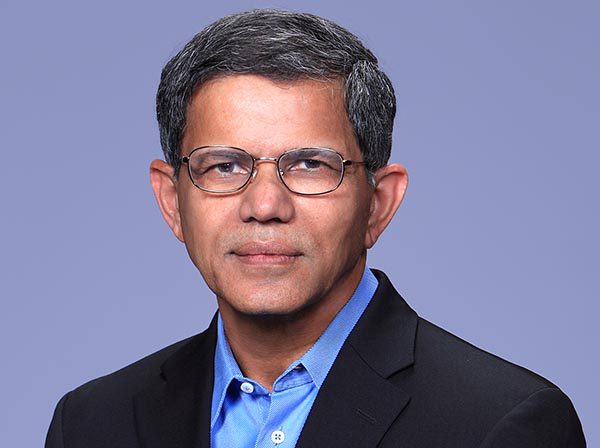
Click to learn more about author Ibrahim Surani.
Traditionally, companies have relied on the use of Extract, Transform, Load (ETL) solutions to gather data from disparate sources and populate a data warehouse. However, increasingly complex IT infrastructures of businesses and the need to acquire near real-time or real-time data for accurate decision-making have compelled businesses to look for new data integration solutions that involve less developer overhead and can accelerate the data pipeline.
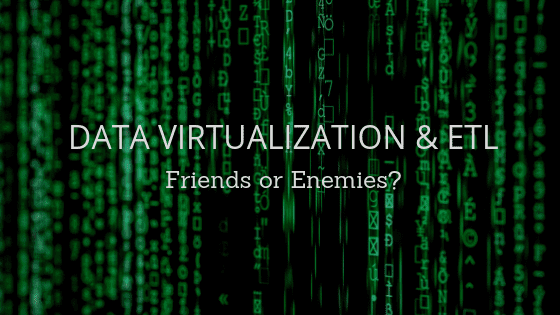
Data Virtualization, which is the process of creating an abstraction layer above the physical implementation of data, has been touted as a possible solution. The technology eliminates the need to manually access each system and create point-to-point integrations, thereby saving time and developer resources.
The question is: can ETL and Data Virtualization work hand in hand or is it more like a one-or-the-other decision for a business?
ETL and Data Virtualization Work Together, Not Against Each Other
Contrary to popular opinion, ETL and Data Virtualization serve unique but complementing purposes in the data journey of an enterprise. ETL, which is a more suitable technique for bulk movement of data, helps businesses migrate data from legacy systems to a new application, as well as populate their enterprise data warehouse (EDW). Data Virtualization, on the other hand, abstracts data from multiple sources and creates a virtual view for business users who want to access and query data in a near real-time manner.
Here are a few use-cases where Data Virtualization can be used to extend, and not just replace, the capabilities of an ETL deployment:
Prototyping an EDW
The process of building a data warehouse, when done the traditional way, can be time- and resource-intensive. Enabling access to heterogeneous data sources requires establishing connections to various modern and legacy databases and use of complex transformations to convert data into the required format. Even after all the hard work, several iterations are needed to build an enterprise data warehouse that can fulfill the business-specific data analysis and reporting requirements.
To prevent all the back and forth, businesses can use a Data Virtualization solution to test data warehouse requirements without having to first build point-to-point integrations and complex mappings to extract, transform, and load the data.
Consolidating Hard-to-Integrate Data
ETL continues to be a good match when dealing with structured data, i.e., the data that resides in relational databases. However, 80 percent of data that enterprises collect or generate is unstructured or semi-structured. To liberate this unstructured data trapped within machine logs, PDF forms, text files, and more, businesses must deploy an unstructured data extraction solution. Another viable solution is Data Virtualization using which enterprises can combine unstructured content with traditional structured data and get a unified, complete view of the picture.
Building a More ‘Responsive’ EDW
The schema of a traditional data warehouse is defined based on the schema of the individual data sources and the relationships between them. Adding new data sources or making changes to the schema of an operational database is therefore not possible without disturbing the structure of the traditional data warehouse. However, a rigid data warehouse is not an ideal choice either in today’s highly dynamic, data-driven landscape. The answer is a ‘responsive’ data warehouse built using a hybrid approach.
Data Virtualization can be used for virtualized integration of all enterprise data and for adding new sources without any significant rework. However, for successful virtual integration of data, it is crucial that the data is first prepared for consumption using ETL. Therefore, a hybrid approach is needed to build a data warehouse that can fulfill data analysis and reporting requirements today and tomorrow.