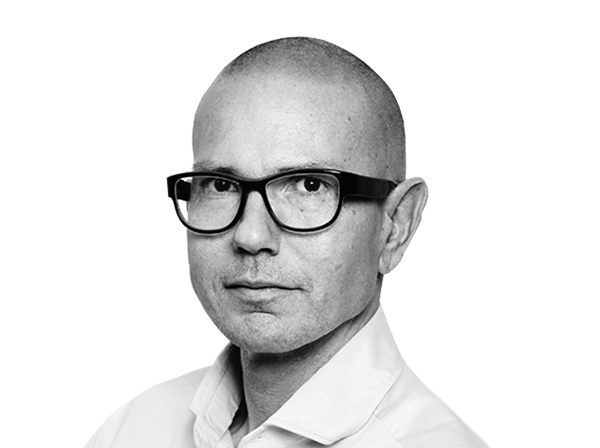
In my last DATAVERSITY article, “The Machine Economy Is Here – The Digital Transformation Era Is Over,” I discussed the end of digital transformation, the arrival of the machine economy, and the emergence of data empowerment.
In this article, I follow up by laying out the problems with traditional Data Management and why data empowerment is now flourishing across global circles.
To start, let us understand that there are significant obstacles that can grind the process to a halt for building a modern data estate:
- Organizations are experiencing an explosion in both the amount and the types of data that need to be collected, stored, and processed from a growing number of sources.
- Line-of-business teams outnumber data teams, which leads to a never-ending backlog of analytics requests. A quarter of business experts admit they have given up on getting an answer they needed because the data preparation and analysis took too long.
- Many companies are having a difficult time finding qualified people who possess the required and appropriate data and analytics skills.
- Data and analytics professionals are under constant pressure to spend what little free time they have on learning the latest technologies, tools, and methodologies.
- The data team is often forced to spend significant amounts of time on manual, repetitive data preparation tasks, which can lead to burnout and high turnover – 79% of data professionals have considered leaving the industry entirely.
- Almost all of the data professionals surveyed report concerns around controlling access to sensitive data, accidental data deletion, errors when analyzing data that lead to poor decision-making, security breaches, and regulatory compliance issues.
- Communication barriers between business experts and the data team often create bottlenecks and slowdowns – 34% of business experts admit they are not confident in articulating data questions or needs to the data team.
These issues can cause bottlenecks and frustration, inhibit growth, and do considerable damage to your organization. To overcome these challenges, data teams typically take one of two approaches to building their data infrastructure:
Traditional Approach #1: The Stack
The process of ingesting, preparing, and delivering data for analysis has traditionally relied on a highly complex stack of tools, a growing list of data sources and systems, and months spent hand-coding each piece together to form data “pipelines.”
There are several problems with this approach:
- Manual coding and pipeline creation: New pipelines must be manually built for each data source, data store, and use case (for example, analytics reports) in the organization, which often results in the creation of a massive network of fragile pipelines. A large number of data professionals have stated that they spend a significant amount of their time on these types of manual, repetitive tasks.
- Stacks on stacks of tools: There is often a separate stack of tools for managing each stage of the pipeline, which multiplies the number of tools in use and creates additional silos of knowledge and specialization.
- Vulnerable, rigid infrastructure: Building and maintaining these complex data infrastructures and pipelines is costly and time-consuming, introduces ongoing security vulnerabilities and governance issues, and makes it extremely difficult to adopt new technologies in the future.
- Fragile pipelines: Even worse, these data pipelines are hard to build, but easy to break. More complexity means a higher chance that unexpected bugs and errors will disrupt processes, corrupt data, and fracture the entire pipeline.
- Manual documentation and debugging: Each time an error occurs, data engineers must take the time to go through the data lineage and track down the error. This is extremely difficult if the metadata documentation is incomplete or missing (which it often is).
Traditional Approach #2: The Platform
The Data Management market is now full of “platforms” that promise to reduce complexity by combining all your data storage, ingestion, preparation, and analysis tools into a single, unified, end-to-end solution.
While this might sound ideal, these claims start to fall apart upon closer inspection:
- Stacks in disguise: Most “platforms” are just stacks of tools that have been bundled together and sold under an unnecessarily complicated pricing model. This requires that you have professionals who are qualified to use each of the tools in the stack. Plus, you’ll have to account for training and having data siloed throughout your organization.
- Since it’s a “platform,” you’d expect a simple, clean user interface, right? Instead, you get chaos. Yes, all the tools have been bundled together and sold by the same vendor, but they’re often collected through acquisitions, and it ends up just being a big, ugly mess of incompatible code that has been haphazardly stitched together into a “platform.”
- Low-code: Many of these platforms brag about being “low-code,” but when you dig into the details, there’s usually only one or two features that actually have this functionality.
- Welcome to Data Management prison: Worst of all, you end up being locked into a proprietary ecosystem that won’t allow you to truly own, store, or control your own data. All tools and processes are pre-defined by the platform developer, and then hidden in a “black box” that you can’t access or modify. Many of these platforms even force you to migrate all your data to the cloud, and do not offer support for on-premise or hybrid approaches.
- Trying to escape might cost you everything: Not only do these platforms significantly limit your Data Management options, but if you decide to migrate to a different data platform later, you must rebuild nearly everything from the ground up.
These solutions are not truly “platforms,” and they don’t really “unify” anything. They’re just stacks with better branding and a lot more restrictions.
Data Management Is Dead – Data Empowerment Has Emerged
I don’t believe you should be forced to spend months hand-coding fragile pipelines between each component of your data estate using a complex stack of tools. Nor do I believe in poorly integrated “platforms” that impose strict controls and lock you into a proprietary ecosystem.
It’s clear that these old approaches to Data Management simply cannot meet the needs of modern data teams. The rapid pace of the Machine Economy does not allow for bottlenecks, slowdowns, and limitations these approaches bring.
The entire status quo of the “Data Management” industry is archaic, burdensome, and oppressive, and should be abolished – replaced by solutions that are low-code (to automatically generate all code and documentation); agile (with drag and drop user interface to expedite user needs for data, analytics, AI, and machine learning); and integrated into a single, unified solution that overlays your data infrastructure. With that, you are well on your way to achieving data empowerment.