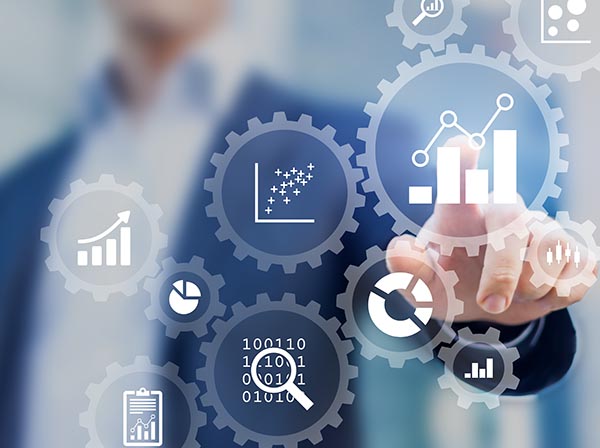
What is a company to do when Business Intelligence (BI), designed to leverage data as an asset, costs too much time and money due to failures in traditional Data Management processes, such as the prepping and cleaning of data?
Should a business analyst, wanting to produce ad-hoc reports, have to be tech-savvy or wait for someone more knowledgeable to help fix these problems? Many firms are turning to self-service BI to make business analysis easier and more intuitive, but Data Management process issues continue to exist upstream, making pure self-service BI cumbersome because of the continuation of poor-quality data.
Businesses need to understand Data Management’s impact on BI. In a recent DATAVERSITY® interview, Mike Brody, CEO of Exago, explored where Business Intelligence (BI) sits and how it intersects with traditional Data Management tasks. Brody defined Data Management in-line with the DAMA DMBOK®:
“The development, execution, and supervision of plans, policies, programs, and practices that deliver, control, protect and enhance the value of data and information assets throughout their lifecycles. Data Management covers all practices and policies put in place to handle data assets.”
Business Intelligence, he said, “describes business data analysis through software tools, primarily to monetize business data.” In Brody’s view:
“When we extract business insights from data, we’re effectively monetizing it. Peter Drucker was right: ‘What gets measured gets managed.’ We measure data with BI because it’s valuable, and we manage data because we measure it.”
If BI is set up with the intent of monetizing data, where do data analysts end up after being drawn into traditional Data Management duties?
Business Intelligence and Data Management: The Data Pipeline
Business Intelligence depends heavily on good Data Management implementation. Brody stated:
“Data Management provides the foundation on which good Business Intelligence rests and determines BI’s form. Effective BI depends heavily on an organization’s structure and its data needs. If data is poorly managed, the report builders on the other end will be frustrated with BI.”
Think of Data Management as a pipeline. Business Intelligence sits at the far end of the pipeline and only sees data as filtered by various Data Management processes such quality control, cleansing, and preparation for later report insights. Data Management issues are magnified once they reach the BI end of the pipeline. According to Brody, how the data is presented in a reporting application reveals a lot about the Data Management processes further up the pipeline:
“When analysts encounter false or stale information, like duplicate records or discrepancies between data models and data semantics, it’s an opportunity to refine one or more Data Management practices. In many cases, however, those anomalies go unreported, and the BI team compensates for the Data Quality issues through report design, which is incredibly inefficient.”
Data analysts and ad hoc report authors can grow frustrated with their BI solution, particularly if they are “familiar with what the data means and indicates” but “do not have the technical knowledge” or authority to improve the data-garbage-to-data-asset ratio.
Furthermore, these business people “do not always have a way to report back to data stewards, those accountable and responsible for Data Management practices, about problematic data quality,” Brody said. Analysts need some sort of BI feedback loop to impact changes in Data Management processes so that they are not stuck correcting the same reporting issues over and over again.
Looping BI/Data Management Feedback
The BI/Data Management feedback cycle can have myriad issues depending on the processes at a given organization, but data analysts need to produce reports without having to compensate for a growing backlog of Data Management issues. Brody said, “red tape or bureaucracy can make it completely pointless for the analysts to point out data quality problems stemming from Data Management.”
To call attention to Data Management issues, George Firican, Data Governance and Business Intelligence Director at the University of British Columbia, says data analysts sometimes take a renegade approach. He notes that purposefully not cleaning the data at the reporting level, leaving it in raw form, tends to get stakeholders’ attention, creating an opportunity to communicate with the stakeholders about the amount of time and money spent cleaning up the same problems every time a report is built using specific datasets.
At organizations without self-service BI, “individual contributors press BI analyst teams for the reports they need, creating a bottleneck.” The people who need the information right away cannot access it until the people with programming skills send back a meaningful, well-formatted report. Data Management issues only compound this problem:
“Making data-driven decisions should not rest on the few so that they feel overtaxed. Virtually everyone needs access to some kind of data to do their jobs effectively. Should the information processing responsibility rest only on a few shoulders or should there be some degree of independence and autonomy among that user group? Those who are non-technical and struggling need to have a way to express their pain points to the right people, so something can be done.”
Effective Data Management/BI feedback loops can make it easier for both data analysts and non-technical ad-hoc report authors to access quality data.
A Simpler BI Model for Better and Faster Business Insights
Analysts need a new model to get better business insights quicker without getting stuck in Data Management/BI feedback problems due to poor Data Management process implementation at the top of the pipeline. Exago, a company that develops embedded BI, has created a simpler way to ensure the data set is clean. Brody noted:
“Exago gives the end-user, as much as possible, immediate access to data through basic report designers. They are intuitive for non-technologists and as powerful as possible for those with more advanced skills and requirements. Organizations can therefore eliminate bottlenecks how they wish.”
A BI model that takes away an analyst’s pain in generating reports will encourage more use and lead to higher data monetization. In addition to a good application, Brody recommended:
- Thorough data preparation, devoting 80 percent of resources to planning and 20 percent to implementation
- Garnering a clear picture of how a company’s BI will be used so that it can be optimally administered
- Creating freer-flowing feedback channels back to data stewards
Data preparation is a key function within the entire Data Management pipeline. An organization can’t have an effective BI program without good data. The benefits of BI are exponential in proportion to the quality of that data. Once the data is in good order, understanding the specific organizational BI requirements and finding the right solution that satisfies those best is imperative. It’s all about creating an effective intersection between traditional Data Management processes and downstream BI requirements, but that intersection needs to flow both ways, “cooperative feedback along the entire pipeline affords success to the entire data stream,” Brody said in closing.
Image used under license from Shutterstock.com