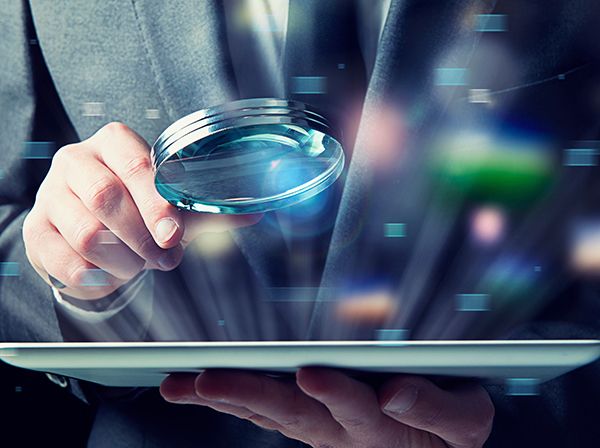
As a process, data observability is used by businesses working with massive amounts of data. Many large, modern organizations try to monitor their data using a variety of applications and tools. Unfortunately, few businesses develop the visibility necessary for a realistic overview.
Data observability provides that overview, to eliminate data flow problems as quickly as possible.
The observability process includes a variety of methods and technologies that help identify and resolve data issues in real time. This process builds a multi-dimensional map of a business’s entire data flow, offering deeper insights into the system’s performance and data quality.
When asked about data observability, Ryan Yackel, CMO of Databand, an IBM Company, commented,
“As the volume, velocity, and complexity of big data pipelines continue to grow, companies rely on data engineering and platform teams as the backbones of their data-driven businesses. The problem is that most of these teams have their work cut out for them. They are fighting data with reliability and quality incidents, making it difficult to focus on strategic initiatives involving AL/ML, analytics, and data products. Data observability provides a solution.”
Initially, data observability might seem to be a form of data lineage, but the two processes serve different purposes.
Data observability focuses on resolving problems with the data quickly and efficiently through the use of a measurement system. Data lineage, however, is used primarily for collecting and storing high-quality data – data that can be trusted.
Additionally, data lineage can be used as a component to support an observability program. (Some articles promote data observability as serving the same purpose as data lineage, and there is some truth to the claim. Data lineage is a component of data observability.)
The term “observability” was originally a philosophical concept developed by Heraclitus around 510 BCE. He determined observability required comparative differences – cold can be observed in comparison to warmth. In 1871, James C. Maxwell, a physicist, developed the idea that it was impossible to know the location of all particles within a thermodynamics experiment, but by observing “certain key outputs” for comparative changes, accurate predictions could be made.
Maxwell’s description of observability using key outputs was adapted and applied to a variety of automated applications, ranging from factory equipment to aircraft sensors. The concept was then embraced by DevOps for debugging and dealing with “production incidents,” in approximately 2016. In 2019, Barr Moses – CEO and co-founder of Monte Carlo – developed an observability process designed to provide an overview of an organization’s data flow.
“Data observability is an organization’s ability to fully understand the health of the data in their systems. Data observability eliminates data downtime by applying best practices learned from DevOps to data pipeline observability.”
Five Pillars of Data Observability
Data observability works to resolve data and information issues by providing a thorough map of the data in real time. It provides visibility for the data activities of an organization. Many businesses have data that is siloed, blocking observability. Data silos must be eliminated to support a data observability program.
When activities such as tracking, monitoring, alerting, analysis, logging, and “comparisons” are performed without an observability dashboard, a form of organizational partitioning can take place. People in one department don’t realize their efforts have unintended consequences in another department – such as missing/siloed information promoting bad decision-making or part of the system is down and no one realizes it.
Remember, observability is about taking the measurements of certain key outputs. The five pillars (or key outputs) Barr Moses developed for measurement purposes are:
- Quality: High-quality data is considered accurate, while low-quality data is not. Measurements of the data’s quality provides insight into whether your data can be trusted. There are a variety of ways to measure Data Quality.
- Schema: This involves changes in how the data is organized, and schema measurements can show breaks in the flow of data. Determining when, how, and who made the changes can be useful in terms of preventative maintenance.
- Volume: Large amounts of data are useful for research and marketing purposes. This can provide organizations with an integrated view of their customers and market. The more current and historical data used during research, the more insights.
- Data lineage: A good data lineage program records changes to the data and its locations, and is normally used to improve data quality. However, it can also be used as part of a data observation program. In this capacity it is used to troubleshoot breaks that might occur, and list what was done prior to the damage.
- Freshness: This is essentially about not using old information, or, as Barr Moses refers to it, stale data. Freshness emphasizes up-to-date data, which is important when making data-driven decisions. Timestamps are commonly used to determine if the data is old.
When combined, the measurements of these components, or pillars, can provide valuable insights into problems that develop – or simply appear – and promote the ability to make repairs as quickly as possible.
Data Observability Challenges
The right data observability platform can transform how businesses maintain and manage their data. Unfortunately, implementing the platform can present some challenges. Compatibility issues will present themselves when the platform is a bad fit.
Observability platforms and tools can be restricted if the data pipeline, the software, the servers, and the databases aren’t completely compatible. These platforms do not work in a vacuum, making it important to eliminate any data silos from the system and ensure that all data systems within the organization are integrated.
It is important to test a data observability platform before signing a contract.
Sadly, even when all the business’s internal and external sources of data are integrated correctly into the platform, different data models may cause problems. Many businesses support 400 or more data sources, and each external source may present a problem if it is not using the same standards and formats.
Except for open-source tools, observability platforms are cloud-based and they may offer some flexibility that supports fine-tuning.
The best observability platforms are focused on a standardized measurement process and logging guidelines. This promotes the effective correlation of information, but external data sources and customized data pipelines may cause problems and require additional manual efforts to accomplish tasks that should have been automated.
Additionally, some tools may come with unusual storage costs that restrict scalability.
Data Observation Platforms
Data observability platforms typically contain a variety of useful tools. These often include automated support for automated data lineage, root cause analysis, data quality, and monitoring to identify, resolve, and prevent anomalies within the data flow.
The platforms promote increased productivity, healthier pipelines, and happier customers. Some popular data observability platforms are:
- Databand provides a highly functional observability platform that can detect and resolve data issues very quickly, using a continuous observability process that identifies data issues before they impact your business.
- Monte Carlo offers an observability platform that can be described as providing observability “from pipeline to business intelligence.” It brings data reliability to the orchestration of various data services and tools.
- Metaplane features end-to-end observability.
- There are a variety of open-source observability tools available, which would be worth investigating.
The Importance of Data Observability
For organizations dealing with large data flows, observability can be used to monitor the data system as a whole and send out red flags when a problem presents itself.
As businesses collect massive amounts of data from a variety of sources, they develop systems to handle it, layer upon layer. These systems include data storage, data pipelines, and a number of tools. Each additional layer of complexity increases the chances for data downtime from issues such as incompatibilities, or old and missing data.
According to Yackel, “The continuous use of data observability to monitor data pipelines, data sets, and data tables alerts data teams when a data incident occurs and shows how to fix the root cause, before it impacts their business. With data observability, engineering can focus on building great data products rather than maintaining broken processes.”
Data observability will help businesses to proactively identify the source of pipeline issues, data errors, and data flow inconsistencies to strengthen customer relations and improve data quality.
Image used under license from Shutterstock.com