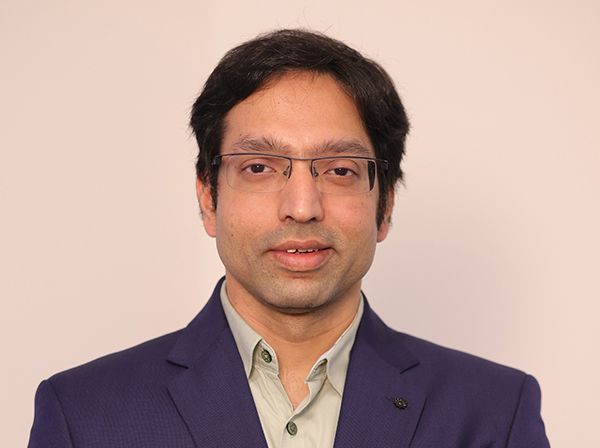
Dirty data – data that is inaccurate, incomplete, or inconsistent – costs the U.S. $3.1 trillion per year, according to IBM. Along with the staggering cost, it prevents health care stakeholders from realizing the enormous potential value that they could be realizing from downstream analytics, including population health management, value-based care, and digital health. Health plans will need to adopt best practices for data quality and artificial intelligence (AI) in order to discover the hidden potential of dirty data in health care.
As we approach 2023, we can expect to see health plans deprioritize manual data entry and switch to machine learning (ML)-based techniques, which are cost-effective, faster to implement, and easier to manage. ML algorithms can learn from data itself – you only need to feed ML systems large historical datasets and they can automatically discover hidden patterns and useful insights. Specifically, AI will be used to solve problems caused by dirty data. Some examples:
- Detecting data quality issues in clinical data: This can better monitor care provided to patients.
- Identifying misattributions in value-based care programs: This can ensure patient care and the costs of that care are attributed to the right physicians, aligning incentives across stakeholders.
- Detecting overpayments in claims processing: This can ensure that providers are fairly compensated and that payers are reimbursing the right amounts.
- Detecting inaccuracies in provider directory data: This can prevent surprise billing for patients and greatly improve the member experience.
Using AI to Move Health Care Operations from Reactive to Proactive
Health care AI will soon move health plan operations from reactive troubleshooting and response to proactive detection and action. For this to happen, AI and ML systems will have to work in real time. This can be achieved in a few distinct ways.
One way to realize proactive, or predictive AI, is to have a closed-loop MLOps-based system where ML model training happens in the background. The system then applies those models on live, real-time data. The system monitors the quality of prediction and if it degrades, this triggers an automated closed loop that retrains the data to generate a new model. The system then automatically puts the newer version back into a streaming prediction pipeline.
In the health care industry, a proactive, real-time AI/ML approach can have impact in multiple areas:
- Claims overpayment: Suspected overpaid claims can be blocked and sent for review before reimbursements are distributed, saving the effort and cost of post payment recovery.
- Attributions in value-based care: AI can be used to detect real-time misattribution to prevent any potential care issues or financial losses.
- Provider directory accuracy: This can avoid surprise billing for patients, save payers fines from CMS, and improve the member experience.
- Clinical data accuracy: This can result in improved HEDIS/STARs ratings, more accurate risk adjustment scores, and better and more coordinated patient care.
Adopting Data Quality Best Practices in 2023
2023 will be the year that the data quality issue in health care will come to the forefront. The federal government is looking at provider data quality more seriously (with the CMS National Health Directory proposal, for example) and it is becoming more apparent that ML-based interventions in health care cannot meet their promise in the real world due to poor data quality.
If health care providers and health plans continue to rely on dirty data, the system won’t realize the benefits and promise that is possible. AI/ML-based data quality management can refine dirty health care data and magnify its power, improving member experiences and delivering results.