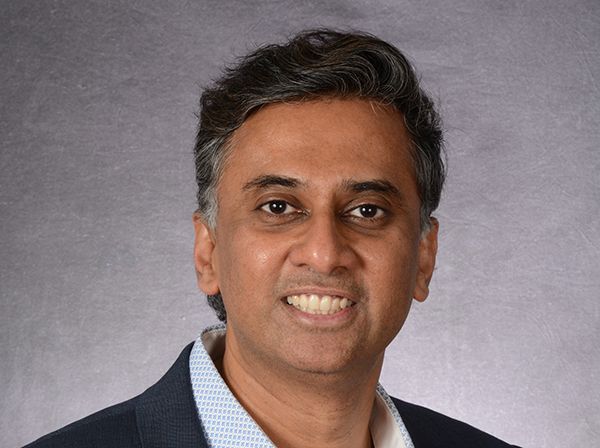
The once-disconnected worlds of data management and analytics are increasingly colliding in front of our eyes, even if not everybody sees it. We are in the midst of a quiet revolution and entering a new era in which all the key ingredients for successful decision-making are coming together. That combination will see the emergence of a single, harmonious decisioning engine. With tools working seamlessly together and with preset (but editable) workflows and processes, the new ability to plan based on empirical, auditable, timely information will in turn change the way that organizations plan and set strategy.
Tricky Times
Back in 2020, Deloitte correctly proclaimed that making data-driven decisions in the “new normal” post-pandemic period would be more difficult than ever. That’s because the COVID-19 pandemic created a dividing line between “before” and “after.” Immediately post-pandemic, we couldn’t rely on historical precedents, because so many of our habits had changed. We no longer worked, shopped, socialized, or traveled in the same way, so the rear-view mirror became less useful than it was previously.
Today, we are getting used to the post-lockdown world, even if its long-tail effects persist in the form of flexible working, a lagging economy, and pressure on healthcare services. But we also live in a time of renewed uncertainty, and we must factor in so many variables and imponderables. Think, for example, of geopolitics and trade embargoes, the crisis in Ukraine affecting supply-and-demand dynamics, the rise of political populism, growing globalization impacts, the explosion of richly funded disruptive startups, hyper-inflation, and other factors.
These all mean we need to get to decisions quickly and, just as importantly, be able to adapt on the fly to changes in our environments and course-correct. For this new reality, the old world of dashboards, reports, and scorecards with little automation and lots of manual processes won’t cut it. We need to be faster, more accurate, able to understand more vectors, and more adaptive.
Bringing It All Back Home
For too long, the core elements of a decisioning stack have been scattered like confetti and disconnected like an archipelago. Making smart decisions will typically involve digital tools for data science, AI, machine learning, metadata, master data management, data quality, business intelligence, querying, and visualization. But these have been treated as silos, or at best, small groups of tools have been integrated.
Today, however, the arrival of comprehensive and truly integrated cloud suites with outstanding usability and cross-tool interactivity means that we can do so much more. We can understand operations at a forensic level, make decisions in close to real time, and anticipate opportunities and risks. Machines and humans can work together so users don’t even always have to ask questions for assistance. Our bots can warn us, flagging risks and opportunities, and then recommend prescriptive actions to optimize or remediate.
Yes, we still need smart people, but they can use these new decision-support fabrics without having to shell out for different tools that slow performance or suffer inter-application disconnects that create data islands. The new decisioning engines are also great platforms on which to build AI and other hot technologies because the foundations enable us to manage data that is reliable, clean, and complete.
What will the new world of comprehensive decisioning engines help us address? The short answer is everything: From financial analysts identifying investor churn risk, to doctors pinpointing patient care pathways with the treatments most likely to succeed, to crime agencies discovering where to station police officers.
Understanding Domino Effects
As with any major inflection point, this new world will necessitate other changes in the way decisions are made and sanctioned, as well as how we manage operations. We will need to build cultures in which it is widely accepted that evidential data should back up every significant decision.
The adage, “If you can’t count it, it doesn’t count,” will need to be underlined twice, then highlighted with a fluorescent marker. But, at the same time, Einstein’s epigram also holds true: “Many of the things you can count, don’t count. Many of the things you can’t count, really count.” That is, we will need to use data sensibly, in such a way that “analysis paralysis” doesn’t kick in because we start to believe that even the simplest call must have its work shown like a child’s math homework. And we should always have human gatekeepers who understand the possibilities of “garbage in, garbage out” and exercise common sense and domain knowledge for large decisions.
The possibilities of dealing with dirty data or misreading data are always present. After all, no matter what the data tells us, if it barks, wags a tail, and has four legs, it will probably be a dog. But, if we are serious about using data in a sophisticated manner, and if we want it to inform decision-making, we will have to jettison some arcane activities. The old, often macho, tenets of managing by hunch and gut must go. CEOs may still become superstars, but not by intuitive reading of markets or trends or making ridiculous bets. Instead, they will do so by fostering environments that enable them and their people to make smarter, more logical decisions.