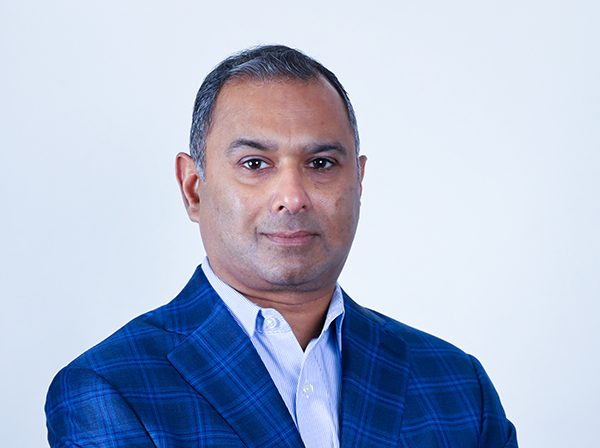
The quality of the data you use in daily operations plays a significant role in how well you will generate valuable insights for your enterprise. You want to rely on data integrity to ensure you avoid simple mistakes because of poor sourcing or data that may not be correctly organized and verified. That requires the use of data observability tools and partnerships with users capable of using them to the full advantage needed.
Defining Data Observability
In simple terms, data observability refers to monitoring the progression of a data flow lifecycle from data sources through repository and through any transformation cycle. With data observability, you have the advantage of knowing about potential incompletions or errors prior to the data visualization or data automation phase.
When users access your company’s data, they shouldn’t see only one small part of it. Instead, they should see all the information they need to achieve their goals and make decisions based on that information. That is what makes for an easy-to-observe dataset – providing decision-makers with a clear view of their current state so that they can act accordingly.
Data observability means that companies can address issues quickly before those issues negatively impact business goals and outcomes. This helps prevent problems before they happen instead of trying to fix them after they arise.
Components of the Data Observability Lifecycle
Data observability open-source solutions are a set of practices, technologies, and tools that help organizations with their data-driven strategic plans. Every business organization designs its own data operations lifecycle (DOL), which is a standardized way to carry out these practices. A well-rounded DOL provides the following:
- A plan for change whenever you detect surprises early
- Automation of critical tasks that involve the data lifecycle
- Improved quality, efficiency, and predictability of your data and its journey
- Trust within the organization that the data you are observing is of high quality
The data observability pillars include metrics, traces, and logs. These allow your organization to visualize and set up alerts for any risks while assessing the overall health of our data. This way, you can conduct audits and analyze logs to generate reports for decision-makers.
Business Need for Data Observability
We live in a highly competitive data-driven world. Without a form of data observability tools readily available, your organization will suffer. You need real-time insights into your data so you can respond to different market trends and consumer needs.
This is the major difference between data observability versus monitoring. When you monitor data, you know if something is wrong. When you use observability, you can determine the cause behind the problem. You can go a stage deeper to ensure these root causes are remedied, so they do not occur in the future.
Businesses require this approach because it helps them understand and control how their data is used and flows through the lifecycle. Without it, there is no way to understand and analyze exactly what is happening and how it can help the organization as a whole.
Alternative Approaches to Implementing a Data Observability Solution
You may have already started implementing a data observability solution, and you might be wondering what other approaches exist. Other ways of implementing a data observability solution are by using a data platform, building it into the platform you already use (such as your data warehouse), or using open-source data observability tools. All of these methods offer their own unique benefits and challenges.
Use a data platform: If you’re not already familiar with them, data platforms are designed to manage all your organization’s data within one place and give you access to that data through APIs instead of having to go into the platform itself. There are many benefits to using a data platform: fast, easy access to all your organization’s information; flexible deployment options; increased security; and more. Additionally, many modern-day platforms incorporate built-in capabilities for data observability so that you can ensure that your databases are performing well without having to implement an additional solution.
Build data observability into the platform you already use: If your organization is currently only using one application or tool for managing its databases, then choosing this approach is probably best for you if your platform provides you with an observability function.
However, if you rely on complex Data Management from multiple sources, then integrating data observability into your current configuration will be a key requirement. This will lead to greater reliability on the data flow cycle you use for decision-making.
Technology + Integration
Data observability solutions are often built on existing Data Management platforms, using a combination of open-source and proprietary technologies to address the challenges facing data operations teams.
This enables you to use your favorite tools without additional infrastructure or significant upfront investments. Because of this, you can use these data observability tools to get started with data observability quickly. That is something you’ll never be able to do with home-grown software solutions that require significant engineering effort, which makes them impractical for most companies (and especially startups).
There are many investments required to implement a solution, including investing in data collection and management, investing in monitoring technology, and investing in the people, process, and technology. But what’s more important is making sure you’re putting your investments in the right place. This is where identifying a successful outcome will help.
The alternative is to hire a company that already has these tools integrated into their technology-enabled services. This way, you are paying a basic rate for an all-inclusive solution instead of having to invest in multiple technologies across many different verticals.
ROI
When examining the true impact of a new technology, it’s particularly important to consider the return on investment (ROI) you’ll get from its use. But since data observability is such a broad and encompassing topic, it’s been hard to pin down exactly what its ROI consists of. Let’s break the concept down into five components that form the basis for the ROI calculation:
Financial: A financial calculation of ROI considers all costs incurred in creating and maintaining the new system, including hardware, software licensing, equipment maintenance and replacement, labor (including both development time and ongoing operations), energy consumption, etc.
Operational: The operational component includes the operational efficiencies gained by improving data utilization or eliminating time spent on manual processes that can now be handled automatically – things like eliminating downtime due to inaccurate or incomplete data or spending less time acquiring data manually and more time analyzing it for insights.
Analytical: This refers to gaining a competitive advantage by using data analytics tools more effectively to improve decision-making processes and forecast trends with greater accuracy than your competitors. One example includes improving customer satisfaction through faster response times based on better insights into customer needs. A significant component here is how much confidence people have in their ability to make decisions based upon their analysis of those results. When managers and executives know that they can expect consistent high-quality information from their analytics, then they will have confidence in making important business decisions based on that information to improve company performance.
Service Quality
The goal is to integrate data observability throughout your DataOps process. That requires careful consideration of the quality of your data so you can minimize data downtime or errors in the operations you are using for decision making.
You need to consider the capabilities of any partnerships you engage in for managing your company’s data flow. They should be checking for:
- Is the data up to date?
- Is the data being distributed to the correct silos for interpretation?
- Is the data complete?
- Can you track any changes to the data and who/what made them?
- Is it easy to trace the lineage of the data from the source all the way to reports or dashboards?
The service you receive should be secure to alleviate any peace-of-mind issues with your enterprise. You want extreme reliability with your service quality so that whenever you need pertinent information with anyone in your enterprise leadership or structure, it is readily available.
Advantages of Data Observability
Data observability also offers several additional advantages, including:
- The ability to track the flow of data through your systems. Data observability can help you track where your data is going and whether it’s being used for its intended purpose.
- End-to-end visibility of the full data operation lifecycle. This includes how your data is being collected, stored, analyzed, and visualized – as well as how that information is used to improve business processes throughout your organization.
- The ability to monitor the performance of your data in real time by capturing all changes across systems over time while providing an audit trail of those changes.
Getting Started
Data observability is essential to driving better data quality and ensuring valuable business insights. We recommend always beginning with a discussion on your specific business outcomes. Assess if you have the expertise and the automation infrastructure to help you avoid data downtime and improve data pipeline quality.