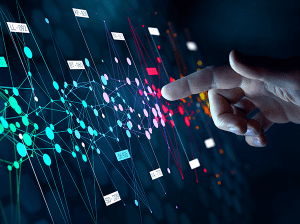
Data modeling, the practice of diagramming business requirements, has grown significantly. According to a 2024 DATAVERSITY® survey on data management trends, 64% of organizations actively use data modeling, a 13% increase since 2023.
This trend will only increase in 2025 as companies face heightened opportunities and risks. Newer technologies and AI are set to disrupt predictive analytics, especially with huge volumes of data and thousands of parameters.
Simultaneously, demands for clear models have never been greater. Organizations face challenges from a labyrinth of regulatory requirements, demands for good data quality, and increasingly complex data ecosystems.
To handle these issues, companies will demand quality, up-to-the-minute accurate models describing data assets, how they flow, and their relationships. In addressing all these imperatives, data modelers will need to simplify complex business problems more quickly and efficiently.
Data modeling trends driving how well engineers can do this include:
- Increased impact of AI
- Better flexibility and scalability
- Enhanced data quality and improved trust
- Greater interconnectedness with data governance
This article will show how these patterns will impact data modeling in 2025.
Increased Impact of AI
AI, a technology simulating human thinking, will increasingly become common across most of the marketplace. Already, over 66% have adopted an AI technology for at least one business function. As AI usage increases in 2025, organizations are advancing their applications for gathering insights.
Predictive analytics and automation from generative AI models will become a must-have. Analysts will work with AI-driven decision support systems, automated analytics dashboards, and intelligent business process automation tooling to get relevant insights quicker. Data modelers will present these components when diagramming business use cases and their data-driven solutions.
Also, organizations will demand more visualizations to understand where, when, and how AI uses data assets. This information will support data privacy and adherence to regulations. Especially where only 12% of organizations report sufficient quality for AI implementation, companies will rely on data modelers to ensure AI functions with accurate, reliable, and ethical data.
Fortunately, data modelers can access powerful AI functions that automatically suggest data models and optimize databases for better understanding. For example, AI can analyze existing databases and automatically generate initial data models, saving time, reducing bottlenecks, and improving performance. Consequently, data modelers will more efficiently address complex business problems.
Better Flexibility and Scalability
As AI accelerates data modeling capabilities, both the AI models and the modelers need to become more adaptable and accommodate more flexible and scalable data architectures. To this end, modelers will face more demands to diagram data flows using different modeling approaches from the traditional Entity-Relationship (ER) model, specifying an enterprise schema to the graph database, which reveals patterns and insights among data and its associations.
Demand will grow for graph database models to clearly interpret data processed in real time and to translate these complex data processes into simple visualizations. Knowledge graphs – interconnected sets of concepts and relationships – promise data modelers a practical approach to describe intricate data systems and the inputs from which AI can learn.
For instance, a knowledge graph could map how customers connect to their purchases – which link to products, suppliers, and shipping routes – creating a flexible web of relationships that can easily scale to accommodate new connections and data sources. When a company adds new product lines or enters new markets, the knowledge graph can naturally expand without great effort.
While organizations use knowledge graphs as one flexible and scalable solution, some will explore a more revolutionary approach: quantum computing. Quantum computing is a new technology that uses a wavelike memory state to scale up computer processing and storage. This expanded capability to model complex scenarios in seconds will benefit time-sensitive use cases, such as financial trading and fraud detection.
So, businesses will continue to add more tools and technologies supporting flexibility and scalability to their data ecosystems. However, this added complexity requires additional attention to data quality for successful flexibility and scalability.
Enhanced Data Quality and Improved Trust
As data ecosystems become more sophisticated in 2025, organizations need to pay even more attention to maintaining high-quality data and building trust. Employees must feel confident about using enterprise-wide data for everyday decisions and work tasks.
To build this confidence, organizations must focus on two key areas: data literacy and data security. On the literacy side, companies need to continuously bridge any data literacy gaps so users know if their data models are relevant and accurate. Moreover, well-established cooperation and communication among modelers and their co-workers will become even more critical to ensuring models are up to date.
On the security side, teams will need to involve data modelers to improve information security by actively identifying security measures and making recommendations. Additionally, teammates will rely on modelers to more efficiently arrange and optimize storage areas and make queries more performant, helping companies save on costs while maintaining data integrity.
Having adequate data quality and trust will give organizations a data modeling foundation that leverages AI and the technical resources, improving flexibility and scalability. Neglect comes with financial penalties, legal liabilities, reputational damage, and consumer mistrust. A proper data governance program and framework ensures appropriate attention to data quality, expanding opportunities, and reducing risks.
Greater Interconnectedness with Data Governance
Data modeling and governance will become more interconnected in their processes and deliveries. Organization-wide governance synchronizes data activities and roles as AI and newer technologies become more available. Data governance delivers guidance and evidence of regulatory compliance and self-service access to needed datasets, e.g., through a data catalog.
Data modelers must support governance efforts to ensure data handling according to regulations. They will show how data systems integrate and clear data lineage. Data governance provides modelers with data quality standards to align visualizations with business expectations.
These requirements rely on metadata management: providing context, content, and structure to diagram data entities, attributes, and relationships. Think of a data model as a type of metadata.
While a governance program manages the metadata, it draws from data models the metadata to manage governance information, such as data ownership, user accessibility, and quality metrics. Then DG draws from the models to improve roles, responsibilities, and processes, leverage opportunities, and resolve issues.
Data modelers, through data governance’s metadata management, ensure their deliverables are more accurate, up-to-date, and compliant. These diagrams depict how the technical architecture is defining and solving business problems and how well this matches up with data governance requirements. By providing these visualizations, data modelers uncover complexity and guide data governance conversations to a crisper understanding.
Conclusion
As 2025 approaches, businesses face a maze of opportunities and risks through AI impacts and resources improving flexibility and scalability. To make sense of these changes, organizations need to turn to data models for clarity.
Data models promise the foundations needed for security and trust, but engineers cannot do this alone – they need guidance from data governance. When governance and modeling interconnect effectively through metadata management, organizations can better leverage newer technologies while ensuring regulatory compliance in the face of complexity.
With the guidance of governance, AI and other newer technologies will augment human modeling capabilities, not replace them. The work processes will change in 2025, but modelers will find more opportunity to upskill to use new tools and present complex business information simply.
In the meantime, data modeling will continue its long practice of transforming business challenges into clear and meaningful insights through visualizations. In 2025, these models will improve with attention to adequate data quality – making gnarly business problems solvable with trustworthy organizational data.