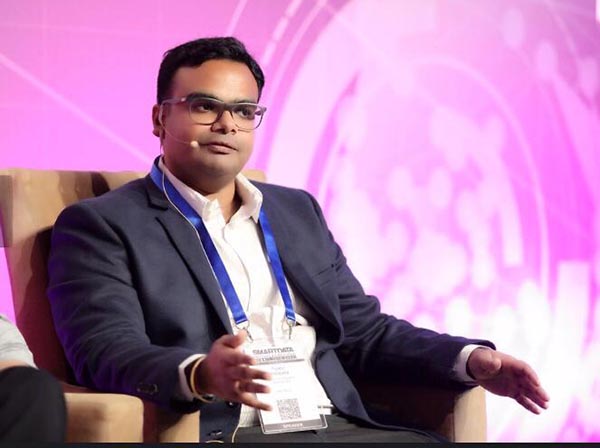
Click to learn more about author Tejasvi Addagada.
Clean Data is a crucial need to get an outcome from Machine Learning capabilities. Scale and diversity in data is also another important aspect. How accurate is the data to give a usable outcome – is a major question? Accuracy
What is easy to access – are the machine-learning services and algorithms, but data is still the prime constituent of AI. The basic predictive efficiency of AI models is defined by diversity, scale and quality of input data. Coverage & Availability.
Most of the data with Information aggregators or large institutions is not consistent across systems and processes, while it is also not consistently formatted across the organization. Structural Consistency & Semantic Consistency.
Data or Information in a common financial service landscape, is usually available across disparate systems. These systems create, acquire, store, maintain and archive data in varied ways. Here is the challenge in terms of integrating and aggregating data perhaps in a common data lake as an input to AI based services. Integration.
AI is driving the need to build real-time data flows across institutions to access essential data. Real-Time data flows are still a far cry for most organizations. Here is the next challenge of architecting data flows that can assist in making available streaming data with less Information lag to AI based services. Lineage & Currency.
We are not just referring to internal data that can be partly trusted but also to external and public data that is also required for scaling the data to AI use. The organizations will have to fix irregularities in missing data and invalid data. Completeness.
Data Governance Gives the Direction to an Organization to Embrace AI
Organizations would also want to monetize their data as it is proprietary data while AI would necessitate that this data must be shared with competitors to reach minimum requirements of efficiency. Monetizing and data sharing need to be addressed with great efficiency in direction and Guidance. Corporate Guidance & Policy.
Nevertheless, Financial services Industry participants are making large-scale investments in Artificial Intelligence. However, Regulators are eyeing substantial uncertainties that need to be regulated through guidance in the form of policy, in the use of AI in the banking and financial institutions. Collaborative solutions built on shared data-sets will radically increase the accuracy, timeliness and performance of non-competitive functions. But is there Governance, Guidance and Oversight over the collaboration of data? Data Governance
All the data might not be fit for
purpose or contextual to an AI use-case. Let’s refer to an insurance firm that
uses alternative data like channel usage characteristics, rather than
traditional and passive data to price insurance products for cyber security risk. The vast sources of
external and alternative internal data (perhaps unstructured) might not be
relevant to the context of the outcome
that the model would provide.
This makes it even
more important to simplify and understand the data better before applying it for purpose.Relevancy.
Summarizing the Enablers for Data to be Used for AI
- Data Quality: Accuracy, Completeness, Validity, Currency, Availability, Coverage, Structural and Semantic Consistency
- Data Governance: Corporate Guidance & Policy,
- Content Management: Relevancy, Lineage,
- Architecture: Data Integration, Aggregation