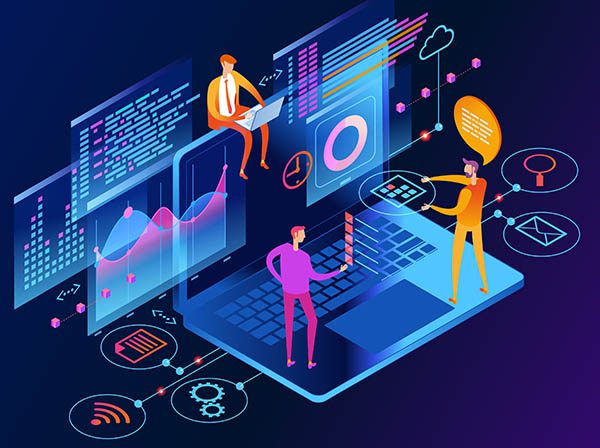
For any transformational Business Strategy, data-driven intelligence must be “readily accessible, interpretable, and actionable” whenever necessary. At the macro level, a data-driven culture has to be consciously propagated throughout the organization, end-to-end.
Digital businesses have acknowledged the importance of data accessibility and have embraced this culture within their business model to remain competitive. The late adopters or data-culture beginners can more quickly adapt to a data-driven Business Strategy by aligning their core business goals with organizational Data Strategy goals. A data-driven Business Strategy will ideally combine the best practices of Data Science with the best practices of business for higher efficiency, better performance, more productivity, higher profits, and lower costs.
At the micro level, data technologies drive decision-making with function-specific KPIs across business functions like HR, finance, operations, or marketing. KPMG’s Data-driven Business Transformation stresses that:
“The tools, techniques and processes that comprise the field of data & analytics are critical to improving standard, day-to-day data and transaction processing (for lean finance).”
What Can Businesses Do to Build a Winning Business Strategy?
According to Asha Saxena, CEO of Future Technologies Inc., very few organizations “align their Data Strategy with their business objectives.” In Why Your Data Strategy Needs to Align with Your Business Strategy, Saxena explains aligning Business Strategy with Data Strategy.
Central thoughts related to building a data-driven business strategy include:
- Linking data to business impact: If the purpose and use of business data is directly linked to the core business goals, then perhaps the outcome will be profitable data use cases. In many cases, business leaders and operators fail to map their available data use cases with actual business needs, and this is why even the most planned Business Strategy fails to yield the expected results.
- Strategic management buy-in of data policies and programs: This can only happen when data is demonstrably linked to realized business impact. The management buy-in strategy has to continue through several cycles of convincing, converting, setbacks, and renegotiating. Unless the buy-in strategies are carefully designed for all levels of staff, the wider business users in an organization will not adapt the data-driven culture so easily.
- Data use cases to be regularly tested: The use cases, as and when tested,can lead to better plan modifications and even business strategy modifications. Unless the data case plans are regularly monitored and tested against actual business situations, the gap between the desired results and the actual outcome may be hard to overcome.
- Adobe’s data-driven operating model (DDOM): This business model has proven beneficial for Adobe’s customer engagement and management programs. Adobe uses DDOM to decide whether a customer is about switch vendors or whether that same customer is a good target for other Adobe products and services. Adobe’s DDOM model can act as a leading model for businesses struggling with their existing CRM programs. After all, if enterprise Data Strategy cannot enhance customer relationships, then what is it good for?
- A Datanami article suggests that customer Data Governance is a key component of an effective Business Strategy.
Thus, it is probably safe to assume that the cultural aspect of a data enterprise is the key driver for future success of data-driven decision-making at all levels. McKinsey shares the function-specific benefits of a robust data-driven Business Strategy in Seeing Your Way to Better Strategy.
All About Data Strategy: Why and How?
A Digital Research Formation Pro study indicates that 78 percent of enterprises believe that big data analytics can transform their businesses in the coming three years. On the other hand, Bernard Marr of Forbes, an expert on Data Strategy discusses the value of an organizational Data Strategy. He believes that “a comprehensive enterprise-wide Data Strategy” can help organizations reap the benefits from their huge data assets. Marr also thinks that a Data Strategy “ lays out a vision for employing data-related or data-independent capability.” He suggests “data’s greatest contributions to any business includes discovering links and patterns across disparate data, predicting customer behavior, and forecasting sales.”
With such excellent endorsements coming from reputable industry spokespersons, data assets have to have hidden values, which can only be discovered with the help of a strong Data Strategy. The Data Strategy, ideally, is not just a preoccupation of the Chief Information Officer (CIO), but begins at the Chief Operating Officer (COO) level, and works its way through Chief Data Officer (CDO), down to the grassroots levels of the enterprise.
