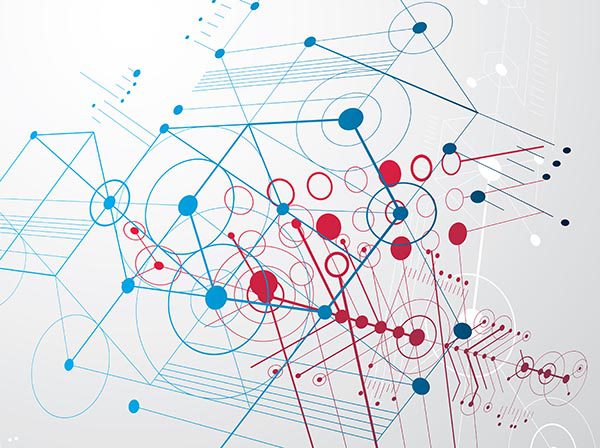
Machine learning (ML) is empowering average business users with superior, automated tools to apply their domain knowledge to predictive analytics or customer profiling. The article What is Automated Machine Learning (AutoML)? discusses a prediction that by 2020, augmented analytics capabilities will play a key role and be a “dominant driver” in the growth (and purchase) of business analytics and Business Intelligence (BI) platforms.
These are not just empty promises to worldwide business leaders; in 2017, the age of automated, ML-powered analytics and BI dawned, and has since transformed one industry sector at a time. The automation revolution has not paused and is likely to storm global businesses in years to come. The era of AutoML is beginning to enable business users to tune existing data models and apply custom models to their everyday business situations as well.
Data Modeling Trends in 2019 talks about the newer challenges facing data modelers and Data Management professionals. It suggests that as “business goals and technology goals continue to converge” across businesses, a new era of Data Modeling will usher in a “part-automated, part manual” process, lending more control to citizen data scientists and therefore, by extension, citizen data modelers.
In Gartner’s study Preparing and Architecting for Machine Learning, the characteristics of machine learning (ML)-aided platforms may be summarized as:
- The hidden power of ML lies in its ability to learn and improve algorithms by studying available data. The higher the data volume, the better the learning process. Bringing this into the creation and management of data models is only starting to be understood
- In order for data pipelines to work in an ML environment, the Data Architecture must be designed to work with the underlying data platform.
- ML works best with Big Data with high-volume data samples. Modeling such samples is still a resources heavy task, but improvements are coming
- IT professionals are now applying ML-enabled intelligence to development and operations activities to achieve operational efficiencies.
The study also proposes several recommended upgrades to existing architectures to accommodate and support the development of ML algorithms and the completion of models through machine learning techniques. Ideally, the selected ML platform should be interoperable with multiple types of ML frameworks to accommodate commercial packages or other service providers. In absence of in-house ML expertise, the public cloud may offer the best solution for ML development work, for its obvious elasticity.
The self-training capacity of an ML algorithm enables powerful automation for modern data platforms. The blog post titled An Introduction to Hashing in the Era of Machine Learning describes a technique called “hashing,” where a large dataset is combined with an ML algorithm to self-train and run a trained model on the dataset.
Machine Learning: What Does it Offer?
More and more, enterprises are looking for democratization of Data Management practices and functions so that they will not need to invest in cost-intensive data centers with expensive data scientists; nor will they engage heavy-duty IT professionals for day-to-day business analytics and other data-intensive tasks.
The new dream of global enterprises is to bring the data centers down to the main business corridor, where an average business user will be able to conduct self-propelled analytics and BI tasks from their desktops, aided by automated AI technologies and tools, which includes Machine Learning technologies.
Machine Learning: An Exciting New Era for Technology talks about making business predictions and finding solutions to problems through the use of automated ML technologies. This article shares the popular belief that ML technologies will empower the average business user beyond anyone’s imagination. Such imagination includes taking the traditional practices such as Data Modeling into the future as well through machine learning techniques to aid modelers in becoming more efficient at their jobs.
Model Management and Machine Learning
- The Resurrection of Knowledge Base Construction (KBCs): Although back in the 1970s Knowledge Base Construction were popular among expert systems, it lost its significance somewhere along the line. However, advanced ML research and development later allowed KBCs to resurface in the form of Amazon Alexa or Google Assistant. KBCs are designed to extract information typically used in question-answering, search, visualization, or supervised machine learning modeling.
- KBCs for Data Model Management: KBCs may also play a significant role in data model management, which is much more than simply monitoring models. Today, model management can aid businesses to “consistently and safely develop, validate, deliver, and monitor models that create a competitive advantage.” Domino Data lab has more in What Is Model Management.
Model Governance in the Machine Learning Era
Currently, global businesses exploit the power of machine learning models for critical decision-making and for extracting competitive intelligence. The SAS Institute blog post Model Governance in the Machine Learning Era offers a business case in support of ML models in corporate decision-making. It offers a warning signal that data models are only as good as their “input data” and their development process. In other words, bad data can surely defeat the purpose of an analytical model it is designed for.
Model governance is the framework through which Data Quality and ML algorithm development process can be monitored, evaluated, and validated. Even automated model development processes can use a governance framework to monitor each step in the whole process.
AI technologies have to depend on the highest quality data for their systems to work. Medium’s Free Code Camp offers some expert advice on how to detect data-related problems early on and then use data-assessment phases to apply additional data cleansing steps to prepare high-quality data for analytics tasks. In the case of deep learning, the emphasis is on “embedded data,” which machines can use to gain knowledge, mimic human behavior, and make predictions. In Deep Learning – The Rise of Machine Intelligence in the AI Era, additional Data Quality related issues are discussed.
Use Cases
In HOLMeS eHealth expert system, deep learning and Big Data technologies have been combined to build human-machine interactions on a healthcare support system. The easy availability of cheap and powerful computational systems and Big Data has made this novel facility possible.
HOLMeS stands for “Health Online Medical Suggestions.” The aim of this expert system is to provide support to several eHealth applications. At the core of the system, an ML algorithm offers preventive medical advice via chatbots and web apps. Powered by deep learning, the chatbot behaves more human-like.
The report on Wireless Networks Design in the Era of Deep Learning explains the use of deep learning techniques along with traditional network design approaches with a mathematical, model-based approach to build future wireless communication networks. The report describes at length how deep learning may be combined with other AI techniques like reinforcement learning and transfer learning to power artificial neural networks, along with particular models, in the development efforts.
Certainly, this is only the beginning of the intersection between these various technologies, but as the machine learning era moves forward, and advanced modeling techniques are further developed and employed within enterprises, this intersection is likely to grow ever bigger.
Image used under license from Shutterstock.com