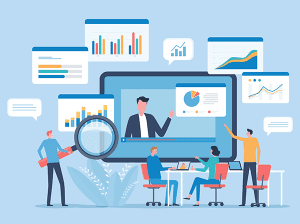
Data strategy trends for 2025 reflect a growing paradox: While organizations race to adopt generative AI and automation, their disconnected data initiatives are creating new silos rather than breaking them down.
DATAVERSITY’s® 2024 Trends in Data Management survey highlights this growing challenge, with 68% of respondents citing data silos as their top concern – up 7% from the previous year.
Leading organizations are addressing these challenges by prioritizing comprehensive data strategies that bridge these gaps. While 37% have already begun this journey, 2025 will see more companies focusing on five key strategic trends that enable enterprise-wide data integration:
- Aligning data and AI strategies with the business needs
- Making data quality a foundation
- Investing in strategic governance
- Integrating architecture components
- Building enterprise-wide data literacy
These trends reflect a crucial shift as successful organizations move beyond tactical data management to create unified strategies that deliver measurable business value.
Aligning Data and AI Strategies with Business Needs
Increasingly, business goals across industries will involve generative AI, with Gartner predicting over 50% of organizations deploying such projects by 2025. Their success depends on two critical factors: communicating AI initiative value and understanding the required data resources for AI productivity.
To get there, companies must focus on data strategies and roadmaps that support AI initiatives. An Informatica survey shows that 40% of Asian-Pacific data chiefs prioritize delivering reliable, consistent data for generative AI alongside improved governance processes.
The challenge becomes connecting data partners, practices, and platforms with AI needs, while developing value-driven policies the business wants. Organizations must orchestrate data resources to fuel AI growth, efficiency, and innovation, while minimizing bottlenecks.
For example, if a corporation’s strategy focuses on disruptive AI innovation, it must account for greater flexibility and uncertainty than in a more mature market. Technical debt will translate into the time and money spent fixing data issues and allowing for experimentation. While such investments may initially show red ink, a well-planned data strategy can accept calculated risks with clear paths to future profitability.
Making Data Quality a Foundation
As organizations pursue AI initiatives and other strategic goals in 2025, data quality emerges as the foundation for success. Current data governance initiatives are limited to tactical approaches for specific apps or data systems. Without unified policies, rules, and methods that apply across and beyond the enterprise, poor data quality will remain a growing concern, with 56% of data leaders struggling to balance over 1,000 data sources.
In 2025, leading organizations will make data quality central to their enterprise-wide strategic planning by:
- Investing in automated quality monitoring and remediation capabilities
- Inventorying their data across the organization
- Defining and applying quality metrics that tie back to business objectives
- Ensuring data is consistent and integral to comply with regulations and foster trust of customers and business partners.
For example, modern workplaces that embrace a holistic approach to data quality report a 55% boost in productivity. This efficiency is especially crucial as 60% of organizations integrate generative AI into their marketing efforts. These marketing projects will need good data quality to get the best performance and results with AI.
Investing in Strategic Data Governance
Data governance is evolving from a compliance checklist to a strategic imperative, with organizations implementing company-wide data roles, practices, and technologies. The DATAVERSITY TDM reports over 90% either have some sort of data governance program or are planning to implement one.
Yet viewing governance solely through a compliance lens leads to underinvestment, with 54% of data and analytics professionals finding a lack of funding for their data programs, the biggest hurdle.
This challenge will spur transformation, as 40% of senior data managers are expected to reposition their programs as business enablers by 2027. Additionally, as part of a data governance strategy, companies will focus on recalibrating organizational behavior, a long-term change journey.
The transition will lead to companies moving data governance from a cost to a profit center. Organizations will embrace this idea as a Data as a Service (DaaS) strategy. Like Software as a Service models, DaaS will provide on-demand data access bundled with content and intellectual property, a potential new revenue stream for organizations.
This evolution becomes increasingly vital to keep pace with the competition and with 50% of developing countries enacting generative AI-related regulations by 2028. In successful organizations, data governance funding will grow to both ensure compliance and maintain the data quality required for marketable products.
Integrating Architecture Components
Compounding data quality issues is a need to integrate data architecture components, the infrastructure that makes data accessible throughout the entire organization. Like data governance and data quality, data architecture will require a unified strategic approach, aligned with business objectives and consumption needs.
Data integration across multiple sources needs the single, overarching guidance provided by a good data strategy. Such a policy needs to consider the impact of data storage on sharing and usage, after data creation. It needs to improve data delivery, so it is easy to package and distribute.
Some companies will consider ambient intelligence, a combination of technologies including very low-power wireless technologies, energy harvesting, and low-power electronics sensors to collect and handle data related to loss, damage, or suboptimal performance.
Additionally, many will include data fabric architectures to make data more accessible and manageable in varied organizational ecosystem. While data fabric and other technologies play a crucial role in bridging data silos, they ultimately need to support the organization in achieving its goals. So, any architectural component of a data strategy will require business influence, input, and sponsorship behind it.
Building Enterprise-Wide Data Literacy
If an organization’s data strategy is to successfully tie in AI projects, governance, data quality, and architectural components, then organizations need to ensure skilled people. Advancing enterprise-wide data literacy, the capability to understand, analyze and use data during work, will become crucial to successful data strategies.
According to 42% of global data leaders, improving data literacy is considered the second most important measure of data strategy effectiveness. This figure will only grow, as by 2027 more than half of chief data and analytics officers (CDAOs) will get funding for data literacy and AI literacy programs. Organizations will recognize that failed AI initiatives often stem from insufficient data skills.
As organizations add data literacy to their strategies, they will integrate training and education, promoting data awareness, role-based training, and continuous learning. Some organizations will invest heavily in a technical platform that addresses these components.
However, companies should take a comprehensive approach, and go beyond the data dashboard. They will need to expand their data literacy and training initiatives to include people and processes.
Any data literacy investment needs to align with business objectives, so that data professionals and subject matter experts have the tools and skills to fulfill their duties. Providing a collaborative and innovative environment will prove equally important to fostering data literacy. A data strategy needs to focus on this.
Conclusion
Organizations will take a comprehensive approach when revamping their data strategies. Their strategic roadmaps will emphasize business alignment, governance investment, data quality, infrastructure integration, and data literacy enhancements.
Through this holistic perspective, executives will need to make organization-wide influence a top priority in creating and implementing their data strategy. That way businesses can bridge data silos and demonstrate data’s value.