What Can Businesses Do to Build a Winning Data Strategy?
While the Business Strategy is relatively easier to build if a sound Data Strategy is already in place, unfortunately the reverse is not true. A thriving business may have an established business strategy, but when that great Business Model has to be reshaped and realigned to accommodate a burgeoning Data Strategy, the results may or may not work according to plan. The Data Strategy belongs to highly educated “technologists” or data scientists, who are of a completely different stock. They are highly tech-savvy, knowledgeable in data matters, but they may not understand the business.
As much as business strategists, who are generally the C-Suite executives, have to be inducted into the science and craft of data technologies, the data scientists have to be equally trained about business goals and objectives. In a way, the Business Strategy vs. Data Strategy game may be an advanced “game of strategy” where equally perceptive minds from different disciplines have to appreciate and understand each other’s roles in an organization. This Forrester article highlights how a third-generation Enterprise Data Strategy can not only enhance operational processes but can also significantly enhance the customer experience.
The technologists have to begin at the macro level, spending time with business leaders, understanding and monitoring business processes, experiencing hands-on the daily aspects of a particular business — then begin to devise a winning Data Strategy, which will work toward fulfilling the overall business goals and objectives. Three Keys to Building a Data-Driven Strategy highlights the following capabilities that lead to success with data and analytics:
- Enterprises must have the technological infrastructure for “combining and managing” disparate data sources and types.
- Enterprises must have access to advanced technology for building predictive models.
- Business leaders must have the convincing capability to transform organizational culture into data-driven decision-making.
In other words, the problem is simple. All existing business plans, programs, and processes will now have to be remodeled through an intricate data infrastructure where data-technology reigns supreme. The ultimate goals are to make all business processes super-efficient, reduce operating costs, and increase profit margins. Data and data technologies are the core drivers of this new infrastructure and all benefits have to be realized through the use of data.
Keeping the above goal in mind, data strategists have to take senior business folks into their confidence, without whose help a seemingly well-planned Data Strategy may fail to deliver results.
Understanding a Winning Data-Driven Strategy through a Case Study
There’s No “Data Strategy” — Align Insights Priorities To Your Business Strategy points out that Data Strategy must be adjusted based on changing business priorities and objectives. The role of an ideal Data Strategy, according to this author, is to support the business objectives in the best possible manner. The article makes a case for when it is better to focus on revenue generation (market share) as opposed to cost savings.
The Canadian telematics company Geotab supplies GPS systems for tracking and managing vehicle fleets. Its CEO, Neil Cawse, is a software engineer by trade, and founded the company 17 years ago. Cawse said: “You don’t know what you don’t know, but the data can tell you that. Much of the growth in our company is due to our focus on being data-driven.”
In Geotab, the Data Science team is not locked behind a data center. Each business function or department has its own data scientists to help that “micro-unit” run more efficiently. However, departmental data is shared centrally (in one location) for enterprise-level “comparisons and analysis.”
Here are the direct benefits that Geotab has realized by having a well-planned, data-driven business strategy that precludes a Data Strategy:
- Every decision, regardless of function, is based on data-centric evidence. The insights gained from available data are shared on big-screen TVs for the benefit of teams who need them.
- Data assets are not kept within the restricted access of senior executives. Every team member takes part in data analysis, runs their own queries, and mines insights for informed decision-making for individual roles in the business.
- Customer issues are fixed in real time through graphical dashboards that bring performance problems occurring in remote locations close to data analytics teams.
- The next step is implementing “embedded analytics” in business processes, so that the mainstream mindset revolves around data. Geotab encourages its staff to think through data.
In another case, an important U.S.-based bank used a transformational Data Strategy with a strong governance model to set its business on track to realize $2 billion worth of benefits.
Technology Choices for Organizational Data Strategy
Of course, the choice of technologies used in enterprise Data Strategy can make a huge difference to the overall business strategy initiative. The types and costs of data infrastructure will control the ability to extract data from departmental silos and create secure and compliant data integrations — preparing the data for meaningful analytics.
A common problem identified during the Data Strategy planning stage is the existence of legacy systems or inappropriate hardware. The existing infrastructure often cannot be ignored for cost reasons, and has to be retrofitted with new data infrastructure. Then comes the choice of software technologies. The lack of sufficient in-house Data Science talent can lead to gaps in understanding, resulting in diluted priorities and misplaced goals.
Data Management vs. Data Strategy: A Framework for Business Success, quotes Donna Burbank, MD of Global Data Strategy as saying:
“There’s so much more opportunity than just doing what you do better. Entirely new business models can be created through well-managed data and a solid Data Strategy. But you can’t do the strategy unless all the people are aligned, and there are processes for how to manage the data.”
Image used under license from Shutterstock.com