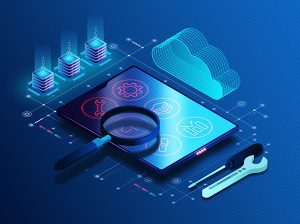
“Data observability” can be described as the practice of monitoring the “health and state” of data pipelines in your system. This practice encompasses some technologies and activities that enable business operators to identify, examine, and solve data-related problems in near real time.
Though organizations rely heavily on accurate and reliable data to make informed decisions, ensuring the quality of this data can be challenging. That is where data observability comes into play.
Data observability is the practice of monitoring and understanding the health, performance, and reliability of data pipelines in real time. It enables organizations to proactively identify and address issues that may impact the quality of their data.
By implementing data observability practices, businesses can gain greater visibility into their data infrastructure, ensuring that it operates smoothly and consistently delivering high-quality insights. This article explores various data observability use cases across different industries to demonstrate how this modern practice helps organizations achieve better outcomes with their data analysis processes.
The Importance of Data Observability
Data observability ensures the reliability, accuracy, and consistency of data throughout its lifecycle. By implementing robust data observability practices, businesses can gain a comprehensive understanding of their data pipelines and systems. With data observability, organizations can identify and resolve data-related issues proactively, preventing potential errors or discrepancies from impacting critical business operations.
The key metrics tracked during data observability processes are data quality, latency, completeness, and schema changes in real time. This empowers data teams to detect anomalies swiftly and take immediate action to rectify them. Ultimately, data observability enhances trust in the organization’s data by providing visibility into its health and performance.
Data Observability Key Benefits and Applications
Data observability offers several benefits and applications in the field of Data Management and analytics. The main benefits are the ability to gain real-time insights into data pipelines for early detection of anomalies or issues, and the capability to optimize Data Quality by providing visibility into data transformations, schema changes, or any other modifications made to the data.
Moreover, by monitoring data pipelines comprehensively, organizations can enhance their overall operational efficiency and reduce downtime. This leads to improved decision-making capabilities based on reliable and trustworthy information.
Data Observability Use Cases
The importance of data observability cannot be overstated. Without it, organizations may face serious consequences such as making incorrect business decisions based on flawed or incomplete data. Additionally, without visibility into their data pipelines, organizations may struggle to meet compliance requirements or fail to detect security breaches in a timely manner.
By implementing robust data observability practices, organizations can ensure that their critical business processes are supported by reliable and high-quality data. Here are some important data observability use cases to know:
- Data reliability and integrity are critical to the success of modern organizations. By closely monitoring and analyzing data pipelines, organizations can identify and address potential issues that might compromise the quality of their data.
- Data Quality monitoring: Data observability enables organizations to continuously monitor the quality of their data. By tracking various metrics such as completeness, accuracy, consistency, and timeliness, organizations can identify any anomalies or errors in their datasets. This allows them to take proactive measures to rectify the issues before they impact critical business decisions.
- Anomaly detection: Data observability helps in detecting anomalies within data pipelines or datasets.
- Data lineage tracking: With data observability, organizations can trace the origin and transformations undergone by their data throughout its lifecycle. This helps in understanding how different processes and systems impact the reliability of the final dataset.
Data observability applications are becoming increasingly essential for organizations across various industries. Here are some real-life applications highlighting its importance:
- Fraud detection: Financial institutions employ data observability to monitor transaction data in real time, identifying any suspicious patterns or anomalies that may indicate fraudulent activities.
- Customer experience optimization: Companies leverage data observability to collect and analyze customer feedback, behavior, and usage patterns. This enables them to identify areas for improvement and enhance the overall customer experience.
- Operational efficiency: By monitoring key performance indicators (KPIs) and system metrics, organizations can ensure smooth operations, detect bottlenecks or errors promptly, and optimize their processes accordingly.
Sector-Wise Data Observability Use Cases
While the concept of data observability is applicable across industries, each sector possesses its unique challenges and requirements. This section highlights sector-wise use cases for data observability across key industries such as finance, healthcare, and manufacturing.
By delving into these specific sectors’ use cases for data observability, you can gain a deeper understanding of how organizations leverage this practice to enhance operational efficiency, improve customer experiences, optimize resource allocation, mitigate risks effectively, and drive innovation.
In the manufacturing sector, real-time data analysis can help optimize production processes by detecting anomalies such as equipment malfunction or production inefficiencies. By leveraging advanced analytics techniques like machine learning algorithms, organizations can proactively identify potential issues and prevent costly downtime or quality defects.
In the financial sector, real-time data analysis plays a crucial role in fraud detection and prevention. By analyzing transactional data in real-time, banks and financial institutions can identify suspicious activities or patterns indicative of fraudulent behavior. This enables them to take immediate action to mitigate risks and protect their customers’ assets.
Real-time data analysis is also beneficial in the healthcare sector. Healthcare providers can monitor patient vitals or medical device readings continuously to detect any abnormal fluctuations that could indicate potential health risks. This allows for timely interventions and enhances patient safety.
Real-time data analysis combined with anomaly detection empowers organizations across sectors to optimize their performance by identifying issues promptly and taking proactive measures for enhanced efficiency, productivity, security, and customer satisfaction.
Compliance Monitoring and Risk Management
Compliance monitoring and risk management are critical aspects of ensuring the integrity and security of data in various sectors. By leveraging data observability, organizations can effectively address compliance requirements and proactively mitigate potential risks.
In the financial sector, data observability plays a key role in monitoring transactions for suspicious activities, ensuring compliance with anti-money laundering (AML) regulations, and detecting fraud. By continuously monitoring data pipelines and analyzing patterns, anomalies can be identified promptly, enabling timely actions to prevent financial crimes.
In the healthcare industry, data observability helps ensure compliance with industry regulations such as the Health Insurance Portability and Accountability Act (HIPAA). Through real-time visibility into data flows, organizations can identify potential breaches or unauthorized access to sensitive patient information. This enables them to take immediate action to address any vulnerabilities in their systems.
In highly regulated sectors such as the pharmaceuticals or energy sector, where strict safety protocols are paramount, data observability is crucial for risk management. It allows organizations to monitor processes and systems continuously for deviations from expected norms that may indicate potential safety hazards or non-compliance with regulatory guidelines.
Image used under license from Shutterstock