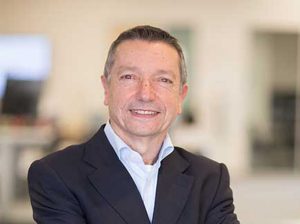
As we near the end of 2023, it is imperative for Data Management leaders to look in their rear-view mirrors to assess and, if needed, refine their Data Management strategies. One thing is clear; if data-centric organizations want to succeed in 2024, they will need to prepare for an environment in which data is increasingly distributed.
With this in mind, we see five important Data Management trends emerging in 2024: Data anti-gravity will prevail; data products will rise in importance; organizations will learn how to adopt generative AI (GenAI) and leverage it successfully; organizations will manage cloud costs more effectively; and data security and governance will be simplified.
Let’s take a closer look at each of these trends in turn:
Data Anti-Gravity Will Prevail
The notion of data gravity, which is an analogy of the nature of data and its ability to attract additional applications and services, no longer exists. Every organization with a modern Data Strategy needs a data warehouse alongside a data lake, if not multiple ones, to fulfill their business needs. In the last two decades, data warehouses and data lakes became popular to solve enterprise data silo problems, yet what they created were even bigger problems. This is because data warehouses and data lakes are comprised of both on-premises and cloud systems, and they are often geographically dispersed. Also, even though every cloud service provider tries to solve many data and analytics problems independently, most organizations run their data and analytics in a multi-cloud environment, cherry-picking products and services from two or more cloud service providers.
This is why data anti-gravity, where data and applications remain distributed across regional and cloud boundaries, will be the new norm in 2024 and beyond. Other factors contributing to data anti-gravity will be the rising costs of data replication, data sovereignty, local Data Governance laws and regulations, and the requirement for accelerated speed-to-insight. As the data anti-gravity trend continues, Data Management leaders should invest in technologies that are built on the premise of distributed Data Management.
Data Products Will Rise in Importance
2024 will be a pivotal year for the ascent of data mesh, which embraces the inherently distributed nature of data. In contrast with traditional, centralized paradigms in which data is stored and managed by a central data team that delivers data projects to business users, data mesh is organized around multiple data domains, each of which is managed by the primary business consumers of that data. In a data mesh, the role of IT shifts to providing the foundation for data domains to do their work, i.e., the creation and distribution of data products throughout the enterprise.
The turning point will be the realization that data products should be treated with the same level of importance as any other product offering. Take, for instance, a Tylenol capsule: Its value is not just in the capsule itself but in the comprehensive package that earns consumer trust—from the description and intended use to the ingredient list and safety measures. Similarly, data catalogs act as the crucial “packaging” that turns raw data into reliable, consumable assets.
In this data-centric era, it is not enough to merely package data attractively; organizations need to enhance the entire end-user experience. Echoing the best practices of e-commerce giants, contemporary data platforms must offer features like personalized recommendations and popular product highlights, while also building confidence through user endorsements and data lineage visibility. Moreover, these platforms should facilitate real-time queries directly from the data catalog and maintain an interactive feedback loop for user inquiries, data requests, and modifications. Just as timely delivery is essential in e-commerce, quick and dependable access to data is becoming indispensable for organizations.
Organizations Will Struggle to Both Adopt GenAI and Leverage It Successfully
Organizations are encountering multiple challenges as they attempt to implement GenAI and large language models (LLMs), including issues with data quality, governance, ethical compliance, and cost management. Each obstacle has direct or indirect ties to an organization’s overarching data management strategy, affecting the organization’s ability to ensure the integrity of the data fed into AI models, abide by complex regulatory guidelines, or facilitate the model’s integration into existing systems.
Organizations Will Need to Manage Cloud Costs More Effectively
As businesses continue to shift data operations to the cloud, they face a significant hurdle: the relentless, unsustainable escalation of cloud data expenses. For the year ahead, the mandate is not just to rein in these rising costs but to do so while maintaining high-quality service and competitive performance. Surging cloud hosting and Data Management costs are preventing companies from effectively forecasting and budgeting, and the previously reliable costs of on-premises data storage have become overshadowed by the volatile pricing structures of the cloud.
Addressing this financial strain requires businesses to thoroughly analyze cloud expenses and seek efficiencies without sacrificing performance. This involves a detailed examination of data usage patterns, pinpointing areas of inefficiency, and a consideration for more cost-effective storage options. To manage cloud data costs effectively, firms need to focus on the compute consumed by queries and the associated data egress volumes, tabulating the usage of datasets, and optimizing storage solutions. These efforts are enhanced by adopting financial operations (FinOps) principles, which blend financial accountability with the cloud’s flexible spending model.
By regularly monitoring expenditures, forecasting costs, and implementing financial best practices in cloud management, organizations can balance cost savings and operational efficacy, ensuring that their data strategies are economically and functionally robust. In 2024, we will see a significant rise in the use of FinOps dashboards to better manage cloud data charges.
Data Security and Governance Will Need To Be Simplified
Poorly integrated data impacts the agility of an organization on many levels, but this impact is perhaps felt most strongly in data security and governance. Because it takes time to update the myriad of siloed systems individually, it is impossible to secure or govern all enterprise systems simultaneously.
To meet this challenge, organizations are leveraging global policies for data security and governance. Global data security policies can be based not only on user roles, but also on location, so that a person on vacation might not be able to access the data from the main office. Global data governance policies too can automatically standardize the spelling of certain words, across the different systems within a company.
However, in order to synchronize the application of global policies in real time, such data security and governance implementations require the foundation of a logical approach to data management, and such an approach is covered in the next section.
The Future Is Logical
To overcome the challenges inherent in each of these five trends, organizations will need to be able to leverage Data Management strategies that are designed from the ground up to support distributed data. Traditional Data Management approaches rely on the physical replication of data from multiple systems into a central repository, like a data warehouse or data lake, but such approaches, by definition and also in practice, do not support inherently distributed data. In contrast, logical Data Management approaches enable real-time connections to disparate data without replication, to support inherently distributed data.
As a result, logical Data Management will be here to stay in 2024 and beyond, as it enables every organization to manage distributed data in the most efficient and cost-effective way possible.