involve visualizations and narratives in PowerPoint or data visualization tools.
Human analysts will always have limitations, and some insights will likely be lost. Data mining techniques such as anomaly detection and pattern recognition have matured so much that a human doing manual analysis cannot compete with an “AI analyst.” An AI analyst will never tire and could potentially capture every relevant insight it has been trained to identify.
AI should not just be able to continuously analyze but also communicate that analysis to business stakeholders via data stories. The challenge with AI-generated data stories is that there will be some noise along with the signal. In the future, when analysts are augmented by AI-generated data stories, their role will involve vetting the data stories and curating the stories that require business stakeholders’ attention. With improved training of AI models over time, the noise will be reduced, eventually allowing AI-generated insights to be directly presented to business stakeholders.
Evolution of Data-Based Storytelling with AI/ML
The final frontier is whether AI can communicate these insights to business stakeholders by generating data stories with visualizations and narratives. The challenge is always the explainability of AI-generated insights.
As of today, analysts devote considerable time crafting stories with visualizations and narratives in PowerPoint. Some data visualization tools offer features that help create data stories manually within the visualization tools. Whether the data stories reside in PowerPoint or data visualization tools, the story-creation process is still manual and time-consuming. Storytelling will always be essential to influencing business stakeholders, but the question remains: Can AI automate the generation of data stories?
With progress in generative AI (GenAI), it’s possible to create complex visualizations from data [1] automatically. Also, NLG data-to-text is a prime use case of NLG [2] [3]. Looking ahead, I expect AI-generated analyses to be communicated through data stories consisting of GenAI-created visualizations and NLG-generated narratives. In the early stages of AI-driven analytics, human analysts must vet, refine, and curate data stories before they can be sent to business stakeholders. However, as AI models are trained better, the entire business intelligence workflow from monitoring, analysis, and storytelling can be automated, allowing humans to consume data stories and take appropriate actions.
Integrating Data Visualization and Automated Analysis
In my work at an investment bank, I could achieve analysis user experience (UX), where algorithmically done analysis is integrated with a scorecard-based visual analytics solution. So, the machine learning algorithm automatically does the analysis and alerts users. Users can click on the alert to see the underlying visualization and analyze it further. This paradigm of enabling analysis can be revolutionary for organizations as analysts do not have to analyze themselves; they can review the analysis done by the algorithm. While automatically generating a compelling data story is still a work in progress, I see a lot of promise in this direction with the rapid pace of AI/ML innovation.
References:
[1] Yilin Ye, Jianing Hao, Yihan Hou, Zhan Wang, Shishi Xiao, Yuyu Luo, Wei Zeng, Generative AI for visualization: State-of-the-art and future directions (2024), Visual Informatics, Volume 8, Issue 2, Pages 43–66, ISSN 2468–502X, doi.org/10.1016/j.visinf.2024.04.003.
[2] Y. Song, X. Huang, X. Zhao and R. C. -W. Wong, “Natural Language Generation Meets Data Visualization: Vis-to-Text and its Duality with Text-to-Vis,” 2023 IEEE International Conference on Data Mining (ICDM), Shanghai, China, 2023, pp. 1337–1342, hdl.handle.net/1783.1/135958.
[3] Islam, M. S., Laskar, M. T., Parvez, M. R., Hoque, E., & Joty, S. (2024). DataNarrative: Automated Data-Driven Storytelling with Visualizations and Texts, arxiv.org/abs/2408.05346.